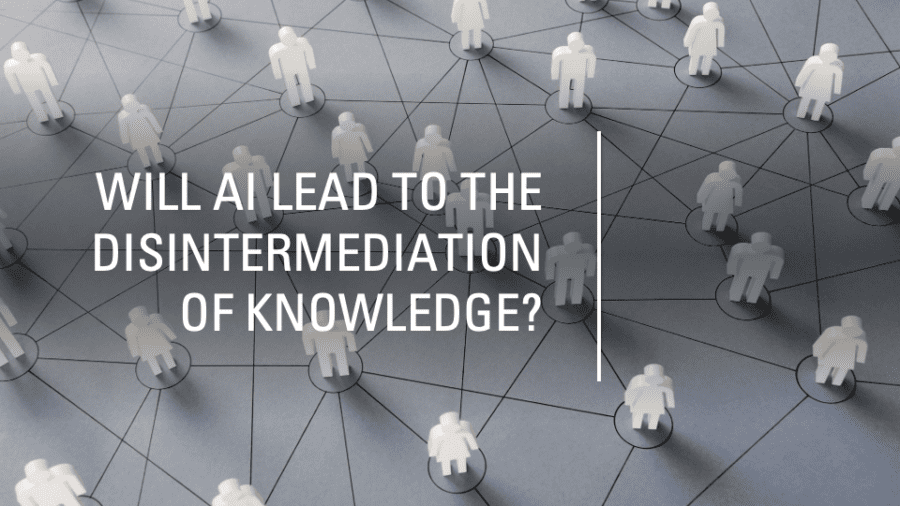
Key Blog Points:
- AI is redistributing knowledge, not replacing it. AI democratizes access to expertise, empowering employees at all levels rather than concentrating intelligence at the top.
- Data economics turns AI into a knowledge multiplier. The more AI-driven insights are shared and reused across an organization, the more significant their impact.
- General Motors’ pivot illustrates the power of AI augmentation over autonomy. AI succeeds when it enhances human decision-making, not when it tries to replace it.
- Cognitive outsourcing is a risk. Overreliance on AI can erode critical thinking, reinforce bias, and weaken institutional knowledge. AI should assist, not dictate.
- Winning organizations will integrate AI to enhance decision-making. The future belongs to companies that leverage AI as a cognitive amplifier while maintaining strong human oversight and adaptability.
For decades, organizations have operated under the central assumption that knowledge flows downward. Senior leaders, industry veterans, and domain experts have traditionally been the primary gatekeepers of critical information. Their insights, honed over years of experience, have been the cornerstone of strategic decision-making.
Enter artificial intelligence (AI). Many folks are concerned that AI will lead to cognitive outsourcing, where organizations rely on algorithms instead of human judgment and will replace humans with algorithms to reduce costs. In this vision, human knowledge is discounted, and AI-driven decisions dictate strategy, often without the necessary human context.
But I believe the opposite is true. AI’s true impact will be on the disintermediation of knowledge. This shift will democratize expertise, making actionable insights accessible at all organizational levels. Instead of concentrating intelligence at the top, AI distributes it to the edges of the organization at the points of customer engagement and operational execution, where customer, operational, and societal value creation happens.
The Legacy of Centralized Knowledge
For much of modern business history, decision-making has been hierarchical, where knowledge flowed from the top down. Employees executed strategies dictated by leadership, leaving little room for real-time adaptation. This model thrived in stable environments with predictable change. However, as businesses now face rapid disruptions and increasing complexity, this hierarchical approach is too slow and reliant on a few decision-makers (Figure 1).
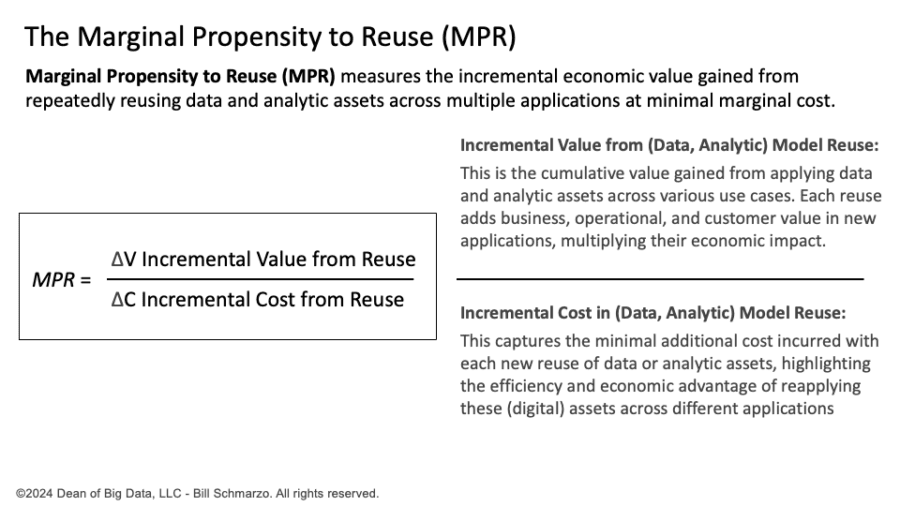
Figure 1: Traditional Organizational Knowledge Hierarchy
Today’s organizations need agility and responsiveness to navigate rapid technological advancements, shifting consumer behaviors, and global economic fluctuations. Many are turning to decentralized decision-making models that empower employees at all levels to contribute, fostering innovation and enhancing engagement.
Modern technology also supports this shift, enabling real-time collaboration through tools like data analytics and communication platforms. By embracing a more democratic approach to knowledge sharing, organizations can adapt more swiftly to change and cultivate a culture of continuous improvement. In this evolving landscape, moving away from hierarchical decision-making is vital for thriving in a fast-paced business world.
Marginal Propensity to Reuse: AI as a Knowledge Multiplier
This is where data economics becomes crucial in today’s landscape. Unlike traditional economics, which focuses on efficiently allocating scarce resources, data represents a paradigm shift since it is inherently abundant and reusable. Its value escalates exponentially when employed across various applications. Organizations that do not adapt to this change risk becoming ensnared in outdated frameworks, where knowledge is hoarded at the top instead of dynamically distributed throughout all levels of the organization.
This is where the Marginal Propensity to Reuse (MPR) concept comes into play. MPR measures the incremental economic value gained from repeatedly reusing data and analytic assets across multiple applications at minimal marginal cost (Figure 2).
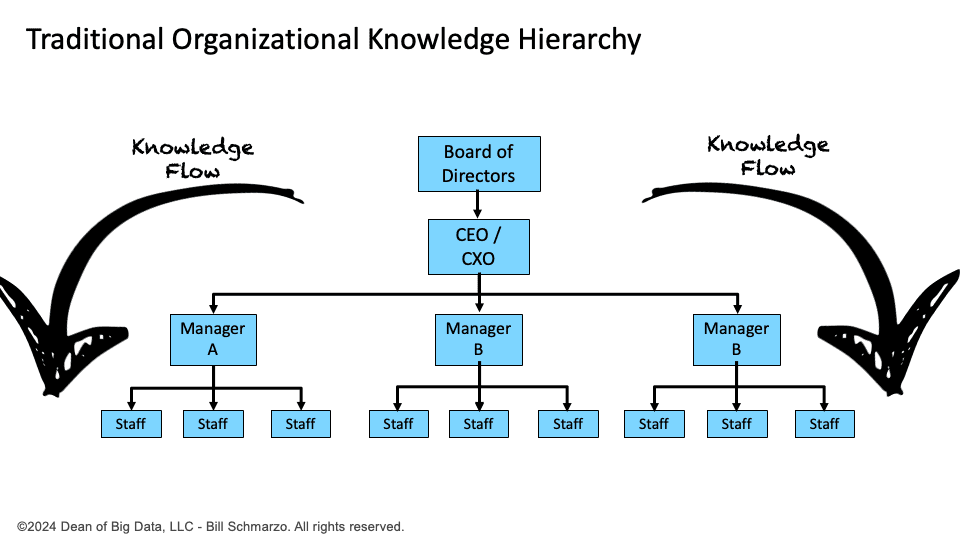
Figure 2: Marginal Propensity to Reuse (MPR)
MPR stands in stark contrast to traditional assets, which typically lose value as they are utilized. Data, on the other hand, gains value the more it is applied across different business functions. The integration of artificial intelligence into this equation enhances these dynamics, enabling the analysis of vast data sets to reveal insights that are not only more accessible but also contextual and reusable across the entirety of an organization.
This shift in perspective underlines the need for organizations to cultivate a culture that embraces data sharing and collaboration, breaking down silos and encouraging innovation. By harnessing the true potential of data, organizations can drive sustained growth and adaptability in an increasingly complex environment.
General Motors’ AI Pivot: A Case Study in Human-Augmented Decision-Making
The recent decision by General Motors (GM) to abandon its commercial robotaxi initiative in favor of personal autonomous vehicles (PAVs) is a real-world example of this shift. GM has shifted away from commercial robotaxis after investing over $10 billion in Cruise, its autonomous vehicle (AV) division .
Instead, GM is focusing on incremental AI adoption, enhancing its existing Super Cruise driver assistance system with AI-driven features that improve safety and the driving experience. AI isn’t replacing human decision-making but enhancing it in practical, incremental, and broadly applicable ways. This approach better aligns with how AI creates value today: not by replacing human control but augmenting it.
GM’s pivot highlights a crucial lesson: AI adoption succeeds when it enhances, rather than replaces, human expertise.
- AI-driven robotaxis represented an attempt to outsource human decision-making entirely, a leap that is too far for both technology and society.
- AI-driven personal autonomous vehicles represent a gradual, pragmatic integration—where AI assists but doesn’t override human control.
This shift mirrors how AI is successfully integrated across industries—not as a decision-making substitute but as an augmentation tool that empowers employees to make better, faster, and more informed decisions.
The Pitfalls of Cognitive Outsourcing
There is a dangerous counter-narrative: AI will lead to cognitive outsourcing, where companies increasingly rely on algorithms to make decisions without human oversight. This approach often pursued efficiency and cost reduction, poses significant risks that organizations must carefully navigate.
1. Loss of Critical Thinking and Human Judgment
- Over-reliance on AI can lead employees to blindly accept machine-generated recommendations without critically evaluating their validity or considering alternative approaches.
- As AI automates decisions, workers may lose opportunities to engage in creative problem-solving, curiosity-driven learning, and experiential knowledge-building—all crucial for innovation.
2. Increased AI Bias and Black-Box Decision-Making
- AI inherits and amplifies biases present in its training data. If left unchecked, this can lead to discriminatory outcomes at scale.
- Black-box AI models, where the rationale behind decisions is opaque, make it difficult for organizations to explain or justify AI-driven decisions. This can lead to potential compliance risks, ethical concerns, and reputational damage.
3. Erosion of Institutional Knowledge and Experience
- If companies lean too heavily on AI for strategic guidance, organizational memory and intuition may erode, leaving them vulnerable to unexpected market shifts that AI cannot predict.
- Institutional knowledge—the hard-won expertise employees develop over years of experience—is often unstructured, contextual, and complex to encode into AI models.
How Organizations Can Avoid Cognitive Outsourcing Pitfalls
Rather than blindly outsourcing decision-making to AI, organizations should embed AI into workflows to enhance human intuition and build institutional expertise. The key is AI augmentation, not replacement.
1. Empower Employees with AI-enhanced decision-making
- AI should inform and augment human judgment rather than dictate decisions.
- Organizations should implement AI-assisted analytics or assistants that allow employees to understand why a model made a specific recommendation and will enable them to override AI when necessary.
2. Ensure Transparent and Explainable AI
- Organizations must demand transparency from AI models and implement explainable AI (XAI) techniques to ensure that AI-driven decisions can be interpreted, audited, and improved over time.
- AI must be subjected to human validation checkpoints, particularly in high-risk domains such as healthcare, hiring, and financial lending.
3. Foster a Culture of Continuous Learning and AI & Data Literacy
- AI should be seen as a tool for knowledge amplification, not replacement. Consequently, all employees should be trained in AI and data literacy, ensuring they understand how AI works, its limitations, and how to engage with its outputs critically.
- A feedback loop should be established, allowing employees to flag AI errors, refine model accuracy, and return their expertise to the system.
4. Balance AI Automation with Human Oversight
- AI models should not be granted unchecked authority—instead, they should operate as collaborative partners with human experts.
- Scenario testing and impact analysis should be conducted regularly to assess how AI-driven decisions affect real-world outcomes.
5. Prioritize AI Strategies That Enhance, Not Replace, Human Ingenuity
- Organizations that successfully integrate AI into decision-making processes will democratize knowledge, improve operational efficiency, and accelerate innovation—but only when human expertise remains central to the process.
- The companies that win in the AI era will use AI as a cognitive amplifier, not a cognitive replacement.
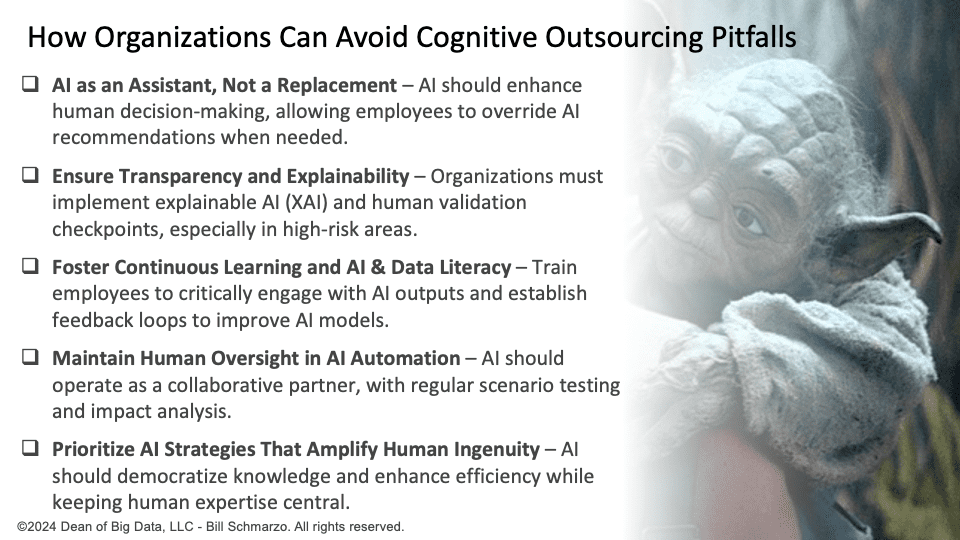
The Future of AI-Enabled Decision-Making
AI will empower the disintermediation of knowledge. Leading organizations will exploit, not fight, that reality by empowering everyone in the organization to understand how best to leverage AI and data to create new sources of organizational value.
Rather than fearing cognitive outsourcing, businesses should embrace AI as a catalyst for democratized decision-making. AI is not an existential threat to human expertise—it is the next evolution of how organizations create, distribute, and act on knowledge.
The companies that understand this shift and build their AI strategies around distributing rather than concentrating intelligence will win. The AI era is not about who controls knowledge but who can access, apply, and turn knowledge into action.