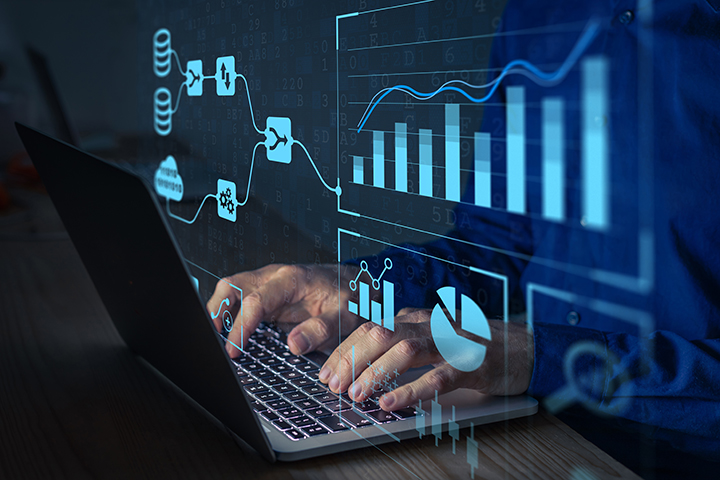
Why do I think Data Management is the most important business discipline for the 21st century (and why my “Big Data MBA” book and course are more relevant than ever)? Here is my self-serving logic:
- Artificial Intelligence (AI) is the most powerful business discipline of our generation (with AI’s ability to continuously learn and adapt to create new sources of customer, product, service, and operational value).
- The ability for AI to drive effective and responsible decisions is uber highly dependent upon high-quality, accurate, complete, unbiased data sets.
- Data management focus on manufacturing high-quality, accurate, complete, unbiased data sets is instrumental to the economic growth in the 21st century
- Thusly, Data Management is the most important Business Discipline of the 21st century
IF A (AI most powerful economic force) = B (AI requires high-quality data), AND B (AI requires high-quality data) = C (data management manufactures high-quality data), THEN A (AI most powerful economic force) = C (data management) yields D (Data Management most important business discipline), right?
And let’s be clear: what I am saying is that Data Management is more than just a technology capability. Data Management is the most important business discipline in the age where data is the most valuable resource in the world and is the catalyst for driving the economic growth of the 21st century. Here’s more on my rationale.
A. AI Most Powerful Business Discipline and Economic Force
Research touting the business and society benefits of AI is everywhere (reminds me of Chickenman…he’s everywhere, he’s everywhere!!). PwC estimates in “Sizing the prize: PwC’s Global Artificial Intelligence Study: Exploiting the AI Revolution” that AI could contribute up to $15.7 trillion to the global economy in 2030, more than the current output of China and India combined, with $6.6 trillion of that $15.7 trillion coming from increased productivity and $9.1 trillion from consumption-side effects (Figure 1).
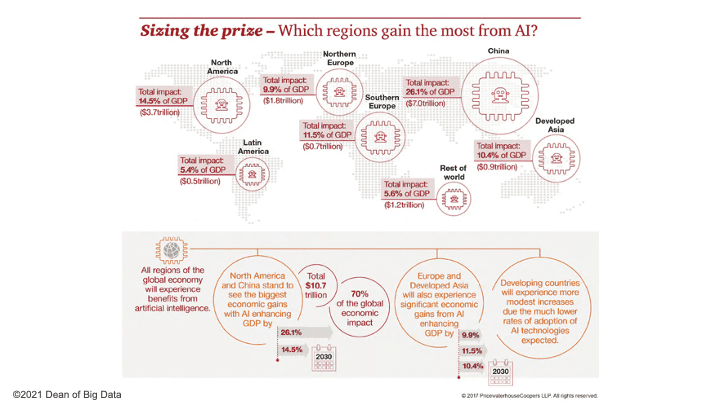
Figure 1: PwC “Sizing the AI Prize”
McKinsey has their version of this same point about the economic and societal value of AI, which they tout in the research titled “The state of AI in 2021.” McKinsey estimates that AI will impact a wide and diverse variety of use cases including those in Figure 2.
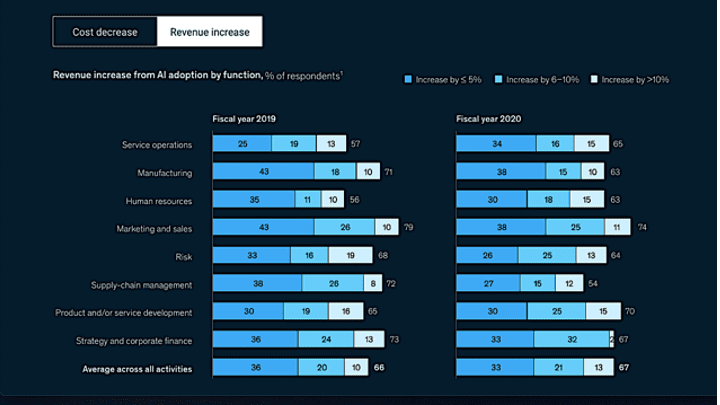
Figure 2: McKinsey “The State of AI in 2021”
Conclusion: Artificial Intelligence (AI) is the most powerful business discipline and economic force of our generation.
B. AI Requires High-quality Data
There is a growing realization by some of the AI industry’s leading thinkers (such as Andrew Ng) that the full potential of AI will never be reached without significant focus, investment, and improvement in the data that feeds the AI machine. From the article “Andrew Ng Launches A Campaign For Data-Centric AI”, we get the following proclamation:
“Paradoxically, data is the most under-valued and de-glamorised aspect of AI” say Google researchers in a recent paper, reporting on their survey of 53 AI practitioners. They found that “data cascades—compounding events causing negative, downstream effects from data issues—triggered by conventional AI/ML practices that undervalue data quality… are pervasive (92% prevalence), invisible, delayed, but often avoidable.”
The Google research paper “Data Cascades in High-Stakes AI” highlights the critical challenge of “data cascades” when dealing with problems that extend across data sets and subject matter experts.
Data Cascades are compounding events causing negative, compounding, downstream effects from data issues triggered by conventional AI/ML practices that undervalue data quality.
Data cascades are pervasive, invisible, and compounded by need for tight collaboration between different stakeholders in discovering those variables and metrics that might be better predictors of performance (ML Features) where each stakeholder has a different view on the problem and even different terminology (Figure 3).
Figure 3: Ramifications of “Data Cascades”
Cascades are triggered in the upstream processes (e.g., data collection, data cleansing, data preparation, data enrichment, data governance, master data management) and have impacts on the downstream execution (e.g., model deployment, analytic score generation, decision making, and value creation effectiveness).
Conclusion: The ability for AI to drive effective and responsible decisions is highly dependent upon high-quality, accurate, and complete data sets. The ramifications from poor-quality or incomplete data on the AI model’s effectiveness and fairness can have disastrous, unintended consequences in areas ranging from employment, loans, housing, education, criminal justice, and healthcare.
C. Data Management Is the Most Important Business Discipline
A Deloitte research article highlights three areas where “Intelligent Data Management” – which is the application of AI / ML to enhance, automate, and optimize key data management tasks – could have material impact on making Data Management more relevant and actionable to the business.
- Data Quality: Identifying and resolving data quality issues. Suggesting data quality rules based on existing datasets and updating existing data quality rules, and then automatically running them. Automating ongoing data quality checks and advanced data profiling. Recognizing patterns and anomalies. Suggesting actions for data cleansing, based on predicted values and manual data cleansing.
- Metadata Management: Labelling, classifying, cataloging, and searching data. Deriving the metadata model and metadata rules from datasets. Automatically collecting, organizing, cataloging, and merging technical and business metadata, both for structured data and unstructured data. Generating and analyzing end-to-end data lineage to identify system dependencies, data flows and anomalies.
- Master Data Management: Identifying and evaluating potential master data. Automatically generating a master data model, mapping data / business entities, and configuring an Master Data Management hub. Suggesting actions for matching and merging to establish a single source of truth, based on usage patterns, trust scores and data steward input.
Summary: Data Management is the Most Important Business Discipline
If data is “the world’s most valuable resource”, then we must reposition data management as a business discipline. We must help organizations understand how best to reframe the data management conversation to help organizations to derive and drive more customer, product, service, and operational value from the application of data to their business.
As data becomes the ubiquitous source of economic value creation, organizations need an end-to-end data-driven value creation process that speaks to and aligns the business executives and the data and analytics teams around the business mandate to unleash the business (or economic) value of the organization’s vast data reserves. Organizations need a value creation flywheel (Figure 4)!
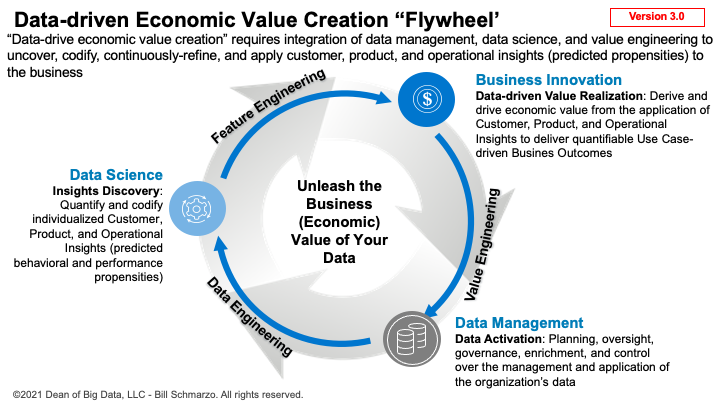
Figure 4: Data-driven Economic Value Creation Flywheel
The goal of Data-driven Economic Value Creation Flywheel is to create an end-to-end data and analytics value creation lifecycle that integrates data management (data engineering) with data science (feature engineering) and business management and data-driven innovation (value engineering), and then feeds back (backpropagates?) the analytics and business outcomes effectiveness to create a continuously-learning, adjusting, and refining (semi-autonomous) value creation process.
Finally, if we believe that data will be the catalyst for the economic growth in the 21st century, then we need to spend less time and investments trying to manage data and dramatically increase the time and investments to monetize or derive value from data. That will require organizations to reposition their data management capabilities as a business imperative that enables the sharing, re-using, and continuous refinement of the data and analytics economic assets to derive and drive new sources of customer, product, and operational value.
As is typical in most business initiatives, it all comes back to “show me the money.”