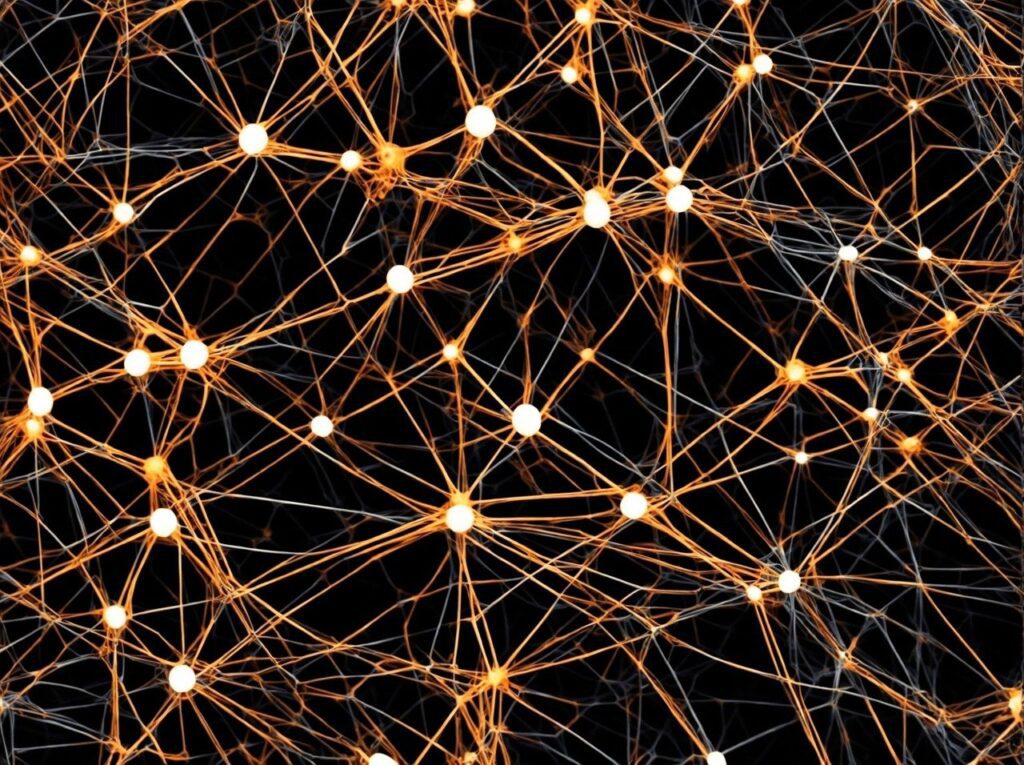
Image by Pete Linforth from Pixabay
Technological advances useful in solving some problems can often trigger side effects and other problems in the process. Consider the power of natural language processing, generative AI and related forms of AI in accelerating the knowledge graph development process, for example.
If you’re an equity analyst, experiencing that kind of power can trigger thoughts like, “Hey. I can process all the annual reports from this OEM (=original equipment manufacturer, such as a computer or car maker) and also from their partner and supply networks, mine those relationships and predict the impact of their second and third-order effects. Connecting that graph with a news graph could help refine, geolocate and time those predictions.’
In fact, senior solutions architect Xan Huang posted in April on the Amazon Web Services Machine Learning blog about exactly this sort of thing, and gave the example of how “delays at a parts supplier may disrupt production for downstream auto manufacturers in a portfolio though none are directly referenced.”
I’m not denying the usefulness of such a solution. But what bothers me is individuals generating their own semantic metadata, resolving the entities and assembling their own graphs with datasets that should already have this sort of metadata and self-describing auto-aggregation capability built in.
What generative AI and Excel have in common: Waste
Let’s step back and ponder the impact of this side effect of individuals burning up this time and energy globally. What a duplication of effort and a waste of energy! Shouldn’t the reports themselves already contain the necessary semantic metadata? Shouldn’t the industry-wide graphs be up and running and accessible as part of a public service?
Such an open public service should be creating and maintaining an industry graph for each industry, updating each graph continually, providing the public with its own visibility into supply and partner chains, on a timelier basis.
Turns out folks at the Object Management Group are working on a standard to make this sort of shared efficiency and effectiveness in financial reporting possible.
The work in progress is called the Standard Business Report Model (SBRM). The goal of SBRM is to logically contextualize business documents (such as public financial report data, which is non-perishable by definition) so that they’re interoperable. When they’re logically consistent and governed, the goal of SBRM is for spreadsheets to “talk to each other.”
SBRM in this way promises to reduce what’s commonly known as “spreadsheet hell,” in which all sorts of rework and duplication has become the norm, simply because an effective means of spreadsheet reusability hasn’t existed. SBRM has momentum because of the clear need for a shared historical record of company and industry performance. Spreadsheets that officially document company financials should obviously be reusable and interoperable.
Growing in popularity: Connected data via a unitary graph data model
A standards-based knowledge graph shares data across systems in a trusted way, via a data model that’s logically consistent from upper ontology, to domain ontology, to domain object. That model can be a functional part of the knowledge graph and is itself reusable because of its role in dynamically contextualizing data.
Proprietary graphs leverage the same basic principles as part of increasingly automated, operational systems. Dave Duggal, founder of interoperation and automation platform EnterpriseWeb, points out that EWEB’s architecture, for example, has all graphs up and down. But when processing, EWEB just sees all of these as a single, unitary graph.
In essence, EWEB has a hypergraph architecture that’s agent managed for enterprise-wide automation purposes. (See “Taming generative AI for enterprise-grade automation” at https://www.datasciencecentral.com/taming-generative-ai-for-enterprise-grade-automation for more information.) Many telecom carriers use EWEB for software-defined network creation and configuration changes.
The global graph technology market generally is forecast to grow at a compound annual rate of nearly 22 percent over a ten-year period, rising from $3.25 billion in 2022 to $23.5 billion by 2032, according to Polaris Market Research.
The knowledge graph market on its own is about a quarter of the size of this graph technology market. M&M estimated the 2023 global knowledge graph market at $0.9 billion, forecasting market growth to $2.4 billion by 2028, with a similar compound annual growth rate of 21.9 percent.
Networked data as a mirror of the natural world
Graph data systems can be designed to grow and scale organically, mirroring what the natural world does. At the heart of all business representations are human connections and how humans in organizations are interacting with each other and with the physical world.
There have been commercial and non-profit efforts to build single family trees such as Ancestry.com, FamilySearch and WikiTree for many years now, in reasonable attempts to reduce duplication of effort.
Several years ago, Blue Brain Nexus, a shared, dynamic knowledge graph resource based in Switzerland for the Blue Brain Project (focused on reverse engineering the brain), made global mixed data sharing possible between academics globally.
In addition, the project built a Github site for users not familiar with knowledge graph technology to build a small knowledge graph and query its JSON-LD that semantically links different contexts together:
These boundary-crossing collaboration efforts can reduce the amount of rework. Much depends on continued efforts to encourage broader data sharing, reduce siloing and focus more on data that has continuing historical value.