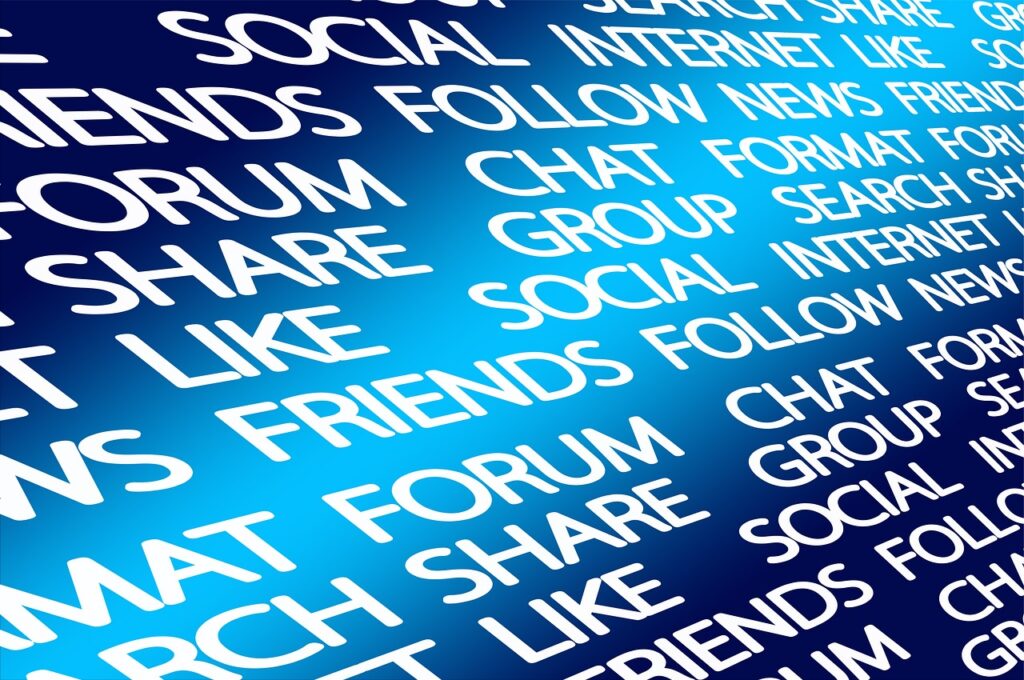
Technical Advisor and former LinkedIn knowledge graph lead Mike Dillinger recently spoke with Juan Sequeda and Tim Gasper of data.world. During a recent edition of the Catalog & Cocktails podcast, Dillinger stated that most data labels are meaningless text strings. “The vast majority are just junk that we pay for,” he noted.
Dillinger is a proponent of knowledge graphs for good reason: When well designed and implemented, they provide sufficient context essential to AI. Large language models (LLMs) need that explicit, articulated context, which is why a hybrid knowledge graph and statistical machine learning approach will be essential for artificial general intelligence (AGI).
When LLMs are trained on document collections, Dillinger pointed out, that method implies duplication and variation. Which version of a company’s product information is the version the LLM should use? That’s just one open question of many surrounding the inadequate data handling methods of today’s data science practitioners.
The need for relationship specificity
In their October 2023 paper “Mind the Labels: Describing Relations in Knowledge Graphs With Pretrained Models,” Zdenek Kasner, et al. of Charles University in Prague and Heriot-Watt University in Edinburgh observe that “Data-to-text generation models use relation labels (such as godparent, occupant, and musicBy) to describe relations between entities…. Unclear labels can lead to various lexical or semantic incoherencies in the output descriptions, such as swapping the relation direction (a) or using too literal expressions (b).”
(See their Figure 1 below for an example of the model interpretation contrasted with the actual reference meaning. The confusion arises because it’s not clear to the model who the godparent is.)
The authors of the paper took the time to assemble a dataset with nearly 4,100 triples and over 1,500 unique relationships in order to assess the effectiveness of verbalizing relationships as a starting point for comparing graph-to-text to the far less explicit, relationship impoverished data-to-text data labeling process under scrutiny.
The larger point I’ve tried to make over the years is that half or more of the essential data tends to be missing in most statistical machine learning processes. A best practice that seems entirely absent in today’s approaches would be to develop the quality, relationship-rich, explicitly logical and articulated data up front, rather than using an ad-hoc method downstream to try to correct what’s wrong with the data in that part of the pipeline.
Organic, lasting, growing data
In a June 30, 2023 post for Data Science Central, I described “data” as silica in terms of how most data scientists approach it. That concept of data assumes it can only be inert and inorganic. You have to add metal and heat to make electrically active silicon wafers out of it. Otherwise, it’s just sand, not a renewable resource.
Whereas data can and should be developed as an organic, dynamic, interactive representation of the living world. Any digital twin that’s interactive and reusable should have connected, meaningful data at the heart of it.
Such an organic approach would front load the data quality effort. It would demand more time and effort up front, but the result could be a renewable resource, implying far less effort downstream.
Building up such a renewable resource will require more than data scientists, architects and engineers. It will require others who are responsible for a wealth of domains who can help with accurate representations of the entities within those domains, how they’re interrelated, and how they interact. Representations are models, and modeling should be a team sport.
A risk-aware approach to data quality
Half measures like the underpowered approach to data labeling that’s most common aren’t helping businesses get their arms around AI. One of the initiatives we’re planning at the Dataworthy Collective for 2024 is a data quality framework. That framework will leverage the findings of previous semantic knowledge graph-related efforts that have demonstratively boosted data quality.
Some of the more candid and insightful folks are fed up with AI’s persistent inadequacies and are speaking out. In the process, they’re helping us put all of the major problems with AI out on the table. One of those is Wikipedia founder Jimmy Wales, who articulated one of the main business risks.
“Most businesses, not just charities like us, would say you have to be really, really careful if you’re going to put at the heart of your business a technology that’s controlled by someone else because if they go in a different direction, your whole business may be at risk,’ said Wales, quoted by Pascale Davies in a November 2023 Euronext article.
I couldn’t have phrased it better myself.