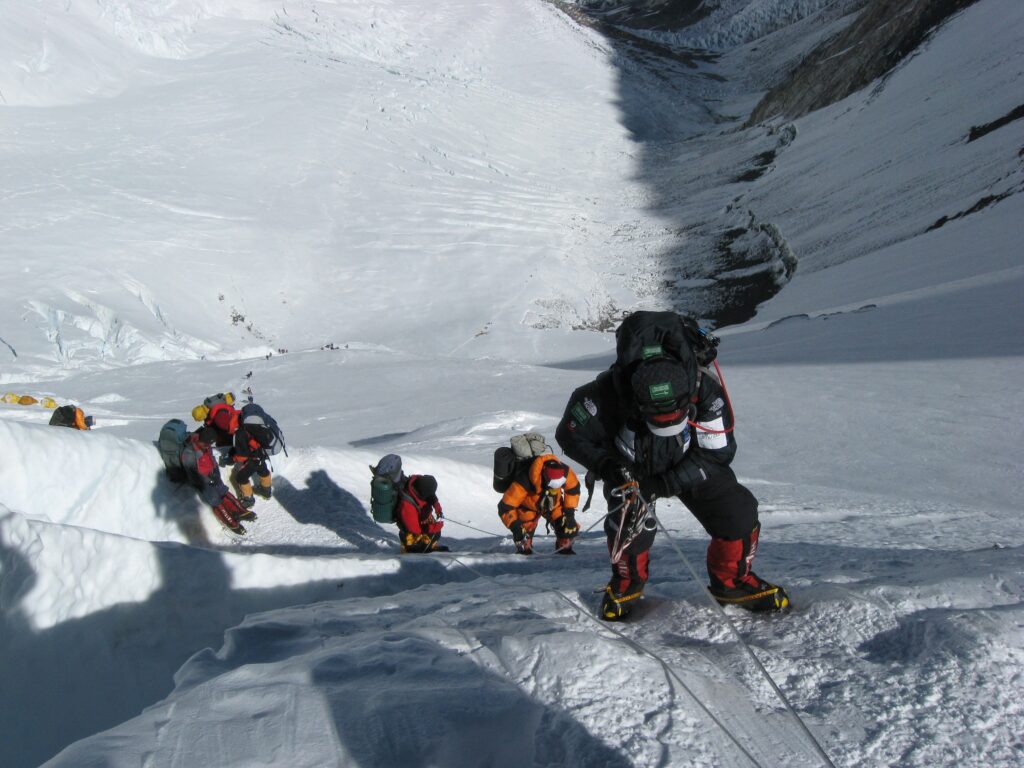
PickPik, royalty-free, undated
Companies for a decade now have been thinking they have to adopt AI, or they’ll get left behind. But most still don’t acknowledge the nature or the depth of the real challenge they face. Ultimately, it’s not an algorithm or machine learning challenge. Instead, it’s a people, process, data resource and app rationalization challenge.
The vast majority of enterprises are not using a divide-and-conquer approach to the challenge. Nor are they looking at systems and data and app maturity holistically, within the context of the kind of data transformed systems that actually enable deep and worthwhile AI adoption, the kind of adoption that leads to competitive advantage.
The AI hiring dilemma and its application-centric legacy
If you look at most of the job requirements, there’s a laundry list of dozens of tools companies are hoping data science, engineering, and related development candidates will be versed in. This situation has existed for many years now, and it’s getting worse.
Few people are going to know all the tools. And the best candidates surely aren’t the ones who know every tool. The best candidates manage to dovetail their efforts with the efforts of other humans who are aligned with a common transformation purpose.
There are so many workforce skills companies need besides tool fluency. Every year, new tools emerge that some will want to make use of. Consider the matter this way: Three times as much code is being written than would need to be written in a findable, accessible, interoperable and reusable (FAIR) knowledge model-driven development environment.
Companies need to acknowledge that on-the-job training is a necessity in a number of cases, and that most applications are commoditized and at least partially redundant these days. If companies focus on the data instead of the apps, they won’t need to hire as many app specialists.
In an AI era that should be led by humans, reorienting people, process and data (including knowledge modeled data for hybrid AI) in light of all the adoption and tech change challenges should be at the top of the priorities list. That means identifying new roles and dividing up responsibilities within established roles so that data scientists, engineers and developers aren’t overburdened.
The leadership and management rationalization challenge
Then there are roles that are buried and under-resourced, say in data, content, or knowledge management. Leaders need to find the talent that’s been buried, and unearth and support it. Leaders need to do all the blocking and tackling necessary to protect and enhance these roles. Otherwise, the data/content/knowledge and relatedness map that brings these together won’t be a managed resource.
Many other established roles staffed by technologists often have a management component that needs to be rationalized. The question becomes, who’s leading the organization proactively to institute effective change? Is current leadership able to take charge, or are they opposed to real transformation?
Is the management tier overpopulated? Is there enough fresh energy and new thinking flowing into a given enterprise?
Several models exist to facilitate role transformation and rationalization. Many of these open the door to job seekers who want to land entry-level, assistant, associate, or specialist roles. Not everyone wants to be a manager. Many people want to learn a trade and get really good at it. These roles suggest a path for AI-enabling trades:
- Apprenticeships
- Stewardship (particularly data)
- Assistants
- Associates
- Technicians
- Master specialists
All of these classes of roles should be staffed up, once the management tier has been rationalized.
Leadership, to be effective, needs to take this people challenge on board before embarking on a holistic, internal AI initiative.
The characteristics of an AI-ready transformed organization
Once the company is backing and fueling a renewed workforce vision, the task-by-task, humanistic AI-enabled innovation work can begin.
Innovation pathfinding: Companies need to organize more guerrilla teams and identify specific pain points that can be addressed quickly with small, innovative, tactical approaches. These teams should be led by change agents, the foxes, rather than the hedgehogs. The hedgehogs are there to keep things moving once they’re been established. The foxes are the curious ones who can help innovate.
The successful guerrilla teams discover patterns of adoption, some of which will be feasible and otherwise scalable.
Data-centric digital transformation: This is real transformation, not just going through the motions and commissioning or subscribing to more SaaS apps. Data-centric transformation means innovating and managing at the data layer and using that transformation to reduce, if not eliminate, duplication and waste at the app layer. AI needs access to quality, contextualized, relevant data.
Systems design and transformation. Not everyone is an analyst. Some are synthesists, people who envision how to connect the dots in a transformed systems architecture and also help facilitate means of boundary crossing between departments and organziations. Support the synthesists.
AI data asset quality and management focus: AI needs the right contextualized data model (ontology) and a scalable means of integration and interoperation (an ontology model-driven knowledge graph). The focus here needs to be on findable, accessible, interoperable, and reusable data and declarative code
Corporate silos guarded by data cartels currently prevent 99 percent of large enterprises from tapping into and reusing the data they do have.
Envisioning the optimized workforce and transformed system first
It’s ridiculous in any case to hire for one developer or engineering role when the challenge is at the system level.
Let’s think about the future workforce proactively, and stop reacting to every AI company who promotes their product as a magic bullet. Take the real transformation challenge on, starting with the kind of people who can help most.
Wild West out there, the Wild, Wild West. AI smells like the beginning of the dot com era, bang, bust, stabilization, centralization.
. ‘AI needs access to quality, contextualized, relevant data”
People need that. With the help of AI.
Why do people are so blind to be ready to plunge into loops that support AI
Instead of demanding and engineering situations that are for their benefit
instead of the Machines and the man behind the machines
The AI Winter is too Aways Imminent not just Ai
In the current Fools Gold Rush the shower makers will loose
Let’s avoid it precipitating a Nuclear Winter
Let’s hope we the people realise that human potential itself is precious
Or as Gilder said the source of all value is knowledge and we are together are the only true source