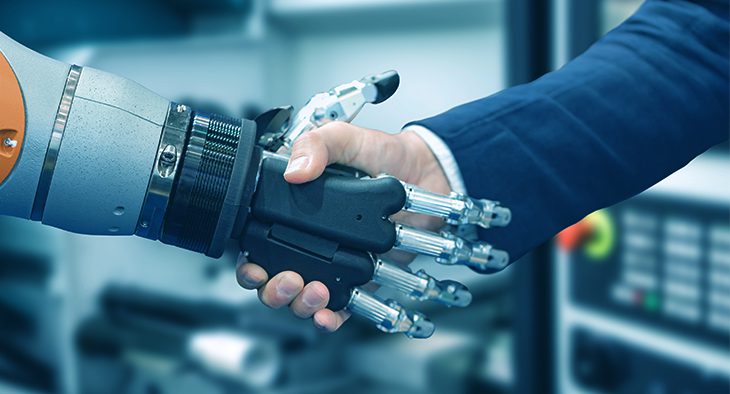
The need to use predictive analysis and modeling in forecasting the growth of data has been brought about by how great the volume and variety of data there currently is. According to Gartner, “Data preparation is an iterative and agile process for exploring, combining, cleaning, and transforming raw data into curated datasets for self-service data integration, data science, data discovery, and business intelligence/analytics.”
Read more: What is data preparation, and why is it important?
What is trustable data?
Trustable data can be defined as data that comes from specific and trusted sources and is used according to its intended use. It is delivered in the appropriate format and time frames for specific users.
Trustable data helps in good decision-making processes. The properties mentioned above make data trustworthy for good decision-making.
What are the trust factors of data?
Trustable data is considered good only when it meets some basic requirements. One of the widely recognized ways to access data is the use of data quality dimensions.
Some of the trust factors that constitute data quality include;
Accuracy
Data accuracy refers to the extent to which data is considered true, can be relied on, and is error-free. In artificial intelligence, where an algorithm in context would need a large volume of data to help decision-making, the data’s accuracy would be considered the main factor. Data accuracy in any setting reflects the actual data state that the user expects in a real-world representation of the gathering and processing stages.
Consistency
Data consistency is the extent to which data is presented similarly and as compatible as the previous data. Consistency also applies to different aspects of data, including; data values being similar in all instances, data attributes, and data types having a basic structure, and data sources having no contradictions.
Completeness
The completeness of data refers to the extent to which a given dataset contains all relevant data expected by the user, and all mandatory data attributes are available.
Similarly, in Artificial Intelligence, data is considered complete only when it reflects all possible states of the user population to avoid biases.
Security
Data security refers to the degree to which data coming from different sources is very secure and that it can hold even sensitive information.
Usefulness
Data usefulness refers to the extent to which data, when processed, applies to the actual context intended for its user or consumer. Generally, data usefulness is achieved when all other data quality dimensions, including reliability, completeness, consistency, etc., are met.
Privacy
Data privacy prescribes that assurances are made to data owners or users that it is lawfully used in compliance with data protection regulations and the General Data Protection Regulation (GDPR)
Reliability
Data reliability refers to the extent to which data from a source can be trusted to carry the intended information.
Interpretability
The interpretability of data refers to the degree to which data is in a proper language and state, is meaningful and the end-user can easily understand the symbols used.
Why do you need trustable data?
Most artificial intelligence and machine learning algorithms require data formatted in a very specific way. This means datasets generally require considerable preparation before yielding a useful purpose. Some of the datasets contain values that are inconsistent, missing, invalid, or in some instances, difficult for an algorithm to process. When data is missing, the algorithm is not able to use it. If invalid, the algorithm will produce less accurate or misleading results. Some datasets could be relatively clean but need to be adjusted. Many datasets also lack useful business context, therefore requiring feature enrichment. It is considered that a good data preparation process produces clean and well-curated data. Clean data leads to more practical, accurate model results.
Trustable data propels innovation as well as accelerates competitive advantage.
Conclusion
Trustable data is a strategic asset for every enterprise. This is why organizations need to invest in expertise, processes, and appropriate technology to ensure their data is trustable, sound, accurate, and reliable. Trustable data is used to maximize all that is good for an organization while fostering trustable business relationships with its customers, clients, partners, and its employees.
Trustable data can improve an organization’s outcomes and provide the foundation to innovate and transform its operations when managed and cultivated correctly.
Looking for a solution to make your organization’s data trustable? Try the DQLabs trial today