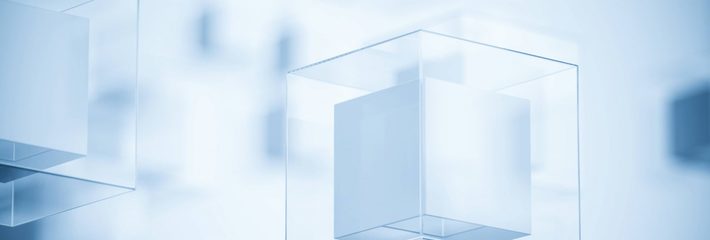
Machine learning solutions continue to incorporate changes into businesses core processes and are becoming more prevalent in our daily lives. The global machine learning market is predicted to grow from $8.43 billion in 2019 to $117.19 billion by 2027.
Despite being a trending topic, the term machine learning is often used interchangeably with the concept of artificial intelligence. In fact, machine learning is a subfield of artificial intelligence based on algorithms that can learn from data and make decisions with minimal or no human intervention.
Many companies have already begun using machine learning algorithms due to their potential to make more accurate predictions and business decisions. In 2020, $3.1 billion in funding was raised for machine learning companies. Machine learning has the power to bring transformative changes across industries.
With machine learning being so prominent in our lives today, its hard to imagine a future without it. Here are our predictions for the development of machine learning in 2021 and beyond.
Quantum computing can define the future of machine learning
Quantum computing is one advancement that has the potential to boost machine learning capabilities. Quantum computing allows the performance of simultaneous multi-state operations, enabling faster data processing. In 2019, Googles quantum processor performed a task in 200 seconds that would take the world’s best supercomputer 10,000 years to complete.
Quantum machine learning can improve the analysis of data and obtain more profound insights. Such increased performance can help businesses to get better results than via more traditional machine learning methods.
So far, there is no commercially ready quantum computer available. However, a handful of big tech companies are investing in technology, and the rise of quantum machine learning is not that far off.
AutoML will facilitate the end-to-end model development process
Automated machine learning or AutoML is automating the process of applying machine learning algorithms to complete real-life tasks. AutoML simplifies the process so that a person or business can apply complex machine learning models and techniques without being an expert in machine learning.
AutoML enables a wider audience to use machine learning, which indicates its potential to change the technology landscape. A data scientist can use AutoML to their benefit, for instance, to quickly find the algorithms they can use or whether any algorithms have been missed. Here are some stages of development and deployment of a machine learning model that AutoML can automate:
- Data pre-processing improve data quality, transform unstructured data into structured data with the help of data cleaning, data transformation and data reduction, etc.
- Feature engineering use automation with machine learning algorithms to help create more adaptable features based on the input data.
- Feature extraction use different features or datasets to produce new features that will improve results and reduce the size of data processed.
- Feature selection choose only useful features for processing.
- Algorithm selection and hyperparameter optimisation automatically choose the best possible hyperparameters and algorithms.
- Model deployment and monitoring deploy a model based on the framework and monitor the condition of the model via dashboards.
Industries to watch for machine learning disruption
Healthcare and pharma
The healthcare industry generates massive amounts of data. Applying machine learning techniques can greatly contribute to better predictions and treatments.
- Disease prediction. The development in technologies improves prediction and prevention of possible disease, rather than treatment after diagnosis. The traditional approach for disease prediction includes a limited number of variables such as age, weight, height and gender, etc. The machine learning approach enables analysis of a wider range of variables based on studies carried out, patient demographics, health records and other sources, which can lead to better results for disease prediction.
- Drug discovery. Drug development is time-consuming and costly. According to a recent study, the median cost of bringing a new drug to market is $985 million. Using datasets with a drug compound’s chemical structure, machine learning algorithms can predict the impact the drug compound could have on different cell lines and genes and detect possible side effects. Using machine learning will speed up the drug testing time, accelerating the process of bringing a drug to market.
- Electronic health records. Electronic health records (EHRs) include huge amounts of data in different forms and from different sources. The application of machine learning techniques, such as natural language processing and image processing, can help to convert the data into a standard format. Machine learning-fuelled EHRs have the potential to streamline and enhance the process of identifying clinical patterns, which can lead to better prediction results.
Manufacturing
Manufacturers are only in the early stages of adopting machine learning. In 2020, only 9% of survey respondents were leveraging artificial intelligence in their business processes.
Machine learning tools can be used for various manufacturing purposes, including monitoring equipment performance and condition, predicting product quality and forecasting energy consumption. With the ongoing advancements in the field of machine learning, we can expect more robots in manufacturing premises in the near future.
Among many other benefits, using machine learning in manufacturing can reduce costs, enhance quality control and improve supply chain management.
Automotive and self-driving vehicles
Tesla, Waymo and Honda are among the car development companies exploring the possibility of deploying self-driving cars. And while manufacturers have already presented cars with partial automation, fully autonomous vehicles are still under development. Machine learning is one of the main technologies that can help to turn these dreams into reality.
Deep learning, a class of machine learning algorithms, can help improve perception and navigation in autonomous vehicle manufacturing, including path planning, scene classification and obstacle and pedestrian detection.
As new technologies continue to unfold, machine learning algorithms can be used more productively. The future of machine learning will open multiple opportunities for businesses. Make sure that your business is ready to make the most of what is coming.
Looking for some advice to help you learn more about machine learning? Get in touch!
Originally published at the ELEKS blog.