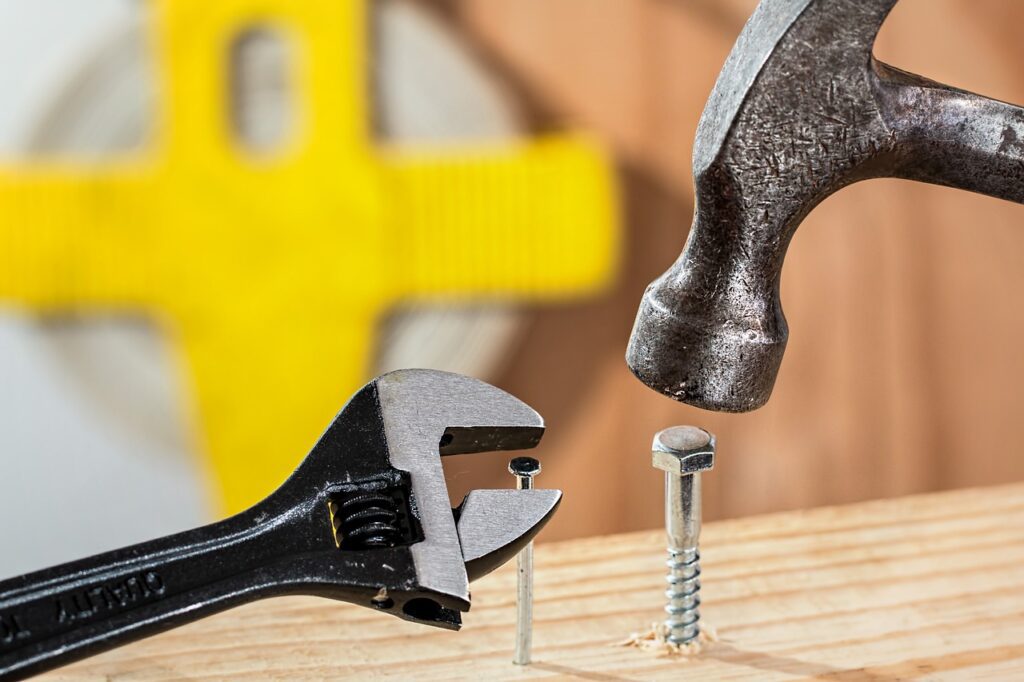
Image by Steve Buissinne on Pixabay
Lowest common denominator (LCD) data science is the unthinking variety of data science that doesn’t question the prevailing wisdom or try to counter it. The unfortunate reality is that LCD data science is much more common and triggers much more damaging side effects than the alternatives.
Consider some symptoms of a society suffering from the current dominance of LCD data science:
The chatbot wow factor and a willingness to be deluded by gen AI’s allure
At this year’s South by SouthWest conference, Microsoft’s VP of AI and Design John Maeda observed that chatbots have been fooling humans since the 1960s. Conversation is often cryptic, leading humans to fill in the gaps with assumptions that aren’t reflective of what the AI’s actually doing or why. As a result, bots can seem smarter than they really are.
Maeda said chatbots for decades have been adept at extending conversations merely by picking up keywords from a human’s conversation and throwing the keywords back at them in the form of questions phrased in ways that imply the bot is genuinely curious.
It’s not difficult for bots to borrow the therapist’s approach to getting a patient to talk about their problems. For example, the bot hears the human mention “mother”. The question in response becomes, “Tell me about your mother.”
Lately, even some trained scientists who’ve been wowed at the recent question answering success of generative AI have been asserting that bots seem to be “sentient” these days. Skeptics, meanwhile, counter that bots are really just doing an elaborate form of autocomplete-style guesswork, and that they’re still hallucinating quite a bit.
Just because chatbots provide useful answers to questions doesn’t prove they understand what the answers they’re delivering mean….or how they relate to the nuances behind the question.
How AI-enabled automation can lower overall business performance
In January 2024, the International Monetary Fund (IMF) released a Staff Discussion Note entitled “Gen-AI: Artificial Intelligence and the Future of Work.” One of the observations the authors offered was this one:
In advanced economies, about 60 percent of jobs are exposed to AI…. Of these, about half may be negatively affected by AI, while the rest could benefit from enhanced productivity through AI integration.
One way to read this sort of assertion with a critical eye is to think about current automation-driven practices and how the quality of those processes has further declined now that AI-enabled software is the norm.
Take the typical HR department’s worst hiring tendencies and how they’re magnified by AI. In a time when popular business books like David Epstein’s Range: Why Generalists Triumph in a Specialized World have proclaimed the value of generalists, the vast majority of job postings online are designed to filter on a laundry list of a dozen or more specialties. The generalists may well be valuable, but what’s the likelihood their application will make it to the hiring manager for consideration?
Much more likely is the prospect that applications from abstract thinking generalists will be filtered with the help of AI out of consideration, precisely because these generalists may not have X years of experience in the Y specialization using the Z software package. More thoughtful AI, by contrast, would steer clear of reducing hiring to a mere resume-to-requirements text matching exercise.
Repeating the lie that a hard problem is solved doesn’t make it so
Timnit Gebru, Founder & Executive Director at The Distributed AI Research Institute, recently shared a video clip from a 1984 episode of a Silicon Valley PBS affiliate’s TV program The Computer Chronicles as an example of the kind of AI hype that’s been around for forty years or more. During the program, one of the consultants interviewed proudly announced,“We’ve reached a watershed, where it’s no longer very expensive or very difficult for individuals with no technical background to build [AI] systems and apply them usefully.”
The truth is that systems thinking is hard, and that most companies fail to support a forward-looking architectural vision. Systems thinking should be a salaried discipline in its own right, one that needs generalists who can abstract, synthesize and clear a path via data-centric architecture for the process improvements that analytics results demand. Business leaders need to fund and nurture 20 different roles, several of which involve architects at different levels, to tackle AI, not just four, and those roles need to represent a full range of intellectual diversity, thinkers with many different styles.
Improving AI implies the need for a radically different approach to data + knowledge management
Many of the skills these roles demand already exist inside the largest enterprises. The problem is that the people with these skills are siloed in dedicated data management, content management and knowledge management departments.
The people from these three departments could instead band together with a single, unified approach to structured and unstructured data management that’s feasible now with a knowledge graph-based data architecture. Leadership needs to de-silo their organizations and empower visionary architects to implement such a unified approach. To fund such an effort, leaders can reallocate budgets from underutilized, siloed application suites.