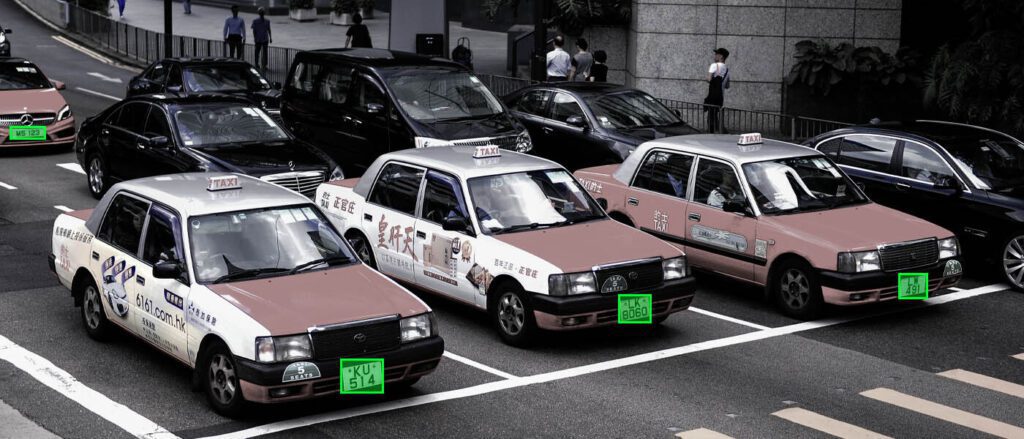
In various fields, such as traffic management, law enforcement, and parking management, license plate recognition is a crucial application of computer vision that is used to analyze license plates. In this article, we will review the Chinese City Parking Dataset (CCPD), which is one of the most widely used computer vision datasets for tasks that are specifically related to license plate recognition. Our aim in this article is to explore the CCPD dataset, its unique characteristics, and its potential contribution to the advancement of license plate recognition technology, in order to identify license plates in real-time.
The CCPD Dataset: An Overview
There are numerous images in the CCPD computer vision dataset that feature Chinese license plates that were captured in real-life scenarios exploiting the CCPD dataset in a comprehensive manner. This is one of the largest publicly available license plate datasets in the world, containing over 300,000 images of license plates. Annotations, which may include license plate numbers, locations, and orientations of each image in the dataset, are included with each image.
A Description of the Characteristics of the Dataset
License plate recognition research can benefit from the CCPD computer vision dataset due to several unique characteristics.
a. Diversity:
There is a wide range of variations that can be observed in the dataset, including fonts, colors, sizes, and styles, thus illustrating the wide range of Chinese license plates. The diversity among the datasets that are used to train license plate recognition models ensures the robustness and generalizability of the model.
b. Scenarios in Real-World:
A variety of lighting conditions, weather conditions, angles, and lighting conditions are captured in the CCPD dataset. As a result of this realism, license plate recognition applications are able to illustrate the challenges they face.
c. Large-Scale Annotation:
In addition to the detailed annotation of the dataset, the license plate bounding boxes are accurate and accompanied by text annotations. Researchers are able to effectively train and evaluate license plate recognition models when they have this level of annotation.
The CCPD computer vision dataset is applicable to a wide range of applications
License plate recognition technology can benefit greatly from the CCPD dataset:
a. Training and Evaluation of AI & ML Models:
A license plate recognition model that uses CCPD Computer Vision datasets can be trained and evaluated by researchers and developers using the dataset. As a result of the large-scale annotations, we are able to produce high-quality training data that allows us to develop algorithms that are reliable and accurate.
b. Comparisons and/or Benchmarking:
License plate recognition algorithms are evaluated using the CCPD dataset. Research can be conducted by comparing and evaluating different models, techniques, or methods of pre-processing by using standardized evaluation metrics.
c. Development and Innovation of Algorithms:
In the license plate recognition field, the CCPD dataset stimulates innovation by stimulating the development of new algorithms. Using the dataset, researchers can develop new approaches to improve the accuracy, speed, and adaptability of license plate recognition systems, such as deep learning-based methods in order to increase their speed, accuracy, and adaptability.
Developing and expansion of business in future
There is no doubt that the CCPD dataset will continue to expand and improve as license plate recognition technology continues to advance. Future development of the system may include an even greater diversity of license plate samples, for example, different countries, regions, or specialized license plate categories. In addition to the existing annotations, the dataset can be extended with additional annotations, such as a description of the vehicle type or information about an attribute, to facilitate the development of more extensive analyses and research.
Final thoughts
As a result of the use of the CCPD dataset, license plate recognition technology has the potential to advance significantly. License plate recognition models can be trained and evaluated by using this dataset, which contains a wide variety of license plate types and models, as well as large-scale annotations and real-world scenarios. By utilizing the CCPD dataset, researchers and developers will be able to create efficient and reliable license plate recognition systems that improve accuracy, improve efficiency, and drive innovation.