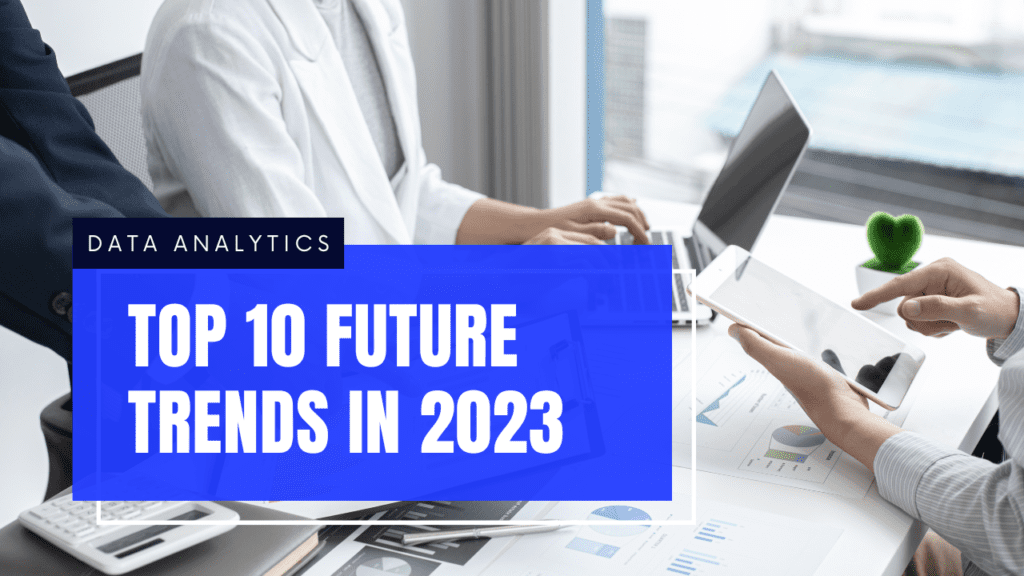
In an era where the landscape of business is evolving and changing at a rapid pace, data collection & analysis often operate as pivotal factors in shaping the destiny of each new market segment, whether it be the healthcare sector, decentralized work, an online company like Amazon, an online customer service network, or even an online banking service.
A few of the key trends driving today’s accelerating market include advances in Big Data Analytics, Data Science, and Artificial Intelligence that are transforming the way businesses are run across the world. With more businesses implementing data-driven models, the data analytics industry is growing steadily. Data analytics played an even more important role in forecasting the future when the COVID-19 pandemic broke out since more and more sectors resorted to analyzing and interpreting data in order to estimate what was going to happen in the future. Increasingly, analysts and businesses are coming together with the goal of improving, simplifying, and enhancing how data can be used.
Data analysts appear to be in a roaring ebb in recent years with a steady rise in the number of data analyst job listings. In this article, we will take a look at the top 10 trends in data analytics that have revolutionized the way we deal with everything from education to economics to the environment and how we use data to make more informed decisions.
Let us examine some of the Data Analytics trends that have become an integral part of the industry in recent years.
Top 10 Future Trends in Data Analytics
1. Artificial Intelligence:
In recent years, there have been a number of technological advances that have revolutionized the way businesses around the world operate, including machine learning, artificial intelligence, robotics, and automation, among others. Data analysis is rapidly evolving with AI, upping human abilities at both the personal and professional levels, as well as assisting businesses to gain a better understanding of the data they collect. The business landscape has changed dramatically since COVID-19, rendering historical data somewhat obsolete. The market now has a vast array of new scalable and clever AI and machine learning techniques capable of dealing with small data sets, unlike traditional AI techniques.
In the long run, businesses would benefit significantly from AI systems by formulating processes that are efficient and effective. There are many ways in which artificial intelligence can be used to boost business value. This includes forecasting customer demand to increase sales, improving warehouse stocking levels, and speeding up delivery times to increase customer satisfaction. A good AI system can be highly adaptive, protect personal information, be faster, and provide a higher return on investment as well.
2. Data Democratization:
Data democratization aims to empower all members of an organization, regardless of technical expertise, to interact comfortably with data and to discuss it confidently, ultimately leading to better decisions and customer experiences. Today, companies are embracing data analytics as a core element of any new project and a key business driver. With data democratization, non-technical users can gather and analyze data without the assistance of data stewards, system administrators, or IT staff.
Artificial intelligence, or AI for short, is also proving beneficial across the globe as a tool for promoting justice, ensuring inclusive education, as well as improving the quality of life for disadvantaged communities. With instant access to and understanding of data, teams can make faster decisions. A democratized data environment is an essential aspect of managing big data and realizing its potential. Today, businesses that equip their employees with the right tools and understanding are better able to make decisions and provide excellent customer service.
3. Edge Computing:
With the advent of 5G, edge computing has created a wealth of opportunities across a wide array of industries. In the world of edge computing, computing and data storage can be brought closer to where the data originates, making data more accurate and more manageable, reducing costs, providing faster insights and actions, and allowing continuous operations to be carried out. There is no doubt that the pace of data processing at the edge is going to increase tremendously, maybe to 75% in 2025 from 10% currently. IoT devices that are embedded with edge computing are capable of speed, agility, and greater flexibility. It can also perform real-time analytics and enable autonomous behavior.
Edge computing consumes less bandwidth and is, therefore, an efficient way to process massive amounts of data. In addition to reducing development costs, it facilitates the operation of software from remote locations.
4. Augmented Analytics:
In today’s predictive analytics world, augmented analytics is one of the main trends you are going to see. Augmented analytics uses machine learning and natural language processing to automate and process data as well as derive insights from it that would otherwise be handled by a data scientist or specialist. An augmented analytics solution can help business users and executives better understand their business context, ask relevant questions, and uncover insights more quickly. Additionally, augmented analytics helps analysts and advanced users perform more thorough analysis and data preparation tasks, even if they don’t possess deep analytical expertise.
5. Data Fabric:
The data fabric is a set of architectures and services that provide consistent functionality across a variety of endpoints that span multiple clouds and deliver an end-to-end solution. As a powerful architecture, it creates a common data management practice and practicality that we can scale across a wide range of on-premises cloud and edge devices. Finally, data fabric improves the use of data within an organization and reduces design, deployment, and operational data management tasks by 70%. As the pace of business is constantly increasing and data becomes more complex, more organizations are going to rely on this framework because it is easy to use, easy to repurpose, and can be combined with data hub skills, different integration styles, and other technological advancements.
6. Data-as-a-Service:
Data as a service, or DaaS for short, is a cloud-based software tool used to analyze and manage data, such as data warehouses and business intelligence tools, which can be run from anywhere and anytime. In essence, it allows subscribers to access, use, and share digital files online via the internet. Since the COVID-19 pandemic broke out, the DaaS industry in the healthcare sector has seen growth opportunities. As users have increased access to high-speed internet, it is expected that DaaS will have a wider reach as well. DaaS will ultimately lead to a greater level of productivity within the business. The use of DaaS in big data analytics will simplify business review tasks for analysts and make sharing data easier across departments and industries. Since more and more businesses are turning to the cloud to modernize their infrastructure and workloads, DaaS has become a more common method of integrating, managing, storing, and analyzing data.
7. NLP (Natural Language Processing):
NLP is one of the many subfields of computer science, linguistics, and artificial intelligence that has developed over the years. Basically, this discipline focuses mainly on the interaction between human languages and computers, and in particular, how to program computers in such a way that they are capable of identifying, analyzing, and processing a large amount of information derived from natural languages, thereby improving their intelligence. NLP aims to read and interpret human language. It is anticipated that NLP will become increasingly important in monitoring and tracking market intelligence as businesses utilize data and information to formulate future strategies. NLP techniques such as syntactic and semantic analysis require algorithms that extract the important information from every sentence using grammar rules. As opposed to semantic analysis, which deals with the meaning of the data or text, syntactic analysis focuses on the sentences and grammatical issues associated with the data/text.
8. Data Analytics Automation:
Data Analytics Automation refers to automating analytical tasks with computer systems and processes to minimize human involvement. The automation of data analytics processes can have a significant impact on the productivity of many enterprises. Furthermore, it has paved the way for analytic process automation (APA), known to assist in unlocking predictive and prescriptive insights for faster wins and higher ROI. The technology will accelerate productivity and improve data utilization. This tool has one notable feature: it can search categorical data to create a set of relevant features. Among the most popular data analytics software are IBM Analytics, Apache Spark, Apache Hadoop, and SAP.
9. Data Governance:
Data governance is the process of ensuring high-quality data and providing a platform to enable data sharing securely across an organization while complying with any regulations related to data security and privacy. By implementing the necessary security measures, a data governance strategy ensures data protection and maximizes the value of data. Having no effective data governance program can result in compliance violations and fines, poor data quality, influencing business decisions, problems finding the right data, delays in analyzing, missed opportunities, and poorly trained AI models. By democratizing data, it has the potential to embed data into every aspect of decision-making, as well as create trust among users, increase the value of brands, and reduce the likelihood of compliance violations.
10. Cloud-based Self-Service Data Analytics:
Self-service data analysis has become the next big thing in data analytics through cloud-based management systems. Human resources and finance leaders are leading this movement, investing heavily in cloud-based technology solutions that allow all users to have direct access to the information they need. Self-service analytics puts data directly in the hands and heads of the users whom it is intended to serve – they are the ones who need it. With self-service analytics powered by the cloud, you can boost your competitive advantage and increase your efficiency. Incorporating cloud-based analytics into your financials or HR platform ensures users have only access to the data they need. Self-service analytics can ultimately transform every aspect of a company from the inside out. As an example, the Chief Financial Officer (CFO) might provide financial information to the HR department, the marketing department, the products department, the sales department, and the operations department so they can conduct their own data discovery and visual analysis and determine the effectiveness of their actions.
Conclusion
With the continued evolution of the digital world, startups, SMEs, and large organizations are increasingly using data analytics to enhance customer experience, reduce costs, optimize existing processes, and reach a wider audience. Apart from these, big data is attracting a good deal of interest from many companies due to its ability to enhance the security of critical information. More and more data analytics trends are likely to emerge and flourish in the years 2022, 2023, and beyond, as we move forward with the development of artificial intelligence.
Based on the top 10 analytics trends discussed in this article, we can conclude that businesses are rapidly becoming data-centric in the business world as a whole. With the advancement of artificial intelligence (AI), the Internet of Things (IoT), and automation in our daily lives, it is essential to recognize these trends, as they can help organizations cope with the many changes and uncertainties that are becoming more prevalent. Identify, experiment with, and then aggressively invest in key trends that are important and aligned with your strategic business goals. Make sure you pay attention to the current trends so you don’t get caught out by future technologies.