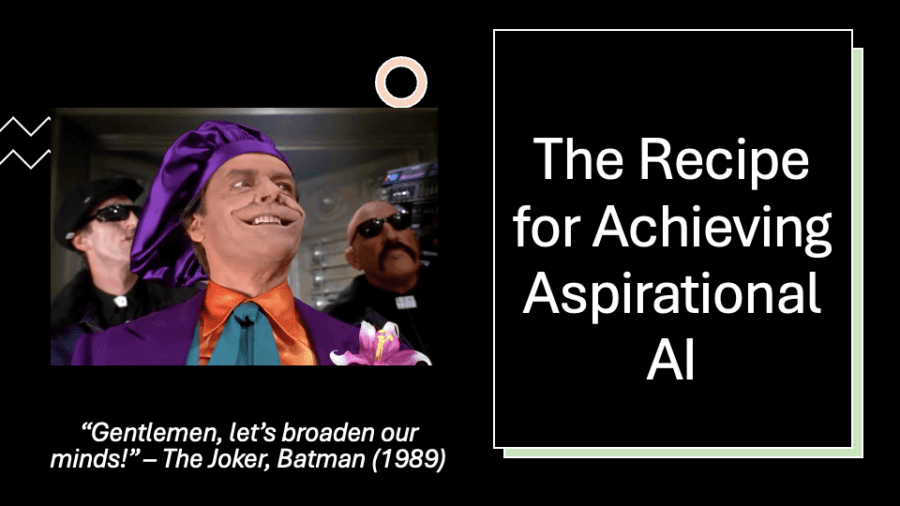
There has been much recent buzz about AI’s potential decline. Articles such as “AI Stock Market Bubble May Be About to ‘Burst'” from Newsweek and “From Boom to Burst the AI Bubble is Only Heading in One Direction” from The Guardian paint a grim picture of AI’s future. Much of this concern stems from misunderstandings about its capabilities.
On the other hand, the integration of Generative, Analytical, Causal, and Autonomous AI has the potential to tackle some of society’s most difficult problems. An integrated AI approach, which I refer to as “Aspirational AI,” can address some of our most challenging business, operational, and societal issues in a meaningful, responsible, and ethical manner.
If the conversation only revolves around using Generative AI (GenAI), our solutions will be incomplete, inaccurate, and unstable. Why focus only on GenAI when we have other types of AI at our disposal? It’s like saying we will build the Eiffel Tower (thanks, Paris Olympics) using only hammers. We should leverage the full range of AI tools and capabilities to achieve our aspirational goals.
Aspirational AI is important because it represents a comprehensive and forward-thinking approach to utilizing AI. Instead of focusing narrowly on the capabilities of Generative AI, it emphasizes the synergy of four distinct types of AI, each contributing unique capabilities in our search to achieve more aspirational goals.
How Do We Achieve Aspirational AI?
To achieve Aspirational AI, we must embrace the following key ingredients for success:
- Ingredient #1: Broaden the AI Conversation: We should blend Generative AI with Analytical, Causal, and Autonomous AI to unleash the full potential of AI to power our business and operational strategies.
- Ingredient #2: Embrace a Collaborative Value-creation Framework: Use frameworks like the “Thinking Like a Data Scientist” methodology to combine these AI tools with the organization’s vast tribal knowledge to drive new sources of value.
- Ingredient #3: Empower Innovative Exploration: Encourage the innovative use of Generative AI tools to expand upon organizational knowledge and foster continuous learning and adaptation between humans and algorithms.
In this blog, we will explore these ingredients in detail and illustrate how they can be applied to create a more impactful and ethical use of AI. By adopting this Aspirational AI approach, we can unlock the true potential of AI technologies and make significant strides toward solving the big, hairy, wicked problems facing our world today.
Ingredient #1: Understand the Four Types of AI
In the blog “Synergy of Generative, Analytical, Causal, and Autonomous AI,” I introduced the four types of AI (Figure 1):
- Generative AI: The “How.” Focused on creation, Generative AI builds upon patterns found in unstructured and structured data sets to generate new content (text, data, images) based on the data distributions and relationships found in the data upon which it was trained.
- Analytical AI: The “What?” Focused on interpretation, Analytical AI analyzes existing data to uncover patterns and trends, making predictions and recommendations on the next best action based on trends and patterns in the data
- Causal AI: The “Why.” Focused on understanding, Causal AI uncovers and quantifies cause-and-effect relationships to provide insights into the underlying reasons behind observed patterns and phenomena. It is essential for making informed decisions based on understanding the true impact of variables and avoiding mistaking correlation for causation, which can lead to potential risks when making predictions based on non-causal data.
- Autonomous AI: The “Do.” Focused on independent operation and continuous learning, Autonomous AI systems are designed to perform tasks, learn, adapt, and make real-time decisions with minimal human intervention. Autonomous AI excels in dynamic environments requiring rapid, continuous decision-making and adaptability.
Check out “How to Transform Your ML Models with Generative AI” for ideas on how to leverage GenAI as a “Mix-In” (Yummy) to improve the accuracy and relevance of your current AI/ML models.
Ingredient #2: Build upon a Collaborative, Value-creation Framework
The “Thinking Like a Data Scientist methodology” provides a collaborative framework to thoroughly define the business and stakeholder requirements for successfully applying data and AI to deliver more relevant, meaningful, responsible, and ethical outcomes (Figure 2).
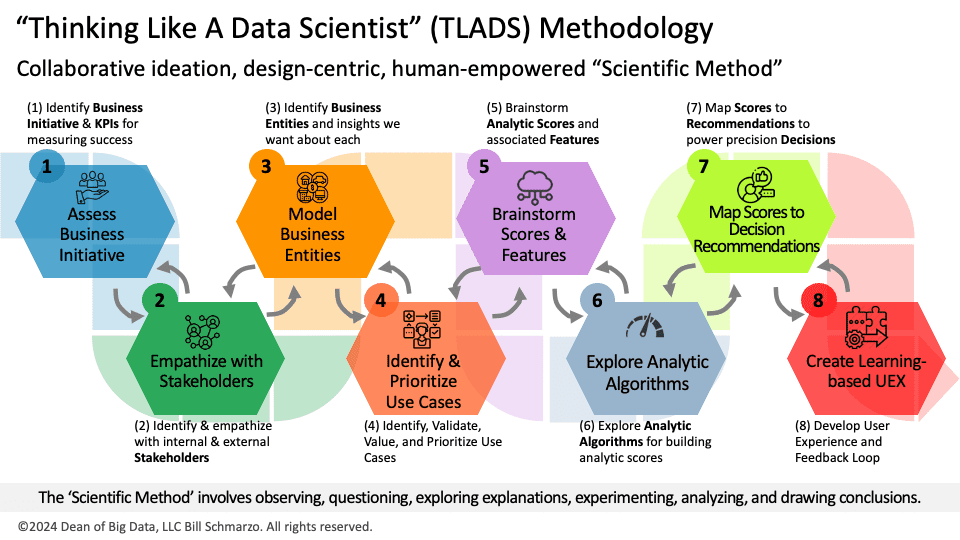
Figure 1: The “Thinking Like a Data Scientist” (TLADS) Methodology
The TLADS (Thinking Like a Data Scientist) methodology provides a structured approach to framing business, operational, or societal initiatives.
- Step 1 involves conducting a comprehensive assessment of the initiative. This includes identifying the desired outcomes, benefits, potential obstacles, risks of failure, and potential unintended consequences. Crucially, this step also involves defining the key performance indicators (KPIs) and metrics that will be used to measure the initiative’s progress and success. This thorough evaluation ensures that all aspects of the initiative are considered, laying a solid foundation for its execution.
- Step 2 uses design thinking techniques and concepts to understand the primary stakeholders affected by the initiative. This encompasses grasping the initiative’s importance to them (WITFM—What’s In It For Me), their desired outcomes, and the crucial decisions they must make to achieve those outcomes. Additionally, this step involves determining the KPIs and metrics stakeholders will use to evaluate decision and outcome effectiveness. Focusing on stakeholders’ perspectives ensures the initiative aligns with their needs and expectations.
- In Step 3, we identify the primary business entities, human or device, whose predicted tendencies and behaviors will be captured and managed. This step is at the core of nanoeconomics, focusing on individual entities’ predictive behavioral and performance tendencies. Understanding these entities can better anticipate their actions and reactions, leading to more informed and effective decision-making.
- Step 4 involves identifying the specific actions or use cases the organization can take to achieve the initiative. This includes determining the stakeholders impacted by each use case, critical decisions, desired outcomes, and the KPIs and metrics that will be used to measure use case progress and success. This step translates the strategic business initiative into actionable use cases, ensuring that each use case is aligned with the initiative’s strategic goals.
Upload the information captured in the TLADS process into ChatGPT to prep ChatGPT for further use case exploration and analysis (Figure 3).
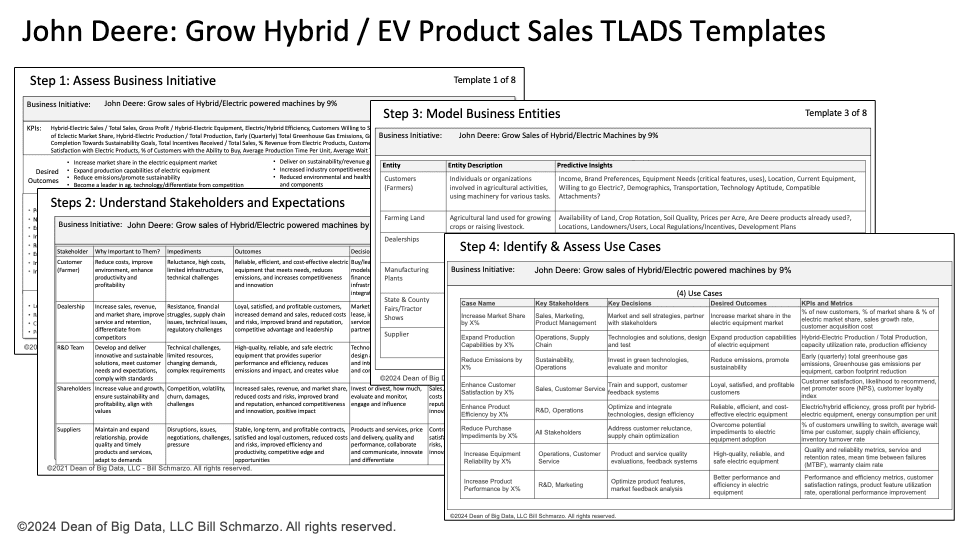
Figure 2: Key TLADS Templates for “Grow Hybrid/EV Product Sales” Business Initiative
Leverage TLADS Templates To Create a Lightweight RAG
Uploading the completed TLADS templates into a ChatGPT thread is a lightweight form of Retrieval-Augmented Generation (RAG), a framework combining retrieval-based and generation-based models. Uploading TLADS templates to a ChatGPT thread allows ChatGPT to access specific, structured information and then use that information to generate informed responses. The benefits of this RAG-lite include:
- Enhanced Contextual Understanding: Leverage detailed TLADS information to better understand the business initiative, stakeholders, and desired outcomes.
- Informed Responses: Generate more accurate and relevant recommendations, predictions, and insights.
- Dynamic Interaction: Enable interactive and iterative exploration and validation process.
- Efficiency and Relevance: Avoid hallucinations and generic responses and provide more tailored and actionable insights.
This approach utilizes the principles of RAG by combining the retrieval of structured information from the TLADS templates with the generative capabilities of ChatGPT, creating a powerful tool for dynamic and informed decision-making.
Ingredient #3: Empower Innovative Exploration
After creating our lightweight TLADS RAG environment, let’s leverage ChatGPT to explore blending Generative, Analytical, Causal, and Autonomous AI to supercharge our prioritized use case. Out of the TLADS methodology, let’s say that the top priority use case was “increase market share.” Here are some prompts to help us ideate where and how we can leverage the different types of AI to achieve that use case.
- ChatGPT Prompt: Based upon Bill Schmarzo’s blog “Synergy of Generative, Analytical, Causal, and Autonomous AI https://www.datasciencecentral.com/synergy-of-generative-analytical-causal-and-autonomous-ai/, create an analysis in bullet point format of how each of these four different types of AI – generative, analytical, causal, and autonomous – impacts the [increase market share] use case. Include a summary of the benefits of synergizing the four types of AI at the end of the use case. The output format should be a quick summary or description of the use case and then bullet points for the 3 to 4 ways generative, analytical, causal, and autonomous AI impacts that use case[1].
The results are in Figure 4.
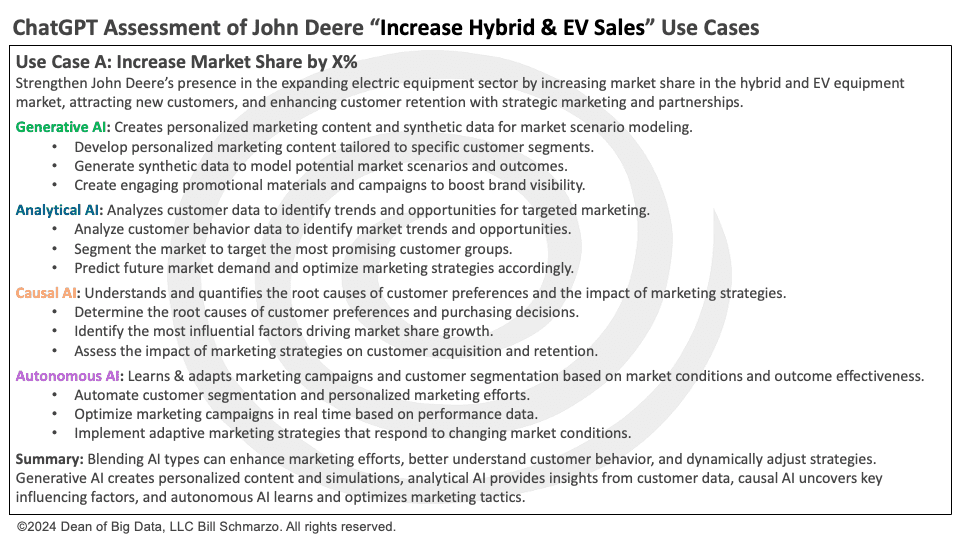
Figure 4: Potential Areas Where AI Can Power Prioritized Use Case
With this as a starting point, we are ready to advance to the following four TLADS steps (Step 5: Brainstorm Scores & Features, Step 6: Explore Analytic Algorithms, Step 7: Map Scores to Decision Recommendations, and Step 8: Create Learning-based UEX) that will help us align data science, data engineering, design thinking, and user experience efforts to deliver more relevant, meaningful, responsible, and ethical outcomes.
Summary
Aspirational AI combines various AI capabilities to tackle complex challenges effectively and ethically. Organizations can use the TLADS methodology and GenAI tools like ChatGPT to drive continuous learning and adaptation, addressing immediate problems and working towards a more sustainable, equitable, and innovative future.
[1] Special thanks to Michael Allen from Dell Technologies for exploring, ideating, and creating this GenAI prompt based on my blogs and my TLADS methodology.
It’s becoming clear that with all the brain and consciousness theories out there, the proof will be in the pudding. By this I mean, can any particular theory be used to create a human adult level conscious machine. My bet is on the late Gerald Edelman’s Extended Theory of Neuronal Group Selection. The lead group in robotics based on this theory is the Neurorobotics Lab at UC at Irvine. Dr. Edelman distinguished between primary consciousness, which came first in evolution, and that humans share with other conscious animals, and higher order consciousness, which came to only humans with the acquisition of language. A machine with only primary consciousness will probably have to come first.
What I find special about the TNGS is the Darwin series of automata created at the Neurosciences Institute by Dr. Edelman and his colleagues in the 1990’s and 2000’s. These machines perform in the real world, not in a restricted simulated world, and display convincing physical behavior indicative of higher psychological functions necessary for consciousness, such as perceptual categorization, memory, and learning. They are based on realistic models of the parts of the biological brain that the theory claims subserve these functions. The extended TNGS allows for the emergence of consciousness based only on further evolutionary development of the brain areas responsible for these functions, in a parsimonious way. No other research I’ve encountered is anywhere near as convincing.
I post because on almost every video and article about the brain and consciousness that I encounter, the attitude seems to be that we still know next to nothing about how the brain and consciousness work; that there’s lots of data but no unifying theory. I believe the extended TNGS is that theory. My motivation is to keep that theory in front of the public. And obviously, I consider it the route to a truly conscious machine, primary and higher-order.
My advice to people who want to create a conscious machine is to seriously ground themselves in the extended TNGS and the Darwin automata first, and proceed from there, by applying to Jeff Krichmar’s lab at UC Irvine, possibly. Dr. Edelman’s roadmap to a conscious machine is at https://arxiv.org/abs/2105.10461