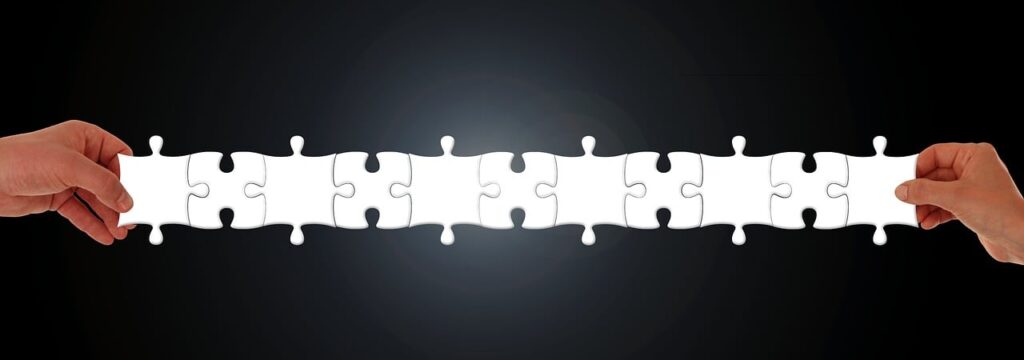
Image by Gerd Altmann from Pixabay
The considerable advantage of the internet and the web is that together they boosted the global economy dramatically. By 2020, internet and web technologies were contributing $2.45 trillion annually to US gross domestic product (GDP), according to a 2021 Interactive Advertising Bureau study. Globally, ecommerce in 2024 will handle $6.3 trillion of transactions, up from $5.8 in 2023, estimates Forbes Advisor.
The internet and the web, of course, were established using open protocols at universities and publicly funded scientific institutions, and much of early web activity centered around improvements to scientific research sharing. CERN, the European Organization for Nuclear Research where Tim Berners-Lee first invented the World Wide Web, the HTML markup language, the URL system, and HTTP, was an early European joint venture established on the border between France and Switzerland that now has 24 member countries.
The design of these now ubiquitous networking methods aligned closely with public scientific goals, opening the door for global scientific collaboration based on broad data sharing.
The global scientific context for open data sharing
In August 2024, Sean Hill, a psychology and physiology professor at the University of Toronto and formerly a co-director of the Blue Brain Project founded at the École Polytechnique Fédérale de Lausanne (EPFL) in Switzerland in 2005, posted on Medium an extensive review on “How Open Data and AI Can Help Solve the World’s Biggest Challenges”.
Hill was one of the principals behind Blue Brain Nexus, an open knowledge graph platform that simplified and allowed the scaling of heterogeneous, multimedia data sharing between research organizations focused on reverse engineering the brain. You can imagine in particular how important large and computationally complex imagery files, for example, are to research sharing when it comes to such engineering efforts.
Now Hill is co-founder of Senscience.ai, which offers an AI-enabled platform that “simplifies the curation and publishing of scientific data, ensuring it’s accessible, understandable, and reusable for everyone.”
Hill organized his Medium post on the world’s biggest challenges and how open data and AI can help around a United Nations list of 17 Sustainable Development Goals (SDGs):
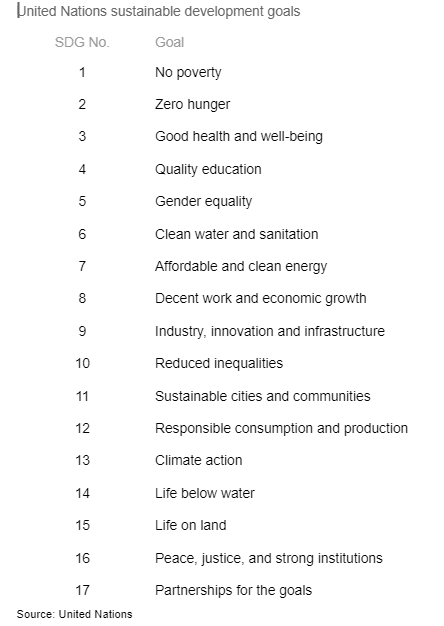
This UN goals list succinctly captures the nature and extent of the sustainability challenges humanity faces, and it’s striking when looking through the list how interdependent the goals are. Without effective partnership and collaboration (No. 1), how can we as a world scale any of the other efforts sufficiently to have a significant impact?
Without responsible consumption and production (No. 12), how can we slow the pace of climate change or restore individual habitats that are all ultimately themselves dependent on the health of the overall ecosystem?
It’s clear how important industry, innovation and infrastructure (No. 9) is to provide the means for the remaining goals on the list. But it’s also evident how unequally the benefits of industry, innovation and infrastructure have been distributed worldwide since the advent of the internet and the web.
The open data and AI challenge
The bottom line challenge for open data and AI over the coming decades that Hill underscores is one of making the most pertinent, actionable and accurate understanding relevant to these goals accessible. In other words, it’s an information, knowledge and wisdom collection, analysis, and distribution problem.
The classic data, information, knowledge and wisdom (DIKW) pyramid comes to mind. Semantic graph database management system provider Ontotext’s version of that pyramid highlights the additional value added the higher up you are in the pyramid:
The data, information, knowledge and wisdom (DIKW) pyramid
Source: Ontotext
How open data stewardship can boost enterprise AI’s near-term impact potential
Hill appropriately places a strong emphasis on data stewardship and best practices in data management in general. Sound data management practices and data maturity are synonymous.
Enterprises who’ve established open data programs can boost their data maturity by participating in scientific open data development and sharing efforts and learning best practices from a scientific community that is focused by necessity on high quality data.
“Data stewards,” he says, “reduce the burdens on scientists and ensure that data is of high quality and ready for future use.”
Knowledge graphs provide a key architectural means of scaling data quality and encourage an important mindset shift that helps tip the balance toward a truly AI-ready culture. Raising the profile of data stewardship by encouraging more businesspeople to participate in the overall data quality effort as domain experts and stewards will be essential to future success.