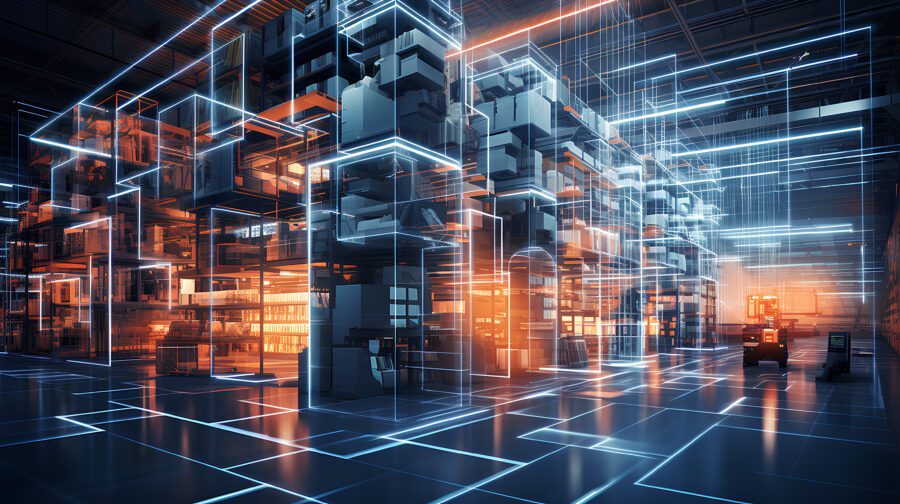
Organizations rely heavily on data warehouses and data lakes to store and manage vast information in today’s data-driven world. Maintaining high performance becomes a critical challenge as data warehouses grow in complexity and scale. One often overlooked aspect that significantly influences data warehouse performance is data governance. Effective data governance practices not only ensure data quality and compliance but also enhance a data warehouse’s overall performance. This article explores the impact of data governance on data warehouse performance. It highlights the key practices that can lead to optimal results.
Understanding data governance
Data governance is the policies, processes, and standards organizations implement to manage their data assets. It encompasses data quality management, security, lineage, and metadata management. The main aim of data governance is to ensure that data is accurate and secure throughout its journey.
Data quality management
High-quality data is crucial for a data warehouse’s performance. Data quality can lead to efficient queries, increased data processing time, and accurate analytics. Data governance frameworks include data quality management practices that involve data cleansing, validation, and standardization. By implementing these practices, organizations can ensure that only high-quality data enters the data warehouse, leading to faster query performance and more reliable insights.
Data lineage and metadata management
Data lineage refers to tracking the flow of data from its origin to its destination, including all the transformations it undergoes. Metadata management involves maintaining detailed information about the data, such as its source, structure, and usage. Both data lineage and metadata management are essential components of data governance.
In data warehouse architecture, a clear understanding of data lineage and comprehensive metadata can significantly enhance performance. When data lineage is well-documented, tracing and resolving any issues becomes easier, reducing downtime and improving query efficiency. Metadata management allows for better data organization and faster retrieval, as users can quickly identify and access the needed data.
Data security and compliance
Data security is a critical aspect of data governance. Implementing robust security measures protects sensitive data from unauthorized access and breaches. In addition to security, data governance also addresses compliance with regulatory requirements.
Integrating data security and compliance measures into the data warehouse architecture can positively impact performance. Secure and compliant data handling practices minimize the risk of data breaches, reducing the need for costly and time-consuming remediation efforts. Moreover, compliance with regulations ensures that data is managed consistently and standardized, enhancing overall data quality and reliability.
Streamlined data integration
Effective data governance facilitates streamlined data integration processes. With well-defined data governance policies, organizations can establish standardized procedures for data extraction, transformation, and loading (ETL). Standardized ETL processes reduce data redundancy, improve data consistency, and optimize data flow into the warehouse.
Streamlined data integration directly impacts a data warehouse’s performance. Organizations can achieve faster data processing times and more efficient resource utilization by reducing the complexity and variability of data integration tasks. This leads to improved query performance and a more responsive data warehouse environment.
Enhanced decision-making and analytics
Ultimately, the goal of a data warehouse is to support decision-making and analytics. Therefore, effective data governance ensures that the data used for these purposes is accurate, consistent, and trustworthy. Consequently, high-quality data leads to more reliable and insightful analytics, enabling organizations to make informed decisions confidently.
When data governance practices are in place, data users can trust their data, reducing the need for time-consuming data validation and reconciliation efforts. This not only improves the efficiency of analytical processes but also enhances the overall performance of the data warehouse.
To ensure effective data governance in data warehousing, organizations should follow several key steps:
- Establish a data governance framework: Develop a comprehensive framework that outlines data governance policies, roles, and responsibilities. This framework should include data quality, security, lineage, and metadata management guidelines.
- Appoint data stewards: Designate individuals or teams responsible for managing and overseeing data governance practices. Data stewards are crucial in maintaining data quality, ensuring compliance, and addressing data-related issues.
- Implement data quality tools: Utilize data quality tools to automate data cleansing, validation, and standardization processes. These tools help maintain high data quality and reduce the burden on data management teams.
- Develop data lineage and metadata repositories: Create centralized repositories for tracking data lineage and storing metadata. These repositories should be accessible to data users and regularly updated to reflect changes in data sources and transformations.
- Integrate security measures: Implement robust security measures, such as encryption, access controls, and monitoring, to protect sensitive data. Ensure that security practices comply with relevant regulations and industry standards.
- Standardize data integration processes: Establish standardized ETL processes to ensure consistent data integration. This includes defining data extraction, transformation, and loading procedures and setting data validation and reconciliation rules.
- Monitor and review data governance practices: Regularly monitor and review data governance practices to identify areas for improvement. Use key performance indicators (KPIs) to measure the effectiveness of data governance and make necessary adjustments.
By following these steps, organizations can ensure robust data governance in their data warehouse environments, which will improve performance, data quality, and overall efficiency.
Conclusion
Data governance is crucial in the performance of data warehouses. Firstly, by ensuring data quality, managing data lineage and metadata, and implementing robust security measures, data governance practices contribute to the optimal performance of data warehouses. Moreover, by streamlining data integration and enhancing decision-making and analytics, organizations that prioritize data governance in their data warehouse architecture will be better equipped to handle the challenges of growing data volumes and complexity. Consequently, they will ultimately achieve faster, more reliable, and insightful data analytics.