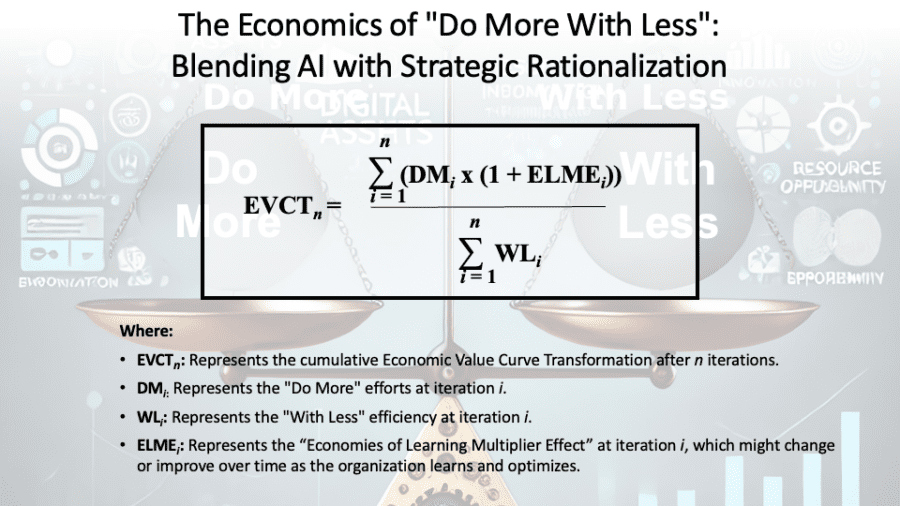
I hear it in nearly every customer conversation: “We must find a way to do more with less.” This modern business mantra reflects the economic realities of constrained resources and growing complexity, balanced against the strategic imperatives of efficiency, innovation, and sustainability. However, “Doing More With Less” isn’t merely about cost-cutting; it’s a strategic approach for achieving sustainable, long-term success. The “Do More with Less” equation has two key components:
- “Do More” focuses on leveraging AI to unlock new sources of economic value—whether from customers, products, services, operations, or even societal impacts—by reusing and continuously enhancing data and analytic assets. By adopting modern digital strategies, organizations can repurpose these digital assets to generate new business opportunities and drive innovation.
- “With Less” emphasizes the need for organizational discipline, prioritization, and empowered decision-making—traits often found in the most successful sports teams. It requires agile and adaptive teams capable of swift responses to changing market dynamics and emerging opportunities, engaging in what I call “Organizational Improvisation” or “Organizational Improv” to fine-tune operations and maximize value creation, even with limited resources.
Let’s dive into both sides of the equation, but first, a warning.
The Paving the Cowpath Dilemma
The “paving the cowpath dilemma” occurs when organizations adopt new technologies to automate existing processes without reimagining them to fully utilize the new capabilities. Rather than innovating to develop more efficient, streamlined, or value-driven workflows that capitalize on digital advancements, they replicate outdated and inefficient processes. For example:
- Some retailers have implemented AI to optimize inventory management by using historical sales data to predict stock levels. However, they rely on outdated patterns and assumptions instead of reengineering the inventory process to dynamically respond to real-time data such as market trends, social media signals, or local events. This approach merely automates the old, flawed process of restocking based on past trends without leveraging AI’s potential to dynamically adapt and improve decision-making, often resulting in overstock or stockouts.
- Some financial institutions have deployed AI models to automate loan approvals based on their existing credit scoring methods. These models use historical loan data and traditional credit scores as their primary input, replicating the same biases and limitations in human decision-making. Instead of reimagining the loan approval process or using AI to provide more inclusive financial services, the AI automates the conventional, biased decision paths, potentially excluding qualified applicants and perpetuating inequities in lending practices.
- Some manufacturing companies have implemented AI-driven predictive maintenance systems to anticipate equipment failures. However, instead of reimagining their maintenance strategy to optimize overall equipment efficiency and integrate cross-departmental insights, they replicate their existing reactive maintenance processes, which means they rely on outdated maintenance schedules or fail to coordinate across teams, missing out on broader opportunities for reducing downtime and maximizing throughput.
Now that we understand the “Paving the cowpath dilemma,” let’s dive into the different sides of the “Do more with less” equation.
#1: The Economic Drivers of “Do More”
The concept of “doing more” has traditionally focused on increasing productivity—the belief that we can accomplish more tasks in less time. While boosting productivity is beneficial, the greater goal should be to enhance effectiveness—not just doing things faster but better. There are several modern data economic concepts that we can leverage to enable the “Do More” side of the equation, including (Figure 1):
- Data Economic Multiplier Effect describes the exponential increase in economic value as data is reused and repurposed across multiple applications, decisions, and business processes. This multiplier effect enables organizations to derive compound benefits from their data investments, creating new opportunities for revenue growth, cost reduction, and innovation. For example, a logistics company could use IoT data from delivery trucks to optimize routes, reducing fuel costs. This data could then be utilized for predictive maintenance to prevent breakdowns, further lowering operational costs and increasing the overall value derived from a single data source.
- Marginal Propensity to Reuse (MPR) measures the likelihood that a data asset, algorithm, or analytic model will be reused in different contexts or applications, enhancing its economic value. For example, consider a healthcare organization that develops an AI model to detect early signs of diabetes based on patient data like age, weight, glucose levels, and lifestyle factors. The same model could also be reused to create a personalized treatment recommendation system, integrating insights from localized treatment outcomes to optimize patient care paths.
- Nanoeconomics is the economic theory of individual entity (human or device) predicted behavioral and performance propensities. For example, President Obama’s election team gathered extensive data on voters’ demographic details, social media activities, consumer behavior, and past voting patterns. By applying nanoeconomics, the campaign identified specific issues that resonated with each voter. It then tailored its outreach efforts to target these individuals with highly personalized communications, ultimately influencing their voting behavior and maximizing voter turnout.
- The Economic Digital Asset Valuation Theorem provides a framework for assessing the value of digital assets, such as data, by evaluating their potential to generate future economic benefits. For example, a financial institution could quantify the value of its customer transaction data by estimating its impact on cross-selling and upselling opportunities and its potential to identify fraud and reduce credit risk, thus quantifying the data’s contribution to the institution’s overall economic value.
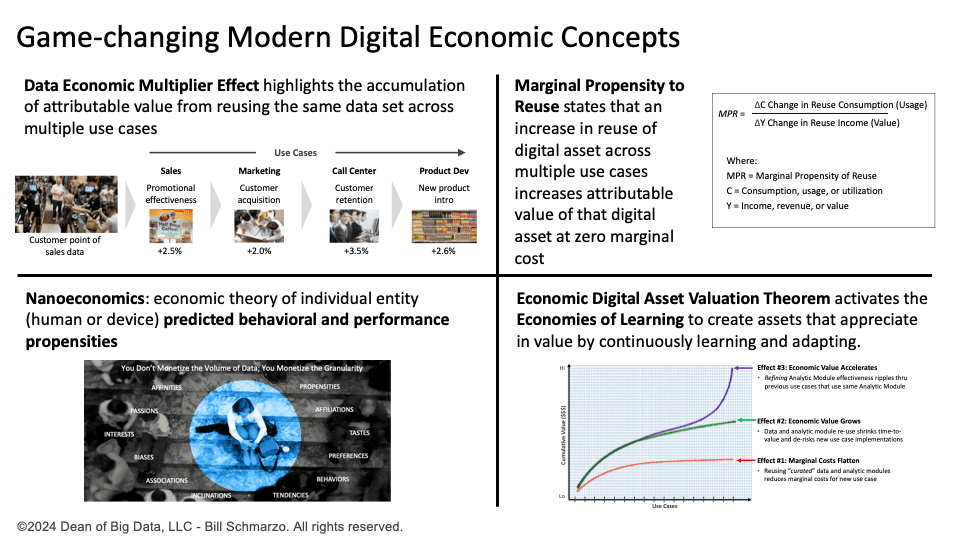
Figure 1: Game-changing Modern Digital (Data) Economic Concepts
#2: The Organizational Discipline of “With Less”
Modern data and AI economic concepts can drive organizational effectiveness, which is essential for achieving more with fewer resources. However, achieving ‘more with less’ is not easy. It requires:
- Prioritization and focus involve determining which projects, initiatives, and tasks align most closely with the organization’s strategic goals and provide the highest value. Organizations can maximize their impact and effectiveness with limited resources by concentrating on high-impact activities and eliminating or deferring lower-value efforts. This requires a disciplined approach to decision-making and the ability to say “no” to non-critical activities.
- Rationalization involves critically evaluating existing products, services, and processes to identify redundancies, inefficiencies, or underperforming areas. The goal is to streamline operations by eliminating unnecessary complexity and focusing on offerings that deliver the most value to customers and the business. This process often includes reducing the product portfolio, optimizing processes, and reallocating resources from low-value activities to those that drive higher returns.
- Strategic resource allocation involves deploying resources—such as capital, talent, and time—where they will deliver the most significant value and impact. It involves understanding different initiatives’ relative return on investment (ROI) and dynamically reallocating resources in response to changing conditions, opportunities, or risks.
These examples illustrate how organizations can apply the “With Less” principle to achieve more effective and efficient outcomes by being intentional about where they focus their efforts, simplifying what they offer and how they operate, and strategically directing their resources. It requires creating the ultimate organizational capabilities and agility—a term I can call organizational improvisation or “organizational improv[1]”.
“Do More With Less” Mathematical Equation
Is there a way to represent this “Do More With Less” as a mathematical equation so that one can better understand how the different components of this formula can become a force multiplier in transforming an organization’s economic value curve?
So, for fun, I decided to give it a try. The formula below might be flawed, but I hope it can serve as a starting point for us to discuss, question, and enhance (Figure 2).
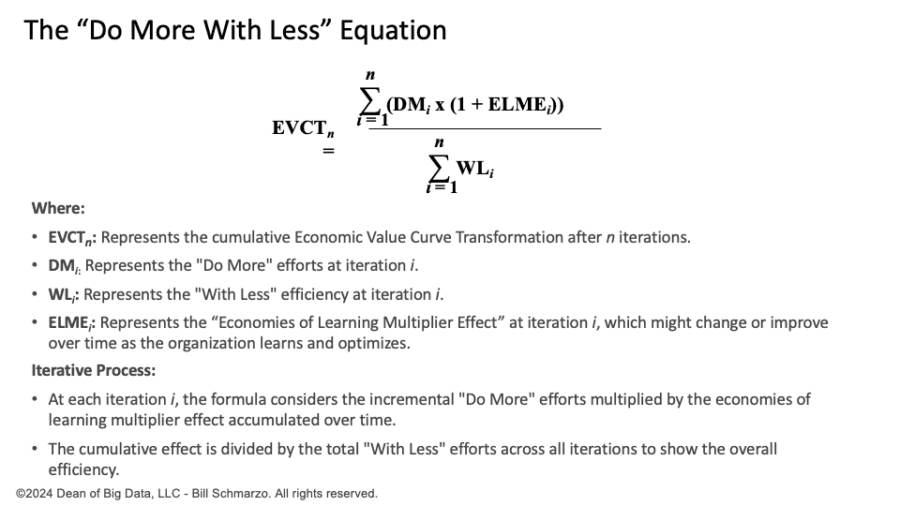
Figure 2: The “Do More With Less” Equation
I would love your thoughts on how to improve this formula.
The Economics of “Do More With Less” Conclusion
The ultimate objective for any organization is to transform its Economic Value Curve[2] by effectively doing more with less. Combining “Do More”—leveraging data and analytic-driven economic strategies—with “With Less”—emphasizing disciplined organizational focus, prioritization, and rationalization—creates a powerful catalyst for business growth and operational excellence.
The critical question is: How can these principles be applied within their organizations to drive sustainable economic growth and competitive advantage?
[1] “Organizational improv” refers to the ability of teams and individuals to adapt quickly and creatively to unexpected changes. It yields flexible teams that maintain operational integrity while adjusting to evolving situations.
[2] The Economic Value Curve defines the relationship between independent variables and their influence on a dependent outcome variable, illustrating how different factors impact overall economic value.