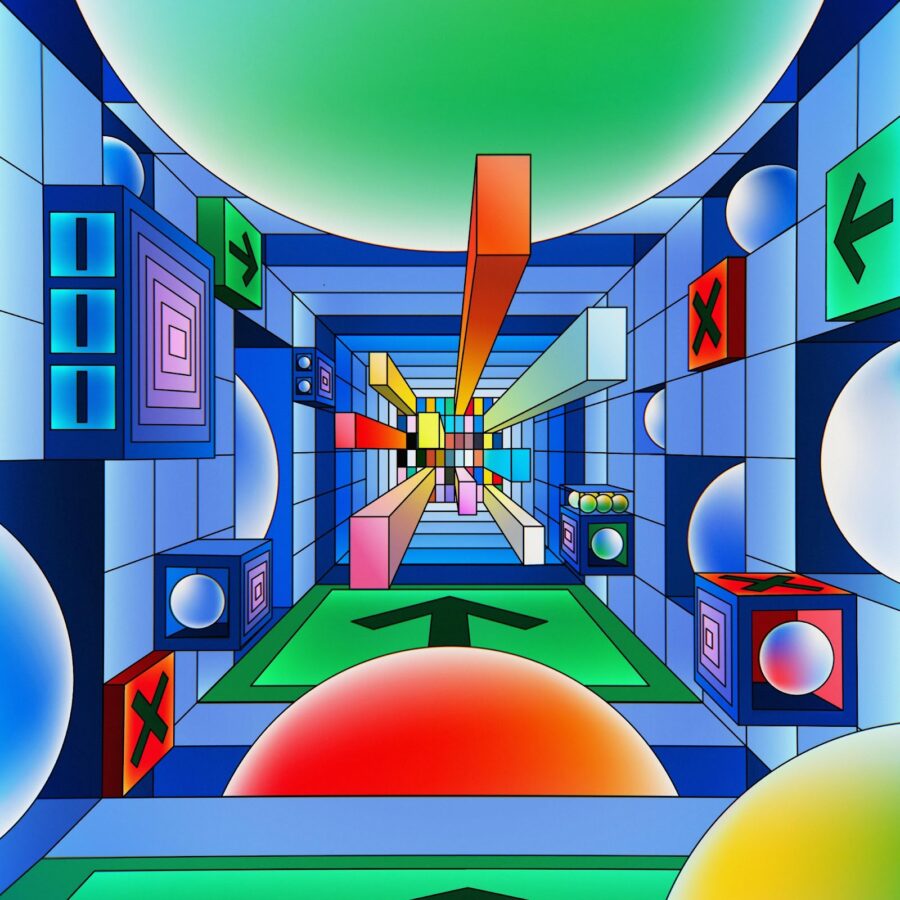
Image credit: Pexels.com
Artificial intelligence burst onto the scene, oozing with promise. Bold predictions foretold of pending transformations across industries. But after the initial enthusiasm, pragmatism set in. Delivering impactful AI innovations remains an arduous endeavor. However, vast opportunities still beckon for those committed to unlocking AI’s potential through relentless experimentation.
This experimental pursuit demands consistently questioning assumptions and taking risks. AI’s future promises will not simply emerge from tweaking current approaches and tools. Rather, we must reimagine solutions by combining creative curiosity with rigorous research.
By examining key frontiers and practices of experimentation, businesses can gain valuable perspectives on navigating AI’s long road of discoveries still ahead. Let’s explore this winding but rewarding pathway.
Cultivating the experimentation mindset
Trailblazing AI solutions rarely follow straight narrow paths. Impactful innovations emerge through winding journeys of failures, insights, and eventual illumination. Experimentation compels us to question the status quo and explore uncharted territories.
Every assumption and norm must be scrutinized, no matter how ingrained. For example, autonomous vehicles will require a radical rethinking of transportation, not just teaching cars to incrementally become more human-like. Breakthroughs come from questioning fundamentals.
Of course, curiosity alone is not enough. Experimentation methodology still matters. Forming hypotheses, collecting data, and quantifying results provides rigor. But room must also exist for testing unconventional ideas without yet knowing what’s possible. Scientific reasoning and creative openness together beget impact.
Organizations must nurture these experimental mindsets. Innovation stems from cultures that encourage exploration, learning, and reasonable risk-taking without fear of failure. Leadership should motivate teams to constantly ask “what if” about reimagining the status quo.
Regardless of industry, AI’s impact on businesses is growing and accelerating. According to a recent study, in 2021, 19% of companies surveyed used more than 30% of their tech budgets for AI projects.
By 2024, 49% of organizations allocate over 30% of their tech budget toward AI. These organizations generated ROI up to 20 percentage points higher than others. In such environments, AI innovation flourishes.
Key areas to experiment with
The expansive scope of artificial intelligence offers manifold possibilities for trailblazing experiments. Nearly every aspect of developing AI solutions warrants questioning assumptions and trying alternate approaches. However, three areas in particular merit emphasis.
Data: Fuel for AI innovation
Data is the raw material powering nearly all AI. Most experts echo the refrain that better data begets better models. But what constitutes “better” must be reexamined.
Rarely will an organization’s current data assets contain optimal training data as-is. Thus, sourcing and curating innovative datasets becomes pivotal. Start by scrutinizing internal data gaps and biases. Fixing these can already boost model performance.
Additionally, explore entirely novel open datasets to combine with internal data. Even seemingly irrelevant data can provide value in unexpected ways. For example, satellite images have improved self-driving vehicle navigational AI by modeling rarely traversed edge cases.
Always validate data by the models it produces rather than proxy metrics alone. Let real-world evaluation guide iterations. With enough exploration, transformative data generation approaches can emerge.
Models: Architecting AI’s intelligence
The machine learning models comprising AI’s intelligence also warrant ample experimentation. Rather than just comparing benchmarks, purposefully try more unique models.
Start by tweaking established model families like CNNs and LSTMs before building entirely new architectures. Creatively alter model complexities and connections to capture specialized intricacies. Combine complementary model types like computer vision and language models for multimedia capabilities.
When constructing novel models, begin simply, validate behaviors, then increase sophistication. Masterfully experimenting with model architectures and learning can unlock game-changing innovations.
In 2021, startup Anthropic built Claude, an AI assistant focused on safety. Its Constitutional AI technique teaches models to make decisions based on user preferences. Constitutional AI promises to reduce harm through fundamentally different architecture. Such creative modeling catalyzes new possibilities.
Deployment: Path to real-world impact
Even the best-performing models mean little without real-world deployment. This last mile uncovers obstacles not seen in controlled environments. Thus, rigorously experimenting with operationalization becomes critical.
Robust profile across expected platforms, especially resource-constrained edge devices. Try various compression tools to optimize. Measure speed, latency, and stability via live testing. Anticipate chaotic user behaviors, too, by running open beta explorations. Fix issues through rapid iteration.
For example, Google software subsidiary Intrinsic rethinks factory robots by experimenting with model simulation and testing. Their platform optimizes models to safely operate actual robots in working factories under dynamic conditions. Such deployment-focused experimentation creates differentiated value.
The iterative journey of experimentation
Charting new AI innovation frontiers is not a straight path but a winding road filled with twists, turns, and surprises. Testing original ideas involves repeating cycles of hypothesis formulation, experiment execution, analysis, and knowledge compounding.
Each cycle expands understanding – negative results still provide wisdom. Continued revolutions yield increasingly refined discoveries, steadily unlocking unprecedented capabilities. Like compounding interest, the momentum builds with each iteration, ultimately achieving breakthrough trajectories.
Of course, dead-ends and detours should be expected on this nonlinear innovation journey. Objective examination after each experiment is imperative to determine the appropriate next actions:
- Iterate experiments when the original hypotheses still demonstrate merit. Refine aspects for greater rigor or insights.
- Pivot alternative hypotheses that show more initial promise based on findings.
- Occasionally restart entirely when fundamental theories prove flawed despite multiple iterations.
Tracking peripheral insights gained, though not yet directly applicable, can inform future work. Scientific discoveries rarely materialize instantly but rather accumulate through compounding knowledge. Thoughtfully navigating the long road of iterations sustains progress closer to the desired innovation.
With persistence through failures combined with scientific rigor and patience, the winding path of experimentation ultimately unlocks breakthrough discoveries over time. Each piece of new knowledge compounds, gradually producing world-changing AI innovations.
Unlocking unique advantages
Investing in bold experimentation promises more than intellectual satisfaction. Market pressures demand AI deliver tangible competitive advantages – enabling key functions to be better, faster, or cheaper.
However, research shows that only 12% of companies use AI to decisively outpace competitors. Accenture found this select group, dubbed AI Achievers, generates 50% higher revenue growth by leveraging AI for strategic differentiation.
The key to joining the AI Achievers lies in pioneering customized solutions tailored to unmet market needs. Specialized datasets surpass generic benchmarks in capturing niche intricacies. Novel model architectures excel at specialized tasks compared to one-size-fits-all solutions. Optimized deployments maximize performance despite operational constraints.
Together, these differentiated capabilities directly translate into measurable business impact:
- Enhanced products and services to improve customer experiences
- Increased productivity by automating higher-value tasks faster
- Lower costs through efficient data and model optimization
- New market expansions by solving previously impossible challenges
- Sustained competitive advantage by continuously pushing boundaries
In essence, pioneering AI innovations satisfy unmet niche needs to carve out defendable market positions difficult for fast followers to duplicate. The compounding knowledge gained from relentless experimentation manifests into enduring strategic value.
The endless pursuit of experimentation
True AI breakthroughs come from compound knowledge gained through endless pursuit of experimentation. Initial successes demonstrate new puzzles warranting further examination. Each turn of the cycle expands understanding in pursuit of immense possibilities still undiscovered.
But this journey demands a commitment to lifelong learning. No tool, technique, or paradigm will satisfy diverse needs across domains. New challenges will continually arise, requiring rethinking assumptions. Thus, consistent questioning, creating, and researching remain imperative to advancing AI’s state-of-the-art.
The future promises of artificial intelligence await discovery by intentional experimenters willing to navigate uncharted frontiers. Creative research efforts can help unlock innovation potentials beyond what we conceive today. We inch closer to achieving AI’s grand ambitions by persistently pushing boundaries.