
It’s been ten years since Google (now a child of holding company Alphabet) coined the term “knowledge graph” and described (in general terms) how their knowledge graph worked.
And it’s been over 20 years since Tim Berners-Lee, James Hendler and Ora Lassila published their first article to describe the semantic web they envisioned. Many knowledge graphs have been built using the semantic standards the W3C subsequently put in motion a decade or more ago.
It’s interesting to ponder what’s happened since. Over the past decade, Alphabet has grown consistently to become one of the top six companies globally to achieve a market capitalization (total stock value of shares outstanding) of over $1 trillion.
Here’s how Alphabet ranked relative to its closest peers in market cap terms early in 2022:
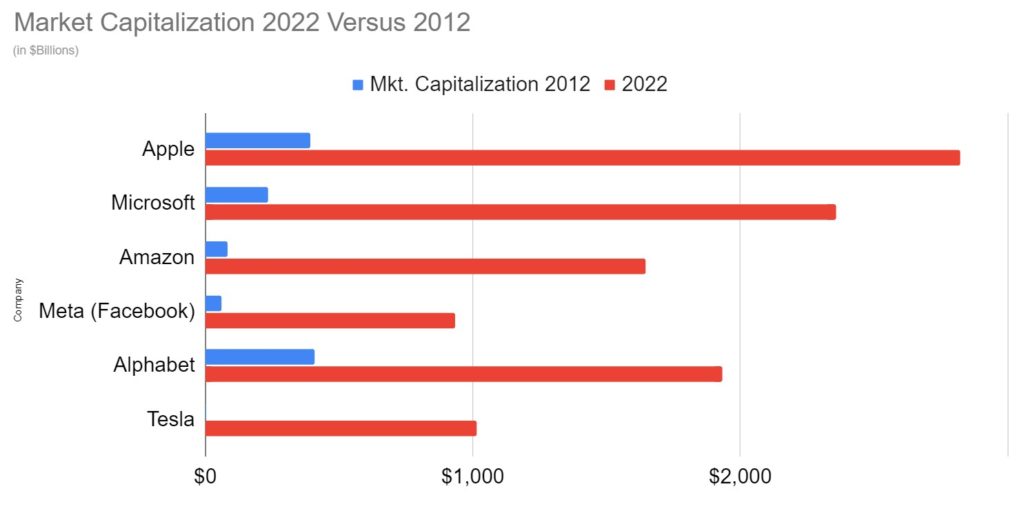
Y-Charts and Macro Trends, 2021 and 2022
What these market cap leaders have been doing with knowledge graphs
I did some desk research on which of the companies listed has invested in knowledge graph development over the past decade:
- Apple acquired Siri, a spinoff from a Stanford Research Institute project, in 2010. Tom Gruber co-founded the SRI team. Gruber, a pioneer in humanistic or human-in-the-loop AI had published on ontology design for knowledge sharing in 1993 at SRI. More recently in 2019, an Apple job rec described its knowledge graph engineering team work as follows:
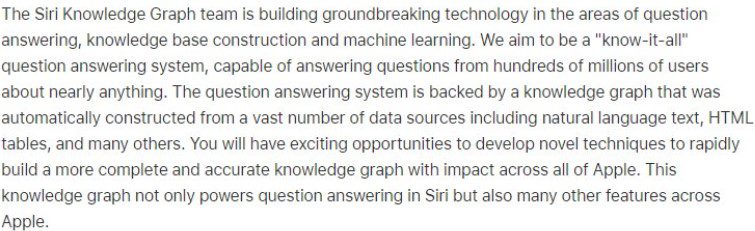
(Courtesy of Aaron Bradley via Twitter, January 19, 2019)
- Microsoft Research Asia developed the Trinity distributed graph store in 2010 and published its in memory RDF graph capabilities in a 2013 paper. Satori for a number of years has been Microsoft’s version of a knowledge/fact graph akin to Google Knowledge Graph. Microsoft Academic Knowledge Graph (MAKG) appeared around 2019. An open 2022 job req for a Data & Analytics Scientist for the company mentions driving “innovations in Business Knowledge Graph models and algorithms” as a requirement of the position.
- Amazon for years seemed to be hesitant about hiring ontologists, but in the late 2010s embarked on a product knowledge graph. The Alexa conversational assistant has a knowledge graph foundation, and Alexa’s Meaning Representation Language is graph based, with a paper published on the topic in 2016. Team Alquist from Czech Technical University won the fourth annual Alexa Grand Challenge Prize for a conversational knowledge graph that was the foundation for the Alquist 3.0 intelligent assistant in 2020.
- Meta (Facebook) announced Facebook Graph in March 2013, and by June 2013 had made it available to all US English users of Facebook. The Graph contained information on a billion different Facebook users at the time. Graph continues to be available in 2022.
- Alphabet (Google) announced its Knowledge Graph in 2012 and continues to have ontologists and data architects with semantic standards experience on staff.
Tesla–I found no evidence (yet) that Tesla’s using a knowledge graph.
Google and the early days of its Knowledge Graph
I still remember the illustrations Google used to describe its Knowledge Graph. One illustration showed a directed graph with named entities (Da Vinci, the Mona Lisa, and the Louvre) and named relationships showing how one entity related to another.
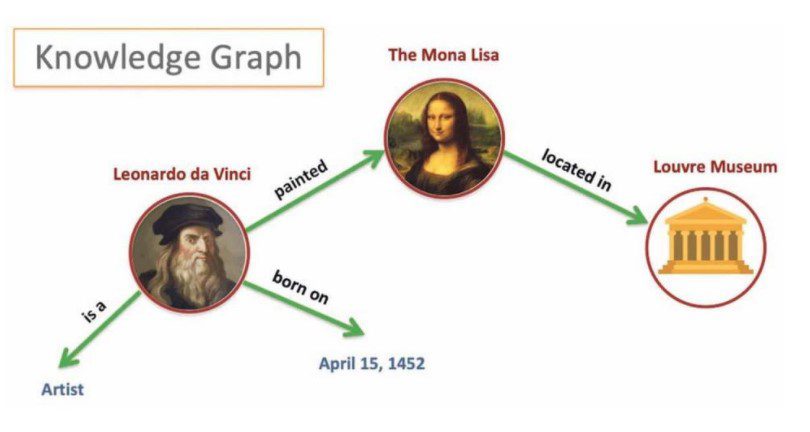
This illustration exemplified the pieces of context that could be developed and connected Tinker Toy style to another compatible semantic graph, a much simpler, more scalable and deeply integrated process than linking relational tables. To reduce the number of clicks to get to the information, Google then used some of the Da Vinci facts chosen to populate its knowledge panels, which appeared directly on the results page. By 2016, a third of Google’s 100 billion monthly search results came with a knowledge panel.
Soon, if all you were looking up seemed to be a simple fact, the Google search results page would often display a fact directly on the page that the search engine and its algorithm took a stab at determining was the fact you were most interested in retrieving.
Knowledge graphs in the 2020s
Fast forward to 2020. Gartner then in its Hype Cycle for AI plotted knowledge graphs near the apex of the Peak of Inflated Expectations.
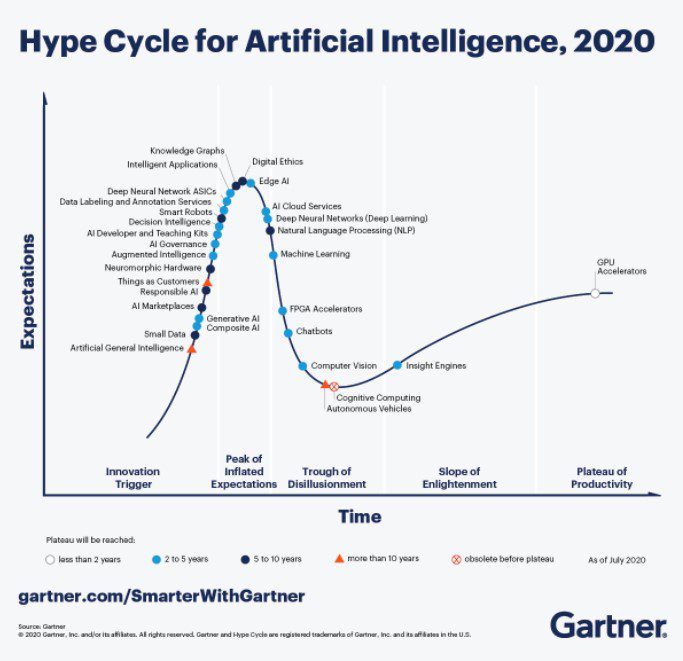
Only two years earlier, in 2018, Gartner first declared knowledge graphs to be an emerging technology, It took 26 years since 1996’s Resource Description Framework (a graph data model that cleared the way for standard subject-verb-object semantics) was introduced for the hype around semantic integration in the form of knowledge graphs to peak.
It’s worth noting, of course, that the theory behind neural networks goes back to 1873, and a specific focus of research on neural nets in AI began in 1943. But it took until the 1990s for compute, networking and storage to progress sufficiently for neural nets to gain a foothold. A number of AI pundits have observed that the neural net algorithms today bear more than a passing resemblance to the algorithms developed when “AI” was just getting started.
Slowly evolving technologies can be the most impactful
A number of other enterprises have made significant strides with their knowledge graph implementations. Between February 28th and March 1st, Semantic Arts hosted its first Enterprise Data Transformation & Knowledge Graph Adoption virtual event. Some of the speakers featured included these:
- Parsa Mirhaji, Montefiore Hospitals
- Thomas Hubauer, Siemens
- Ben Gardner and Colin Wood, AstraZeneca
- Katarinna Kari, IKEA
- Peter Hutzli, The Swatch Group
It’s too early to say that knowledge graphs have gone mainstream, but it’s clear that more and more of the Fortune Global 1000 companies are adopting the technology, for many different use cases.
We keep making slow and steady progress toward scalable, human-in-the-loop, contextual computing. Stay tuned.