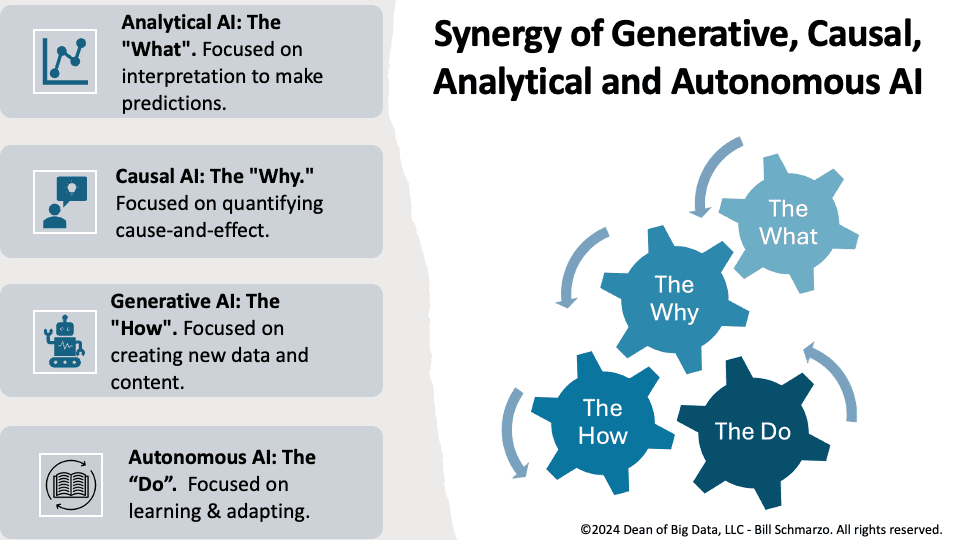
The current fascination with Generative AI (GenAI) – especially as manifested by OpenAI’s ChatGPT – has raised public awareness of Artificial Intelligence (AI) and its ability to create new sources of customer, product, service, and operational value. Leveraging GenAI tools and Large Language Models (LLMs) to generate new textual, graphical, video, and audio content is astounding.
However, let’s not forget about the predictive, understandable, and continuously learning legs of AI – analytical AI, which focuses on pattern recognition and prediction; causal AI, which seeks to identify and understand cause-and-effect relationships; and autonomous AI, which aims to operate independently and make real-time decisions. In the ever-evolving landscape of artificial intelligence (AI), four distinct but equally transformative branches have emerged: Generative AI, Analytical AI, Causal AI, and Autonomous AI.
As organizations strive to harness the power of data to drive decision-making and innovation, understanding the differences, similarities, and collaborative potential between these types of AI is crucial. This blog explores these facets, highlighting how combining Generative, Analytical, Causal, and Autonomous AI can unlock unprecedented economic value and create new opportunities for customer, product, service, and operational advancements (Figure 1).
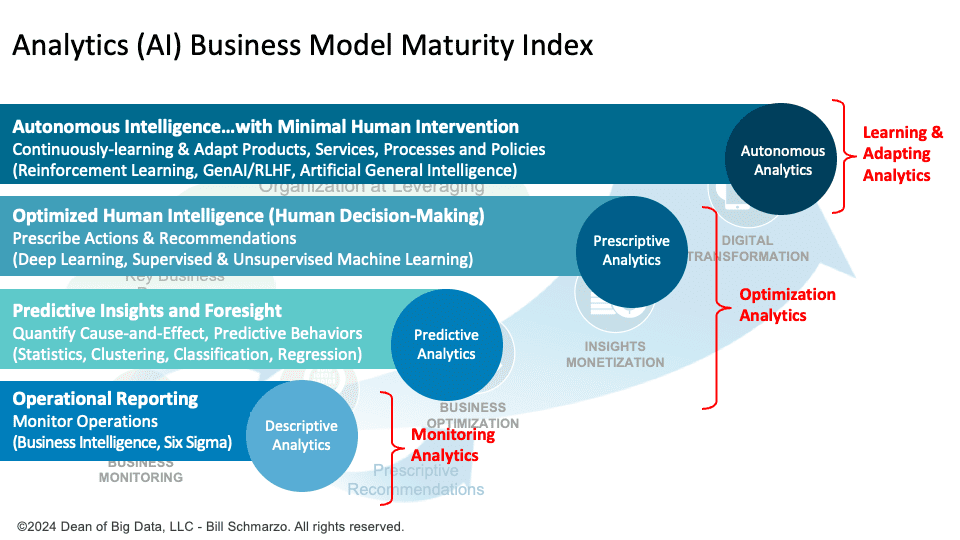
Figure 1: Analytics (AI) Business Model Maturity Index
Defining Generative, Analytical, Causal, and Autonomous AI
As always, let’s start by establishing some definitions:
- Generative AI: The “How.” Focused on creation, Generative AI builds upon patterns found in text and other structured data sets to generate new content, offering innovative ways to solve problems or create products. Applications include content generation, virtual environment design, and synthetic data creation for training models. It utilizes techniques like VAEs and GANs to generate realistic outputs. It is excellent for innovation and exploring new possibilities, scenarios, and solutions.
- Analytical AI: The “What?” Focused on interpretation, Analytical AI analyzes existing data to uncover patterns and trends, making predictions and recommendations. Applications include business intelligence, market analysis, customer behavior analysis, and predictive maintenance. It employs methods such as regression analysis, clustering, and classification. It is great for providing actionable insights and making data-driven decisions.
- Causal AI: The “Why.” Focused on understanding, Causal AI uncovers and quantifies cause-and-effect relationships to provide insights into the underlying reasons behind observed patterns and phenomena. This has applications in healthcare treatment effectiveness, economic policy formulation, and impact assessment of social interventions. Causal AI uses structural equation modeling, Bayesian networks, Granger causality, and multi-regression analysis methods. It is essential for making informed decisions based on understanding the true impact of variables and avoiding mistaking correlation for causation, which can lead to potential risks when making predictions based on non-causal data. (Check out “Causal Artificial Intelligence: The Next Step in Effective Business AI” by Judith Hurwitz and John Thompson to learn more about Causal AI.)
- Autonomous AI: The “Do.” Focused on independent operation and continuous learning, Autonomous AI systems are designed to perform tasks and make real-time decisions without human intervention. These systems integrate perception, decision-making, and action capabilities. Applications include autonomous vehicles, robotics, and intelligent agents. Techniques involve reinforcement learning, deep learning, and sensor fusion. Autonomous AI excels in environments requiring rapid, continuous decision-making and adaptability.
As I wrote in an earlier blog titled “Generative AI: Precursor to Autonomous Analytics,” Generative AI is a foundational technology leading toward developing Autonomous AI. Generative AI, with its ability to create new data and content based on existing patterns, paves the way for more sophisticated autonomous systems. These systems leverage the generative capabilities to enhance their decision-making processes, operate independently, and adapt to dynamic environments. This progression underscores the importance of understanding the interplay between these AI types to fully harness their combined potential in driving innovation and efficiency across various sectors.
Comparing Generative, Analytical, Causal, and Autonomous AI
Let’s create a quick matrix that compares critical aspects of these four different classifications of AI (Table 1).
Aspect | Generative AI (The How) | Analytical AI (The What) | Causal AI (The Why) | Autonomous AI (The Do) |
Purpose and Function | Focused on creation. Builds upon patterns found in massive data sets to generate new content, offering innovative ways to solve problems or create products. | Focused on interpretation. Analyzes existing data to uncover patterns and trends, making predictions and recommendations. | Focused on understanding. Uncovers cause-and-effect relationships, providing insights into the underlying reasons behind observed patterns and phenomena. | Focused on operations. Makes real-time decisions autonomously, integrating perception, decision-making, and action capabilities. |
Techniques and Algorithms | Utilizes VAEs and GANs to generate new data. These models are adept at generating high-quality, realistic outputs by learning the distributions of training data. | Employs regression analysis, clustering, classification, CNNs, RNNs, SVMs, and market basket analysis for pattern recognition and prediction. | Utilizes structural equation modeling, multi-variable regression analysis, and Bayesian networks to identify and validate causal relationships. | Utilizes reinforcement learning, deep learning, and sensor fusion to learn from interactions with their environment and make decisions in real-time. |
Applications | Used extensively in creative fields and for generating synthetic content. Examples include creating marketing content, designing virtual environments, and synthesizing realistic training data for machine learning models. | Applied in business intelligence, market analysis, customer behavior analysis, and operational optimizations. | Crucial in fields where understanding causality is critical, such as healthcare, economics, and social sciences. Helps in developing treatment plans, formulating economic policies, and assessing the impact of social interventions. | Applied in autonomous vehicles, robotics, and intelligent agents. Examples include self-driving cars, robotic process automation (RPA), and drone navigation. |
Strengths | Excellent for innovation, content creation, and scenarios where new data generation is required. | Great for predictive analytics, identifying patterns, and making data-driven decisions. | Essential for understanding the impact of interventions, ensuring decisions are based on sound causality rather than mere correlation. | Excels in environments requiring rapid, continuous decision-making and adaptability. Capable of performing complex tasks without human intervention. |
Challenges and Risks | Risk of generating biased or unrealistic data if not properly managed. Potential misuse for tasks requiring predictive accuracy without causal understanding. | Can mislead if correlations are interpreted as causations. May not explain why patterns exist, only that they do. | Requires robust data and methodologies to accurately identify causality. Misidentifying causal relationships can lead to incorrect conclusions and ineffective interventions. | High dependency on robust and comprehensive training data. Safety and ethical considerations are paramount. Potential for unexpected behavior in complex, dynamic environments. |
Table 1: Four Types of Artificial Intelligence (AI)
Industry Examples: Synergizing Generative, Analytical, Causal, and Autonomous AI
The synergy of Generative AI, Analytical AI, Causal AI, and Autonomous AI can profoundly impact every industry. Here are just a few examples (Figure 2):
- Healthcare—Personalized Treatment Plans. Generative AI creates personalized treatment protocols based on genetic information. Analytical AI predicts patient responses to these treatments based on historical data. Causal AI refines these plans by identifying the most effective treatment components. Autonomous AI administers treatment, monitors patient vitals, and makes real-time adjustments as necessary.
- Finance – Fraud Detection and Prevention. Generative AI generates fraud scenarios. Analytical AI detects unusual transaction patterns indicative of fraud. Causal AI pinpoints the underlying causes of these patterns. Autonomous AI implements real-time fraud prevention measures, monitors effectiveness, and learns and adapts as necessary.
- Manufacturing – Predictive Maintenance. Generative AI creates synthetic data for rare failure modes. Analytical AI predicts potential equipment failures based on sensor data. Causal AI determines the causes of these failures. Autonomous AI autonomously conducts and adjusts maintenance plans and procedures based on real-time learning.
- Retail—Personalized Marketing Campaigns. Generative AI develops personalized email campaigns. Analytical AI predicts which customers are likely to respond positively. Causal AI identifies the marketing elements that drive engagement. Autonomous AI assesses the effectiveness and adjusts marketing strategies in real time based on customer interactions and marketing outcomes.
- Supply Chain Management—Demand Forecasting and Inventory Management. Generative AI simulates potential changes in demand. Analytical AI forecasts future demand based on current trends. Causal AI determines the underlying causes of demand fluctuations. Autonomous AI adjusts inventory levels and supply chain logistics based on operational results in real-time.
- Education—Adaptive Learning Systems. Generative AI develops personalized educational content. Analytical AI analyzes and reports student performance. Causal AI evaluates the effectiveness of different teaching methods on individual students. Autonomous AI adapts the student learning experience in real time based on performance and behavioral feedback.
- Energy—Smart Grid Optimization. Generative AI generates and models different energy consumption scenarios. Analytical AI predicts energy demand. Causal AI identifies the causal relationships affecting energy consumption to optimize grid performance. Autonomous AI monitors and adjusts energy distribution autonomously, optimizing efficiency in real time.
- Agriculture—Precision Farming. Generative AI creates optimal crop planting and harvesting scenarios. Analytical AI analyzes soil, weather, and crop yield data to predict ideal planting and harvesting plans. Causal AI identifies the specific variables that drive ideal farming practices. Autonomous AI autonomously operates farming planning, scheduling, and equipment utilization, adjusting schedules based on real-time data.
Figure 2: Industry Use Cases: Synergizing Generative, Analytical, Causal, and Autonomous AI
These use cases demonstrate how integrating Generative AI, Analytical AI, Causal AI, and Autonomous AI can drive innovation, efficiency, and effectiveness across various industries, leveraging the strengths of each AI type to create significant value.
Synergizing Generative, Analytical, Causal, and Autonomous AI Summary
To fully realize the benefits of AI technologies, organizations must understand and capitalize on the distinct capabilities of Generative AI, Analytical AI, Causal AI, and Autonomous AI. By synergizing across these different types of AI, organizations can drive innovation, elevate decision-making processes, and optimize operational efficiency. The collective potential of these AI technologies emphasizes the transformative influence of AI in developing advanced, adaptable, and streamlined systems.
The synergy of generative, analytical, causal, and autonomous AI is a powerful combination! 🤖💡 It combines creativity, decision-making, causality understanding, and automation to tackle complex problems in innovative ways. 🧠✨ Fascinating stuff!
It’s incredible how Generative AI, particularly ChatGPT, is transforming the way we create content across various mediums. The potential to generate innovative customer experiences, products, and services is enormous. As LLMs continue to evolve, we’re only scratching the surface of what can be achieved. It’s an exciting time for AI, and its impact on industries and creativity will continue to grow in ways we haven’t yet imagined!
Absolutely insightful post, Bill! The comprehensive breakdown of the four branches of AI highlights the intricate interplay between Generative, Analytical, Causal, and Autonomous AI. Your emphasis on their combined potential to drive innovation and operational excellence is spot on. It’s fascinating to see how these technologies can work together to unlock new opportunities across various industries. Thank you for sharing these valuable insights!