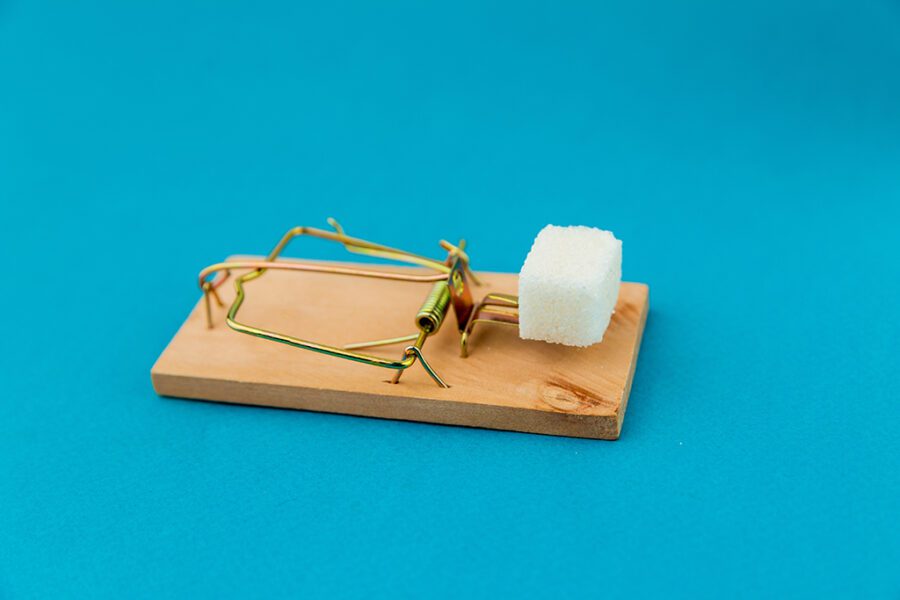
Wow, my blog “Is Data Mesh Fool’s Gold? Creating a Business-centric Data Strategy” created quite a stir. And that was my intention.
I actually believe that the Data Mesh is important data management and governance framework (yes, the Data Mesh is more of a framework than a technology) for helping organizations deliver a business-driven data strategy that enables the application of data and analytics to help deliver meaningful, timely, and accurate business and operational outcomes.
The Data Mesh is a useful framework for aligning data governance and data management responsibilities to the business units that are generating the data. That’s a great start, but insufficient for supporting enterprise-wide use cases that cross business units.
If we are going to use a Data Mesh to its full potential, then we must be aware of the traps in creating a distributed, domain-specific data structure that views “data-as-a-product,” with each domain handling their own data pipelines. If we want to get the most out of the Data Mesh, we best take the time to understand those traps so that we can engineer around them.
Providing Both Business Unit View AND Enterprise View
To make a data mesh a critical enabler of a business-driven data strategy, one needs to blend the Business Unit perspective of the Data Mesh with an Enterprise perspective to support the delivery of enterprise-wide, cross-business unit use cases and business outcomes.
It’s not a question of Business Units OR Enterprise. Yes, our business-driven data strategy needs to support both the Business Unit AND Enterprise data needs that deliver meaningful, timely, and accurate business and operational outcomes.
In a Data Mesh, each Business Unit has the responsibility for capturing and managing the data that Business Unit generates and uses to optimize that Business Unit’s use cases and operational objectives.
The challenge: if the data mesh addresses the capture and management of the data necessary to support the individual Business Unit’s use cases and operational objectives, who is addressing the capture and management of the data necessary to support the Enterprise-wide, cross-business unit use cases and operational objectives? That is, which organization has the responsibility for the capture and management of the data that may not be important to the individual Business Units’ operational objectives, but is important to the enterprise’s operational objectives?
For example, let’s look at what an organization would need to do from a Data Mesh perspective to optimize the Order-to-Case (OTC) business process.
The Order-to-Cash (OTC) is an enterprise-wide business process that crosses business units in the process of receiving and fulfilling customers’ requests for goods or services.
Supporting the enterprise data and analytic requirements for optimizing the Order-to-Cash process might require the Business Units to capture and manage data that may not be necessary for optimizing their own operations but are critical for optimizing upstream and downstream OTC use cases including Order Management, Credit Management, Order Fulfillment, Order Shipping, Customer Invoicing, Accounts Receivable, and Payments Collections and Reconciliation (Figure 1).
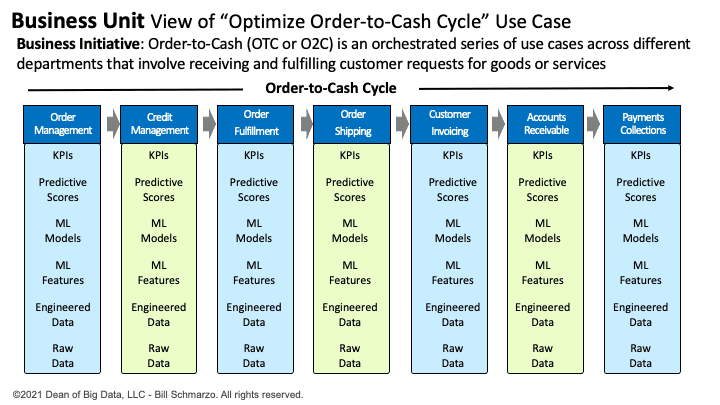
Figure 1: Data Mesh Business Unit View of Order-to-Cash Cycle
Unfortunately, there are gaps between the Business Units where additional data – data outside of the needs of the Business Unit – needs to be captured and managed to support the upstream and downstream Business Units and Enterprise data needs. Each business unit needs to consider more than just their data needs, the Business Units need to also consider the data needs of the Enterprise. Consequently, Business Units may be required to capture and manage data that doesn’t necessarily improve the performance of their business unit but is critical in supporting the performance of the enterprise and other Business Units (Figure 2).
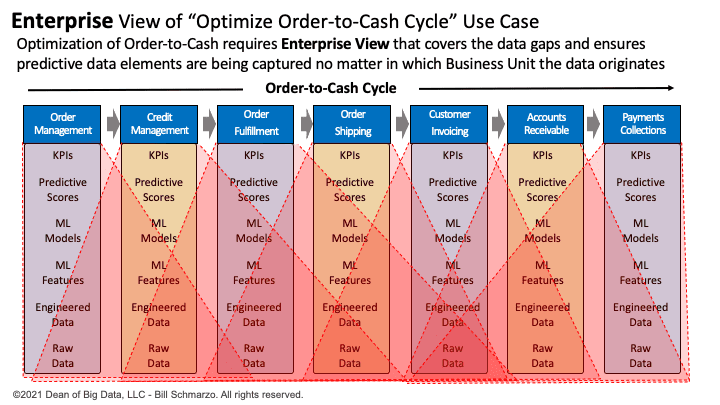
Figure 2: Enterprise View of the Order-to-Cash Data and Analytic Requirements
But wait, there is another benefit for blending the Business Unit and Enterprise views, and that is to enable the creation of enterprise-wide analytic scores (predicted behavioral and performance propensities) that can support each Business Units’ use cases but from an enterprise-wide perspective.
We can create enterprise analytic scores – such as Customer Financial Health Score, Customer Credit Risk Score, and Customer Potential Lifetime Value (LTV) Score – that can be used to optimize the enterprise view of the Order-to-Cash use case, while helping each Business Unit (Order Management, Credit Management, etc.) optimize their specific use cases (Figure 3).
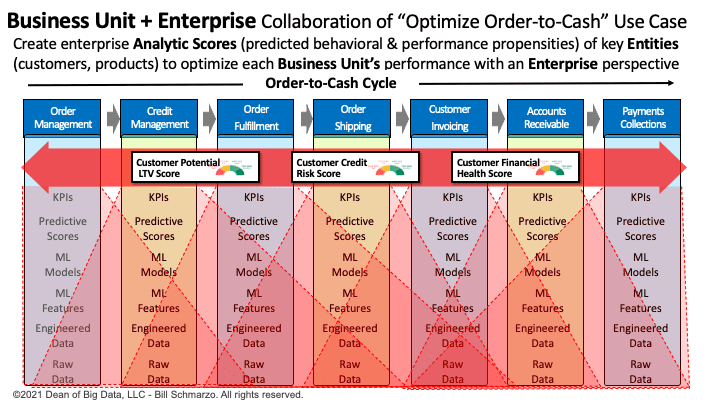
Figure 3: Business Unit + Enterprise Collaboration of Order-to-Cash Use Case
In this example (Figure 3), the enterprise’s most valuable customers are given the appropriate level of financial and service care in consideration of their larger “value” to the enterprise, so Business Units don’t treat a highly valuable customer (high Potential Lifetime Value + high Financial Health + low Credit Risk) inconsistent with the enterprise’s determination of the value of that customer.
If each Business Unit independently calculated and applied their own “Customer Potential Lifetime Value Score”, then customers that are valuable to the larger enterprise may get inconsistent treatment and support as they interact with the different Business Units.
Summary: Data Mesh and The Power of “And” Thinking
The Data Mesh can play an important role in helping organizations create a data-driven data strategy, but only if we blend and synergize across the Business Units AND Enterprise data and analytic requirements. To make that happen, we must:
- Drive enterprise-wide, cross-business unit collaboration to create analytic and data assets that can be share, reused, and continuously refined that benefit both the Business Units AND the Enterprise use cases and operational objectives
- Provide a holistic view and treatment of the organization’s most valuable business entities (customers, vendors / suppliers, products, stores, distribution centers, manufacturing plants, employees, etc.) to ensure that each business unit is optimizing their operations in light of overall importance of these business assets.
- Create Enterprise-level analytics and analytic scores that help the Business Units’ optimize their performance and use case in light of the larger Enterprise business objectives.
Also, organizations should create a Data Management & Governance Council to oversee and drive the collaboration, synergizing, and innovating between the Business Units and Enterprise perspectives and requirements in the creation, reuse, and continuous refinement of the organization’s data and analytics assets (Figure 4).
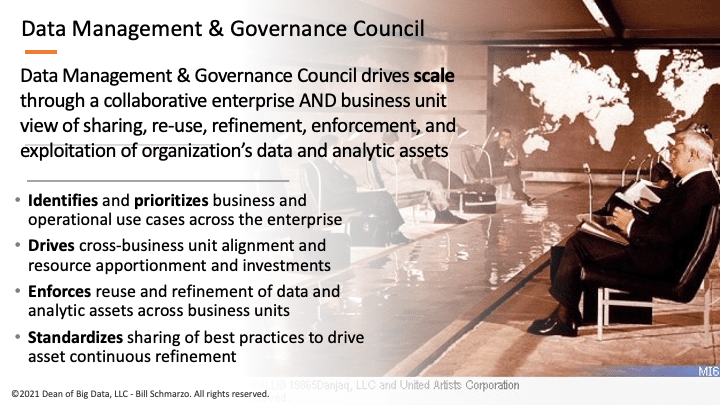
Figure 4: SPECTRE Data Management & Governance Council
By the way, I’d recommend modeling that council after SPECTRE – the “Special Executive for Collaboration, Transparency, Reuse, and Economics (of Data)” – taken from the James Bond book and movie series (a very effective organization in driving organizational compliance and adherence to the organizational mission).
The Data Mesh is an important component in creating a business-driven data strategy, but Data Mesh is NOT a data strategy. As I said in my previous blog:
We must remember to not let technology-lead “solutions” distract the organization from the hard work to identify and build organizational alignment around the organization’s business and operational processes (use cases) where the application of data and analytics can derive and drive new sources of customer, product, service, and operational value.
An organization’s business-driven data strategy should 1) start by understanding how the organization produces value and measures the effectiveness of that value creation, then 2) identifying, validating, valuing, and prioritizing the role of data and analytics to support the organization’s value creation processes.
Follow that advice, and you can avoid an unpleasant encounter with Ernst Stavro Blofeld.