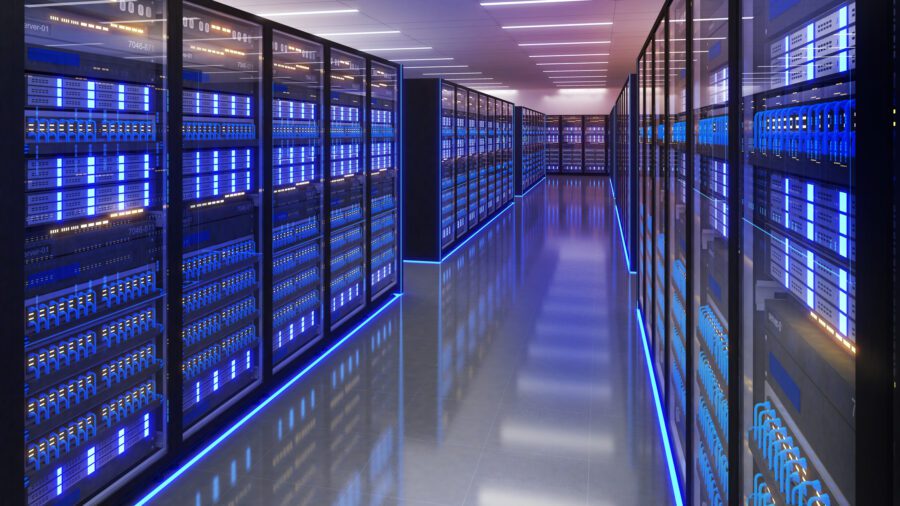
Data centers are consuming a massive amount of power. The easiest solution is to raise temperatures, driving resource utilization down. However, that complicates hardware preservation and building upkeep.
What if decision-makers could see into the future? Predictive analytics technology can help them find data-driven solutions among the noise, enabling energy consumption optimization. Here’s how that would work — and why it’s beneficial.
Data centers are consuming too much power
Data center electricity consumption is increasing exponentially. According to the International Energy Agency, it could more than double by 2026, going from 460 terawatt-hours in 2022 to 1,000 terawatt-hours in 2026. Since this strains local power grids, managers are faced with a difficult decision — invest in expensive power solutions or increase the building’s temperature.
Raising the temperature can reduce energy consumption and lower electricity costs. However, information technology (IT) equipment can only get so hot.
While enterprise-grade hard disk drives are designed to withstand operating temperatures between 41 degrees Fahrenheit and 140 degrees Fahrenheit, their failure rate increases by 30% for every 9 degrees above the maximum threshold. In the age of constant connectivity, the risk of unplanned downtime due to equipment failure is unacceptable.
Hyperscalers have unparalleled access to enterprise-grade, heat-resistant hardware. For smaller operations, the heat of rising temperatures may feel more intense. For instance, while a typical solid-state drive should last up to one decade, it can corrupt after just two years if temperatures consistently exceed 70 degrees Fahrenheit.
Modern data center cooling systems are considerably more energy-efficient than their predecessors. For instance, immersion — a version of liquid cooling that uses a closed-looped pipe system — is up to 4,000 times more efficient than air cooling. Even so, they still require an immense amount of power.
Resource-intensive applications like artificial intelligence (AI), edge computing and cloud infrastructure are becoming more prominent, offsetting the gains made by low-power cooling solutions. One of the only ways to effectively reduce electricity consumption is to leverage a building-specific, data-driven strategy. This is where predictive analytics comes into play.
Predictive analytics models
Predictive analytics models use statistical modeling, data science techniques or machine learning technology to forecast likely outcomes accurately.
1. Decision tree
In a decision tree, variables are categorized by their relationships. They branch out sequentially, following the outcome of each choice. They are easy to understand — even for those outside the IT department — since each step is visualized and sequenced.
2. Regression model
A regression model is a supervised learning technique for machine learning algorithms. It uses statistical methods to analyze the relationship between one dependent variable and multiple independent variables — energy consumption and central processing unit usage, for example.
3. Time series analysis
A time series analysis analyzes data points collected over a period of time at specific, consistent intervals. One common example is weather patterns — data science professionals can use this method to predict when it will rain next based on daily precipitation information.
4. Forecast model
The forecast model is among the most common predictive analytics models. It uses historical and current input data to produce a numerical value. It can consider multiple independent variables simultaneously.
The benefits of leveraging predictive analytics models
Data centers have become more efficient over the years. Globally, the average power usage effectiveness (PUE) rating decreased from about 2.5 in 2007 to 1.56 in 2024. However, with resource-intensive technologies becoming the norm, decision-makers must act quickly for more significant gains.
With predictive analytics technology, they can consistently optimize energy consumption and resource allocation, reducing energy waste and saving money. According to the National Renewable Energy Laboratory, data centers focusing on energy efficiency typically see a PUE rating of 1.2 or less.
Another benefit is the ability to leverage predictive maintenance. Their data-driven, granular insights allow them to fix hardware malfunctions and natural wear before problems occur. This keeps servers, cooling units and ventilation systems in optimal shape, which translates to lower operational costs.
Statistical models and machine learning algorithms cost relatively little — they need no salary, benefits or overtime pay — so running them does not significantly cut into savings.
Since temperatures stay down despite no increased electricity usage, damage to servers, switches, routers and storage systems is avoided. While state-of-the-art IT equipment can handle up to 80 degrees Fahrenheit, experts disagree on whether such higher temperatures are good for equipment.
This solution is incredibly beneficial for data center hot spots — areas where server input air gets exceptionally hot. According to the American Society of Heating, Refrigerating, and Air Conditioning Engineers, the temperature can easily exceed 81 degrees Fahrenheit as heat naturally rises and accumulates at the tops of rack units.
How does this solution optimize energy consumption?
Predictive analytics technology typically considers historical data whether it uses statistical modeling techniques or machine learning algorithms. It considers variables like weather, ambient air temperature, electricity consumption, humidity, server load, airflow patterns and equipment density.
With these internal and external factors, the model can forecast how much electricity a data center will use. Predictions remain accurate since machine learning algorithms get more refined as they absorb more knowledge.
These systems can work around the clock, adjusting their forecasts in real time. This level of dynamic granularity is essential because variables like external temperature and server load fluctuate throughout the day — static, periodic output would quickly become outdated.
How data center managers can use predictive analytics
Here are ways data centers can use predictive analytics to optimize energy consumption.
Workload management
This approach identifies energy-intensive processes to find ways to reduce the IT equipment’s power usage. When managers have data-driven insights, they know whether to schedule processes at off-peak hours, fix resource allocation or upgrade aging hardware.
Energy efficiency
An energy efficiency forecast tells decision-makers how efficiently systems will consume electricity based on various factors. It looks at the building as a whole to identify areas of opportunity, such as changing the cooling system or moving outtake vents.
Management can determine the best methods to optimize power consumption by predicting future electricity needs. Making changes in advance prevents undesirable energy waste — they keep costs down while conserving energy.
Cooling system performance
The IT department can predict cooling system performance and repairs to keep it in the best shape possible. This way, it doesn’t need to expend as many resources to cool hardware down.
Tips for optimizing predictive models and algorithms
Managers should prioritize data to ensure their predictions remain accurate and relevant. Having high-quality, up-to-date information is critical. They should have a worker who periodically revisits the training dataset to ensure the integrity and correctness of sources.
Another essential strategy many overlook is to set a cut-off date for data. Information eventually becomes irrelevant, outdated or inaccurate over time due to model shift or concept drift — a change in input and output relationships that invalidates the training dataset. For example, if leaders forget to account for seasonality, their weather metrics will be skewed.
A dedicated AI engineer or IT professional can ensure the machine learning algorithm or statistical model stays on track. This human-in-the-loop strategy helps ensure companies avoid costly mistakes.
Cool data centers faster with predictive analytics models
Predictive analytics models effectively give professionals a glimpse into the future, allowing them to optimize their buildings in ways they never would’ve thought otherwise. Since their forecasts will get more accurate and in-depth over time, they can continuously improve.