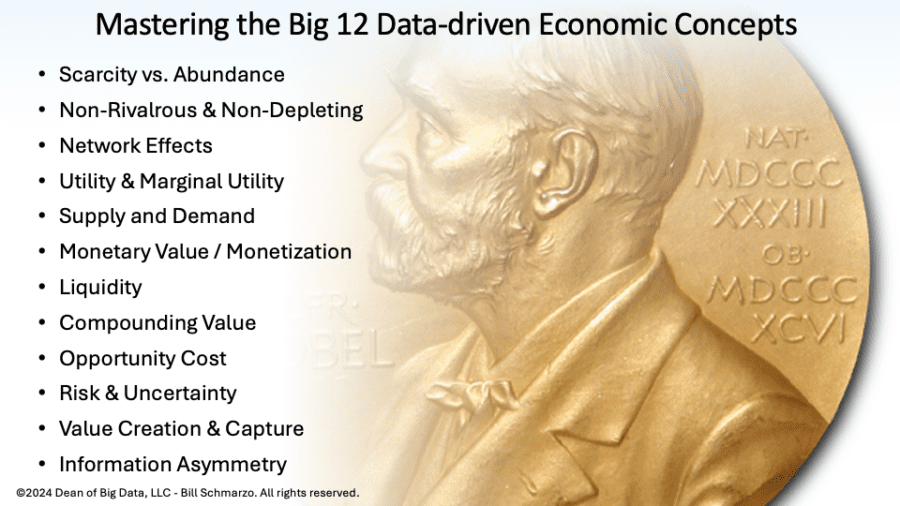
“If you want to change the game, change the frame.”
The game has certainly changed with the data era, and maybe the most drastic change driven by data has occurred in economics, the foundation upon which our modern society is built and sustained. Understanding and mastering the transition from “traditional” economics to modern data-driven economics has become necessary for survival and growth. Unlike traditional economic principles that focus on scarcity, rivalry, and finite resources, data economics introduces a new landscape where data is abundant, non-depleting, and capable of generating compounding value when harnessed effectively.
- Traditional Economics: Traditional economics focuses on efficiently allocating scarce, finite resources to maximize value and meet human needs.
- Data Economics: Data-driven economics emphasizes using abundant, non-depleting data to compound value through deeper customer, product, service, operational, and societal insights, insights-fueled innovation, and optimized operational processes and business models.
Using data as a strategic asset helps organizations make smarter decisions, improve efficiency, and discover new revenue streams. As data is used across various scenarios, its value grows, allowing organizations to enhance their insights and performance. This enables companies to create more agile, responsive business models driven by the endless potential of modern, data-driven economic concepts.
In this blog, I will discuss the difference between “traditional” economics and data-driven economics and introduce twelve “traditional” economic concepts that are being transformed by advancements in data economics.
Twelve (12) Data-driven Economic Concepts
These 12 data-driven economic concepts can be powerful drivers of innovation by fundamentally changing how organizations optimize their resources, improve their decision-making processes, and uncover and exploit new strategic opportunities.
- Scarcity vs. Abundance: By treating data as an abundant resource, organizations can explore new ways to innovate without the traditional limitations of finite resources. This allows companies to experiment, iterate, and scale faster using data-driven models.
- Non-Rivalrous and Non-Depleting: Data’s non-depleting nature allows it to be reused across various use cases without reducing availability. This enables cross-functional collaboration, leading to innovation through diverse perspectives.
- Network Effects: Data value grows with more connections, generating richer insights and uncovering previously hidden patterns, sparking new product innovations, personalized customer experiences, and powering operational improvements.
- Utility and Marginal Utility: With increasing returns on data (unlike diminishing returns in traditional economics), organizations can continually refine their offerings, using data to enhance processes and products, making them more accurate and efficient (and valuable) over time.
- Supply and Demand: Shifting focus from scarce resources to actionable insights unlocks innovation opportunities. Organizations can experiment with new operational models using insights to meet the growing demand for personalized, predictive, and real-time solutions.
- Monetary Value and Monetization: Data offers monetization through direct selling or improving operational efficiencies. This enables companies to innovate in terms of products and services and how they generate revenue and capture value.
- Liquidity: Improving data liquidity allows data to move seamlessly across use cases, fostering an environment where innovation can flourish. It enables real-time experimentation, feedback loops, and faster iterations of new ideas and strategies.
- Compounding Value: As data’s value compounds over time, organizations can build long-term innovation pipelines. They can evolve products and services based on more accurate data-driven insights by continuously integrating new data.
- Opportunity Cost: Recognizing the opportunity cost of not exploiting data pushes organizations to constantly explore new ways to capture new data to streamline processes, enter new markets, or develop innovative products and services.
- Risk and Uncertainty: Data analytics reduces uncertainty, enabling organizations to take calculated risks on innovative ideas. By predicting outcomes more accurately, organizations can reduce the fear of failure and foster a culture of experimentation.
- Value Creation and Capture: By focusing on data-driven insights, organizations can identify new ways to serve customers, optimize processes, acquire customers, and dominate new markets. This insight-driven approach is critical to driving sustained innovation across products and services.
- Information Asymmetry: Reducing information asymmetry unleashes new opportunities for data-driven market solutions. When all parties can access transparent, real-time data, competition shifts toward innovation in service, efficiency, and customer experience.
Figure 1 summarizes the transition from traditional to data-driven economics.
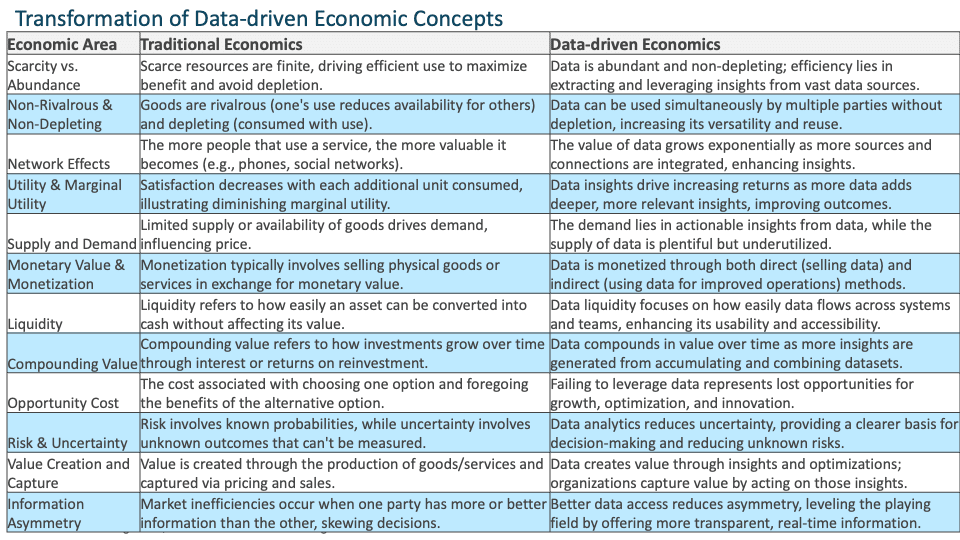
Figure 1: Transformation of Data-driven Economic Concepts
Data-driven Economic Examples
Nothing illustrates the potential for economic transformation better than real-world stories of organizations that understand the power of data. These stories provide concrete examples for each of the 12 concepts of data economics.
- Scarcity vs. Abundance. Amazon continuously collects and reuses vast amounts of customer behavioral data across multiple use cases, such as personalizing shopping experiences, optimizing logistics, and predicting demand trends—something impossible with limited physical resources.
- Non-Rivalrous and Non-Depleting. Google Maps uses traffic data from millions of users to provide real-time navigation. The non-rivalrous nature of data means everyone can benefit from the same data without diminishing its usefulness.
- Network Effects. Facebook’s platform grows more valuable as more users share their preferences. The network effect increases the platform’s value as each additional user generates more behavioral data, enhancing advertising effectiveness.
- Utility and Marginal Utility. The marginal utility from users’ viewing habits allows Netflix to refine its recommendation algorithms, providing increasingly relevant suggestions that enhance customer acquisition, cross-sell / up-sell, and retention.
- Supply and Demand. In advertising, deep customer insights drive the demand for highly targeted campaigns. Facebook and Google command high advertising rates because they supply valuable insights from vast insights about users’ behavioral tendencies.
- Monetary Value and Monetization. Experian directly monetizes its data by selling credit scores to financial institutions. Meanwhile, companies like Uber and Lyft monetize data indirectly by optimizing the customer experience, which improves customer acquisition, cross-selling, and retention.
- Liquidity. Cloud platforms amplify data liquidity by making data easily accessible. This removes customers’ data storage, access, and real-time analysis hurdles, providing more resources to focus on improved decision-making, operational efficiencies, and innovation efforts.
- Compounding Value. Tesla compounds the value of its autonomous vehicle data as each additional car generates more data, continuously improving the performance, reliability, safety, and value of Tesla’s autonomous vehicles.
- Opportunity Cost. Blockbuster’s failure to leverage data while Netflix used customer viewing data to enhance its streaming platform, power customer recommendations, and improve customer acquisition illustrates the data economics opportunity cost.
- Risk and Uncertainty. Insurance companies use data analytics to reduce uncertainty and risk. By analyzing driving habits through telematics data, they reduce their exposure to high-risk drivers while improving customer acquisition and retention by providing better rates to safer drivers.
- Value Creation and Capture. Spotify captures value through subscriptions and advertising revenue generated by keeping users engaged longer with tailored music experiences, driving customer retention and customer references.
- Information Asymmetry. Zillow has reduced information asymmetry by providing data on home prices, historical trends, and neighborhood comparisons, making the market more efficient and reducing the advantage of insiders.
These examples highlight how data-driven economics transforms traditional economic concepts to reshape operational processes and business models.
Checklist for Exploiting Data-driven Economics
Understanding the potential of data-driven economics is crucial. Here are five (5) actions your organization can take today to exploit data-driven economics (see Figure 1):
Action #1. Identify Value-creation Levers
- Map Business Outcomes to Data: Start by mapping your organization’s key business outcomes (e.g., profitability, customer satisfaction) to specific data sources. Identify which data is most relevant to understanding performance in these areas.
- Create Stakeholder Journey Maps: Use Journey Maps to identify where and how each stakeholder creates value (gains) and the impediments (pains) to value creation. Determine how data can optimize the stakeholders’ gains and alleviate the pains.
- Engage Business Leaders: Involve department heads and business leaders in identifying the most critical value levers. Their deep understanding of the business ensures that the focus remains on areas where data can have the most significant impact.
Action #2. Align Data and AI Strategy with Value Creation
- Prioritize Use Cases Based on Value and Feasibility: Prioritize your data projects based on their potential value balanced against implementation feasibility. Focus on the projects delivering the most significant business value at the lowest implementation risk.
- Drive Stakeholder Engagement on Day 0. Bring key stakeholders into the initial design process. These individuals have the insights to develop more relevant and effective models and play a crucial role in analytic execution and feedback processes.
- Establish Broader Economic Metrics: Measure value across multiple dimensions, including financial, customer, operational, community, society, and environmental. Track how data initiatives contribute to broader economic and societal goals, ensuring continuous learning and improvement.
Action #3. Develop a Data Economics-based Business Model
- Explore Net New Data Monetization Opportunities: Analyze how data can become a revenue-generating asset by creating new data products or offering insights-as-a-service to customers and partners.
- Shift Toward Predictive and Prescriptive Analytics: Focus on predictive and prescriptive models that help optimize real-time decision-making and anticipate future trends that drive new sources of customer, product, service, operational, and societal value.
- Create Stakeholder-centric Value-creation Strategies: Utilize data to comprehend key stakeholder behaviors, preferences, and pain points. Develop personalized experiences or services to enhance stakeholder satisfaction and operational effectiveness.
Action #4. Invest in an Agile and Extensible Data Infrastructure
- Implement Scalable Data and AI Architectures: Invest in scalable infrastructures that enable flexible, scalable storage and processing of vast data sets. This will allow faster access to insights and greater agility in responding to business needs.
- Adopt Incremental Data Management Approach: Focus your data management efforts, such as improving data quality, implementing data governance, and enhancing data, on the data sources directly related to your use case roadmap.
- Build Robust Data Governance Practices: Establish transparent governance practices to ensure data quality, accuracy, and regulation compliance. Effective data governance is critical to maximizing the value of your data while ensuring security and trust.
Action #5. Foster a Culture of Data-driven Value-creation and Innovation
- Develop AI & Data Literacy Programs: Provide organizational training to increase data literacy, ensuring employees understand how to access and use data to enhance decision-making. I know a book your organization might want to invest in.
- Embed data in decision-making processes: Make data-driven decision-making a core part of your business. Provide feedback on decision effectiveness so that you create an organization that continuously learns and adapts based on decision effectiveness.
- Adapt Rewards Structure: Align compensation and rewards to incentivize data-driven value-creation collaboration, innovation, and continuous learning, ensuring employees are recognized for using data to drive business outcomes and innovation.
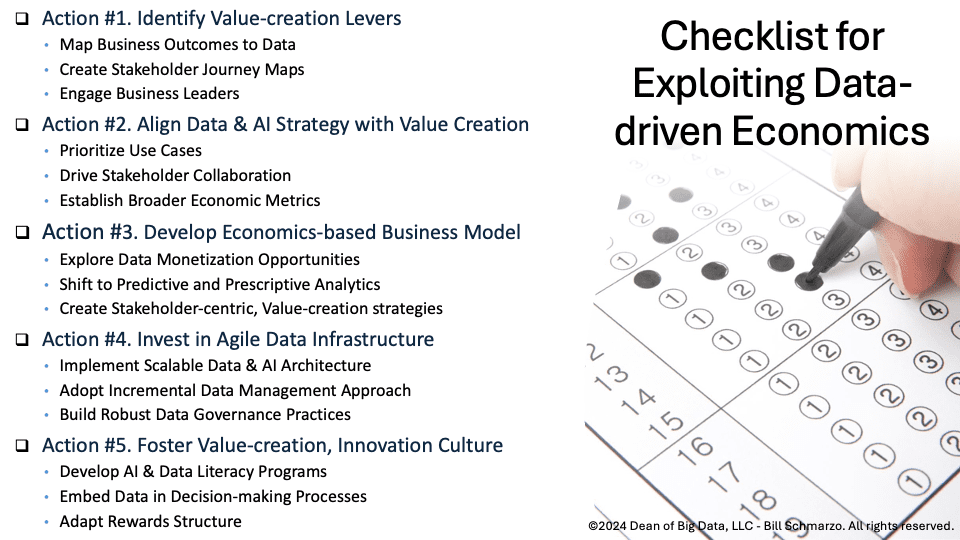
Figure 2: Checklist for Exploiting Data-driven Economics
Organizations can systematically apply data-driven economics to prioritize value creation use cases, making suitable infrastructure investments, and fostering a culture of data-driven value creation can unlock the economic potential of your data.