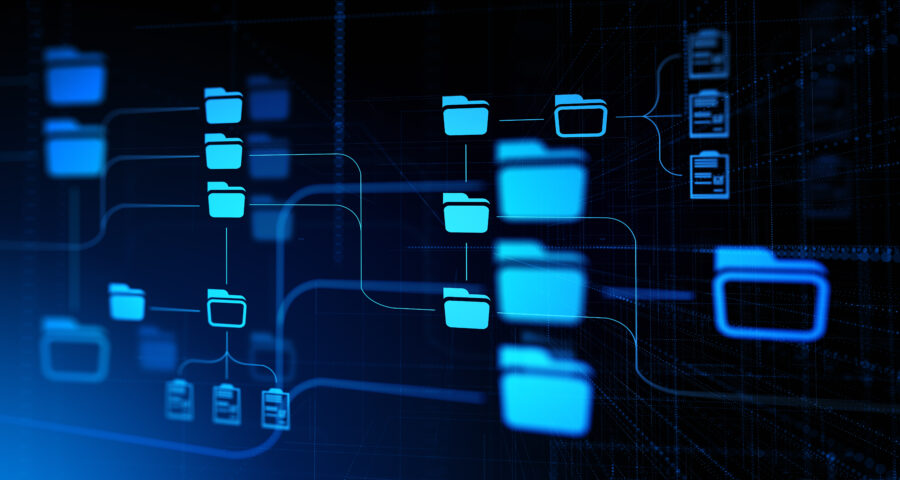
A machine learning (ML) data catalog is a system that leverages machine learning and metadata to automate data management processes. It minimizes manual effort and enhances data accuracy through automated models. If you’re looking to understand ML data catalogs, we’ll cover it here.
What is a machine learning data catalog?
A machine learning data catalog automates various data management tasks:
- Discovery
- Classification
- Profiling
- Lineage tracking
- Governance
- Auditing
Using machine learning algorithms and metadata, these catalogs automate the above tasks. They do this by continuously scanning metadata to tag and organize data assets, eliminating the need for repetitive manual work.
Data stewards often struggle with the massive amount of data objects. With an ML data catalog, ML algorithms identify patterns and relationships in the data, saving countless hours over the traditional manual methods.
What’s the difference between AI, automated, and machine learning data catalogs?
While AI, automated, and machine learning data catalogs are interrelated, they are not identical. AI data catalogs enhance data management with AI-driven insights and natural language queries. Automated data catalogs, on the other hand, don’t necessarily use AI, but they do share a focus on reducing manual effort through process automation. Machine learning data catalogs also aim to reduce the manual efforts associated with data catalog tasks, but they use ML to automate processes like classification and profiling. Essentially, they’re three different approaches with a common goal.
Key capabilities of a machine learning data catalog
Semantic data search
Machine learning data catalogs use semantic search to find relevant data assets quickly and accurately. They understand the context and meaning behind search queries, improving data discoverability.
Automated metadata extraction
ML data catalogs automatically extract metadata from various data sources.
Automated data discovery
ML data catalogs also continuously scan and monitor the organization’s data environment to discover new data assets. They can identify and index new data as soon as it is created or modified.
Automated data tagging and classification
Classification and tagging of data assets is automated, and in the process data types like “PII” or “sensitive data” are identified and categorized.
Data profiling
Data assets are analyzed by structure and relationship. Data quality scores are assigned and anomaly detection provides insights into data patterns and distributions.
Automated data lineage mapping
These catalogs map data asset lineage and movement across the organization’s data environment, providing a clear view of data flow and dependencies. These activities are essential for impact analysis and compliance.
Data stewardship
ML tools and features assist data stewards in managing data efficiently. They get intelligent recommendations for data integrity maintenance and compliance with regulatory requirements.
The evolution of machine learning data catalogs
Data catalogs have evolved significantly from the early days of library card catalogs to digital data dictionaries in the 1960s. The advent of big data in the 2000s led to the development of more advanced data cataloging solutions to handle the exponential growth of data. Traditional data catalogs required extensive manual effort and became inadequate for modern data needs, leading to the creation of machine learning data catalogs (MLDCs).
MLDCs use active metadata management to automate continuous metadata collection, analysis, and updating, making data cataloging more efficient. Advances in AI have further enhanced machine learning data catalogs, enabling them to mimic human intelligence, provide data context, and offer intelligent recommendations for easier data discovery.
Benefits of a machine learning data catalog
A machine learning data catalog offers numerous benefits:
- Improved data management: Enhances efficiency in managing data tasks such as classification and profiling, ensuring data is updated and accurate.
- Identification of value-creating analytics and AI initiatives: Provides insights to prioritize analytics and AI projects with the highest business value.
- Mitigation of risk exposure: Automatically detects and classifies sensitive data, implementing security measures to reduce risk.
- Enhanced data governance: Enforces data governance policies to maintain data integrity and compliance.
- Improved data literacy: Adds business context to data at scale, helping users search and understand data more efficiently.
- Increased productivity: Reduces manual data management tasks, freeing up time for high-value analytical work and decision-making.
Any machine learning data catalog will address the main pain points of data cataloging through sophisticated technical systems. Knowledge graph architecture ensures data is contextually enriched. Your ideal ML data catalog is agile and scalable, supports hybrid architectures, and enriches data with metadata. Ultimately, your data management transforms from a cumbersome chore into a streamlined, efficient process, empowering your organization to harness the full potential of its data.