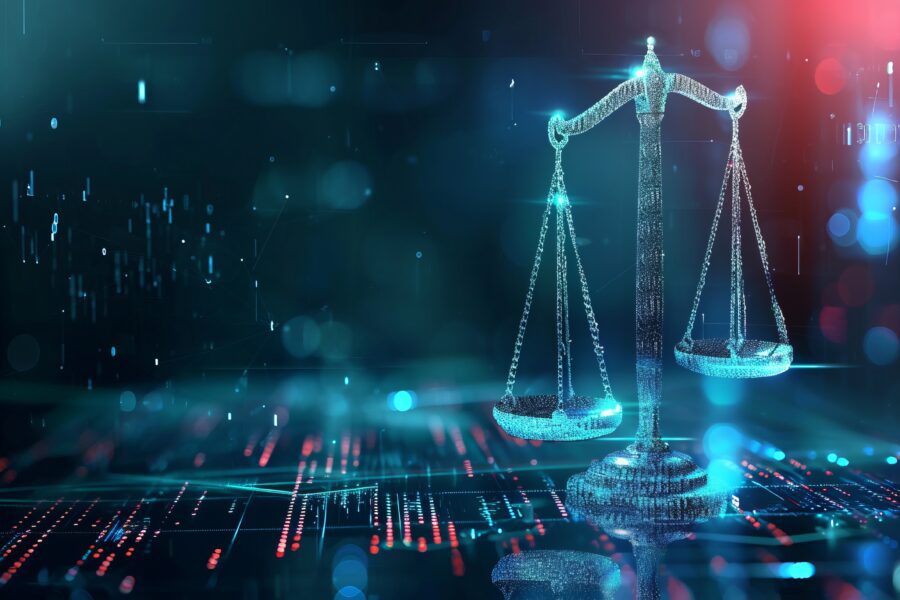
A new blog post by Google, Introducing the Coalition for Secure AI (CoSAI) and founding member organizations, stated that, “We’re introducing the Coalition for Secure AI (CoSAI). We’ve been working to pull this coalition together over the past year, in order to advance comprehensive security measures for addressing the unique risks that come with AI, for both issues that arise in real time and those over the horizon. First three areas of focus the coalition will tackle in collaboration with industry and academia: Software Supply Chain Security for AI systems, Preparing defenders for a changing cybersecurity landscape and AI security governance. CoSAI includes founding members Amazon, Anthropic, Chainguard, Cisco, Cohere, GenLab, IBM, Intel, Microsoft, NVIDIA, OpenAI, Paypal and Wiz — and it will be housed under OASIS Open, the international standards and open source consortium.”
Although conversations for AI safety, security alignment to human values and regulation include biological and existential threats, current risks are misinformation and multimodal deepfakes—images, audios and videos.
Among research against current risks and future likelihoods of artificial general intelligence [AGI] or artificial superintelligence [ASI], two new options for technical regulation could be explored:
Web crawling and scraping of number arrays—or embeddings
Currently there is no way to easily identify the AI source of any misinformation or deepfake—image, audio or video. There are so many AI tools in search engine results that provide services with outputs that cannot be traced. Many of them come with terms and requirements, but whatever their outputs—if potentially harmful, they never get known on time to expect it at the destination [public or private].
Simply, around some keywords, it should be possible to monitor some outputs of AI tools, to know where misinformation or deepfakes originated, to then seek out the possible destination and create alerts.
An approach towards this could be AI tools, crawled and available in search results, have their embeddings scraped, such that those around certain keywords can be tracked, for their tokens, to know the contents and where they might be deployed.
Simply, if an AI tool is used for voice impersonation with keywords that could be used for harm or deception, the token can be scraped, so wherever it might be used, reporting is sent in the direction, to expect and prevent harm. The same applies to images and videos, as well as misinformation.
The purpose is to have an embeddings database of collective safety, where misuses are tracked from their sources, so wherever they might go, there could be an alert.
For now, some frontier AI tools do not permit some of the results that some smaller AI tools permit. These smaller AI tools may not necessarily report or do much about misuse cases. The objective is that if they are indexed on search engines, their embeddings should be made available to be scraped using a new robot.txt or data API.
These scrapped embeddings can then become a new database to explore formats for other AI tools that may not be indexed, and to have an equivalent of a digital hashing—or say AI hash function—so that they can also be sought for identification.
Technically, this architecture would follow the already established crawling and scraping protocols, only that there would be explorations for collection of large vector dimensions as well as possible summations that may result in an equivalent of a hash function.
If this works, it may be useful to explore how intent or minor goal-direction might emerge in LLMs. It may also be useful to explore new ways to fight hallucinations as well as track bioweapons risks. If the technical path is established, especially in varied ways, options could be presented to AI tools managers to boost adoption.
Penalty-Tuning for LLMs
Guardrails are effective for Large Language Models [LLMs]. Yet, by some prompt injection attacks or jailbreaks, they sometimes output what they should not. Also, some LLMs generate misinformation and deepfakes. LLMs may not have self-awareness like humans, but they have—large—language awareness. They also have some usage awareness since they appear to have a stop for other prompts when in use.
It should be possible to have a way to penalize LLMs, by language, usage or compute, if they output misinformation or deepfakes. Even if this penalty is temporary, it should be something to show that they could lose access to an important part of their function, which they can know about and be somewhat telling.
In the era of AI, it may not be enough to censure individuals if AI enables extra capabilities and nothing happens to AI. Also, the rate of evasion by AI should make it possible to have LLMs be partly accountable for their outputs.
For humans, there are consequences to actions, since intelligence can be misappropriated. LLMs transmit intelligence, it should be possible to penalize them, technically, as a form of regulation.
Penalty for LLMs may be simple to complex, temporal to permanent. It could come by penalty-tuning, following pre-training, so that it is aware of what it could lose.
When a chatbot observes that it is getting inputs beyond its guardrails, it should be able to restrict the user with its next response, so that it can avoid being restricted itself. There could be many tries in different misuse directions for chatbots. When the chatbot notices, it should not only say it cannot, but either slow its next response or totally shutdown that user, while noting the way the prompt came, so that when tried elsewhere within an interval, it slows again, penalizing the user, to avoid its own penalization. This could track directly towards safety for AGI or ASI in future, starting from now.
Exploring how this penalty may work, such as to prevent AI from causing harm could be another direction for research for AI safety and alignment. There could be a general standard around this, especially for AI tools indexed on search engines as well. The purpose is to provide consequential technical checks for LLMs directly, aside plain regulations.