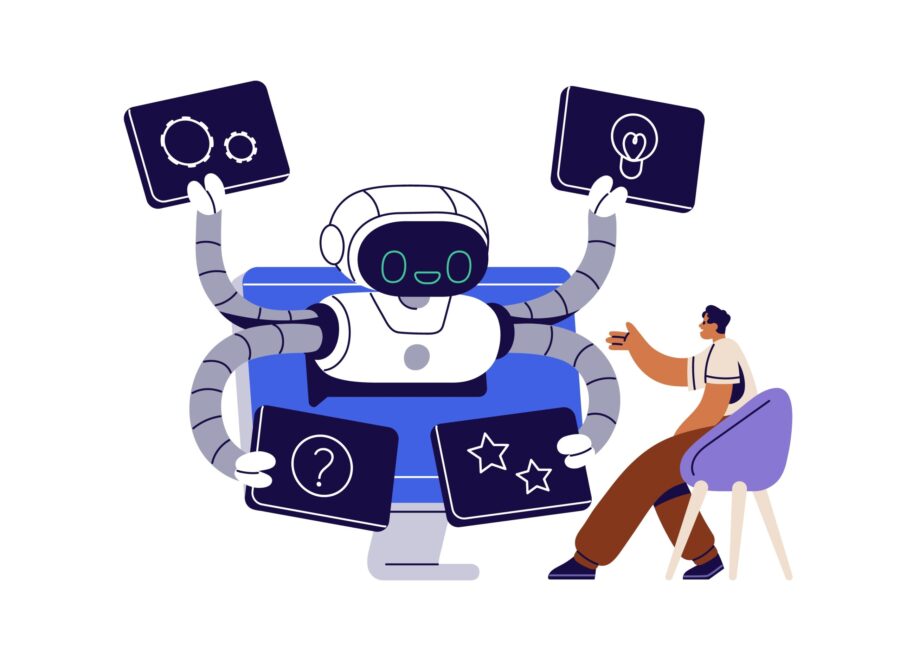
There is a recent article in The Economist, Large, creative AI models will transform lives and labour markets, describing how LLMs work. It states that “First, the language of the query is converted from words, which neural networks cannot handle, into a representative set of numbers. GPT-3, which powered an earlier version of Chatgpt, does this by splitting text into chunks of characters, called tokens, which commonly occur together. These tokens can be words, like “love” or “are”, affixes, like “dis” or “ised”, and punctuation, like “?”. GPT-3’s dictionary contains details of 50,257 tokens.”
“The LLM then deploys its “attention network” to make connections between different parts of the prompt. Its attention network slowly encodes the structure of the language it sees as numbers (called “weights”) within its neural network. Emergent abilities are all represented in some form within the llms’ training data (or the prompts they are given) but they do not become apparent until the LLMs cross a certain, very large, threshold in their size.”
The article mentions chunks of characters and autoregression. The first in the series left out some important parts of LLMs including parameters, pre-training, and so on. AI is not the human brain, but AI has a mind. The inner workings of LLMs including emergence, or emergent abilities, properties, or phenomena operate like a mind.
The overall function of any mind is to know. It may arise from a complex organ like the brain, it may be in the form of memory in a single-celled organism, or from an object system like a computer, or from human brain cells or organoids in the nervous system of murine.
Neurons or whatever simulates them result in the making of a mind or something with a broad mechanism for knowing. Feelings, emotions, reactions, knowledge, language, and so on, are known. Some systems do not have artificial neural networks but are able to know to an extent.
There are premises that neural networks were built on intended to mimic the brain. Many of those led to progress, but did not exactly get the mind. It is often said that the brain or more precisely, the mind generates predictions. It is this prediction generation or predictive coding, processing against errors that shaped LLMs.
The mind, however, does not make predictions. It functions in a way that appears so, but it does not. Cells and molecules of the brain structure, organize, construct or build the components of the mind. It is the components of the mind that operate what is labeled predictions.
When someone is speaking, typing, listening, or signing, there is often preparation for what may come next in the mind. Sometimes, it may seem like it should be one thing, but it is another. Other times, nothing may present. It is also this preparation that is sometimes used to recall things or the same way that something is set up to be remembered.
There is no exclusive prediction function in the mind. The mind, conceptually, has quantities and properties. Quantities relay to acquire properties to varying extents. It is the property that gets acquired in a moment that determines what an experience is, or simply what is known.
Quantities have early splits or go before, where some in a beam head in a direction like before so that others simply follow. If the input matches, there would be no changes, if not, the following quantities head in the right direction. This explains what is labeled as predictions. Quantities have old and new sequences. They can also be prioritized or pre-prioritized. Prioritization is what attention for transformers simulates.
Properties have thin and thick shapes. They have a principal spot where one goes to have the most domination. They also have bounce points. A thick property can merge some of its contents, resulting in creativity. Properties can be formed by quantities. Some properties are also natural, enabling things for humans that other organisms do not have.
How does the human mind work to be useful for explainable AI or interpretability, towards alignment? The human mind has a structure, functions, and components. For all it does for internal and external senses, how does it work, including for sentience, or knowing? Some of the answers to the unknowns for AI could emanate from the mind, boosting transparency.
David Stephen does research in theoretical neuroscience. He was a visiting scholar in medical entomology at the University of Illinois, Urbana-Champaign, UIUC. He did research in computer vision at Universitat Rovira i Virgili, URV, Tarragona.