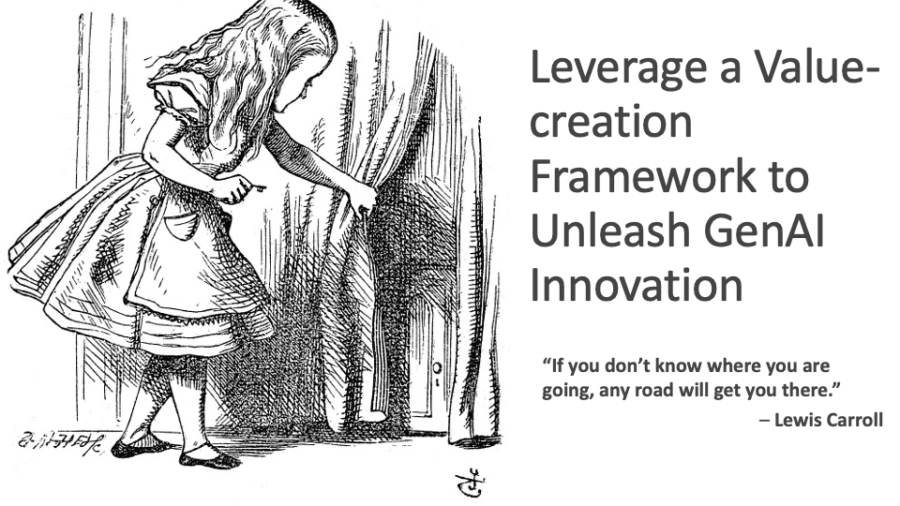
Note: there is a gift for those who read to the end of this blog.
“If you don’t know where you are going, any road will get you there.” – Lewis Carroll.
Generative AI tools like ChatGPT and CoPilot are revolutionizing how businesses and individuals approach decision-making. However, even the most advanced tools require a guiding framework to unlock their full potential. Without a clear destination and structured plan to achieve it, their outputs can lack relevance and actionable value. That’s where value-creation frameworks come into play. They offer a strategic roadmap for leveraging GenAI tools to explore opportunities, fuel ideation, and derive new sources of customer, operational, and societal value.
The Thinking Like a Data Scientist (TLADS) methodology is one such value-creation framework. This framework empowers guides to uncover high-value opportunities, align decisions with business objectives, and deliver ethical, measurable outcomes. By integrating TLADS with GenAI innovation-driven prompts, professionals can combine structured problem-solving with creative exploration to ensure their GenAI tool fuels more relevant and meaningful results.
This blog will explore how employing a value-creation framework like TLADS can help professionals and students use their GenAI tools to drive more actionable and impactful outcomes.
The Thinking Like a Data Scientist (TLADS) Framework
The Thinking Like a Data Scientist (TLADS) framework is an 8-step, collaborative methodology that blends data science, design thinking, and economic principles to leverage data and AI to deliver more meaningful, responsible, and ethical outcomes. The framework emphasizes aligning data and AI capabilities with strategic business and operational initiatives, empathizing and empowering key stakeholders and constituents, and fostering a culture of continuous learning and improvement (Figure 1).
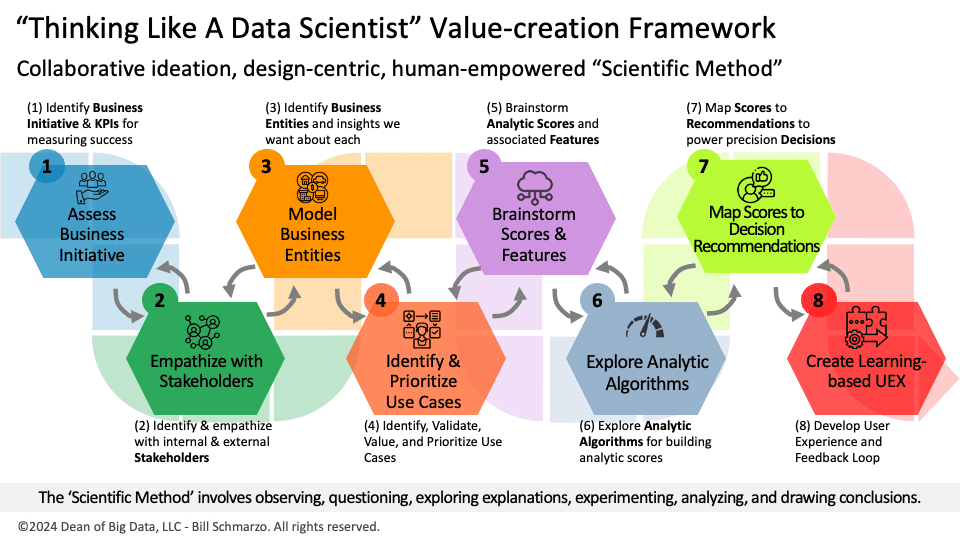
Figure 1: “Thinking Like a Data Scientist” Framework
For more information about the TLADS methodology and its supporting design templates, see the blog series “AI for Everyone: Learn How to Think Like a Data Scientist.”
Supercharging Generative AI and Innovation-Driven Prompts
Generative AI tools are powerful aids for fostering ideation and creativity. However, to fully leverage their capabilities, users must progress beyond standard question-and-answer interactions. These tools excel when combined with human curiosity and imagination, expanding the boundaries to unleash innovation and reveal transformative insights.
By integrating the TLADS framework with a guided exploration process like the Socratic Method or the Nine Categories of Innovation-Driven Prompt Engineering, professionals can embrace a systematic approach to tackle complex challenges, discover new opportunities, and innovate effectively. This combination ensures that every AI interaction is focused, creative, and results-oriented (Figure 2).
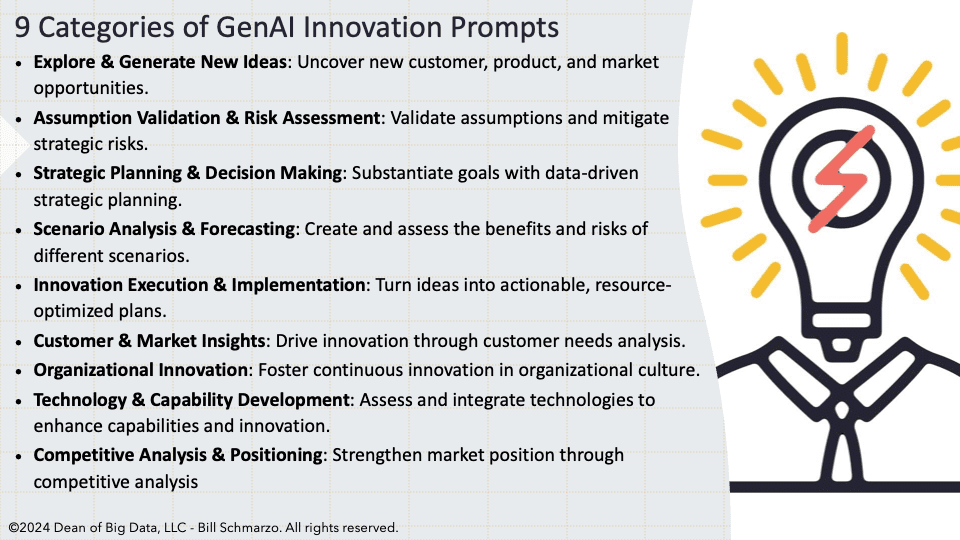
Figure 2: Nine Categories of GenAI Innovation Prompts
Getting Real: Integrating Innovation Prompts with TLADS
Let’s explore how the nine innovation prompts can seamlessly integrate with the TLADS methodology. Table 1 provides a detailed set of examples demonstrating how each step of the TLADS framework can be enhanced using the nine categories of innovation prompting. The depth of the table is intentional—it’s a rich topic that deserves thorough and actionable exploration to transition your GenAI usage from just productivity improvements to true innovation realization.
TLADS Step | Innovation Type | Sample GenAI Prompts |
Step 1: Assess Business Initiative | Exploration | “What are emerging opportunities in [industry] that align with our business objectives?” |
Trend Analysis | “What are the biggest market trends or challenges impacting [initiative]?” | |
Problem Decomposition | “What are the key components of [initiative] that need addressing to achieve success?” | |
Evaluation and Prioritization | “What initiatives from similar industries have delivered measurable ROI, and how can we adapt them?” | |
Scenario Planning | “What would happen to business outcomes if we adjusted our KPIs for [initiative] by 10%?” | |
Step 2: Empathize with Stakeholders | Stakeholder Empathy | “What motivates [stakeholder group] in their decision-making processes?” |
Exploration | “How do [stakeholder group] priorities vary across regions or segments?” | |
Risk Mitigation | “What stakeholder concerns could jeopardize the success of [initiative], and how can we address them?” | |
Feedback and Learning | “How can we create systems that gather stakeholder feedback consistently to refine our approach?” | |
Step 3: Model Business Entities | Exploration | “What new data sources or methods can improve our modeling of [entity] behaviors?” |
Solution Ideation | “How can we use entity-level analytics to uncover hidden behavioral trends for [entity]?” | |
Scenario Planning | “What would happen if external conditions (e.g., market trends, regulations) shifted significantly for [entity]?” | |
Risk Mitigation | “What are potential pitfalls in modeling [entity], and how can we address them?” | |
Step 4a: Identify & Assess Use Cases | Trend Analysis | “What are successful use cases in [industry] for solving similar challenges?” |
Evaluation and Prioritization | “Which use cases deliver the most immediate value, and why?” | |
Exploration | “What are some unconventional use cases that could leverage our unique data assets?” | |
Problem Decomposition | “How can we break down complex use cases into smaller, manageable steps for analysis?” | |
Feedback and Learning | “What can past use cases teach us about the risks or success factors for [initiative]?” | |
Step 4b: Prioritize Use Cases | Evaluation and Prioritization | “What criteria should we use to rank use cases by business impact and technical feasibility?” |
Risk Mitigation | “What are the execution risks for each use case, and how can we mitigate them?” | |
Scenario Planning | “How does prioritization change if we focus on short-term wins versus long-term gains?” | |
Step 5: Brainstorm Scores & Features | Solution Ideation | “What predictive features could be engineered to better measure [specific outcome]?” |
Exploration | “What advanced techniques, like transfer learning or feature stacking, could enhance feature engineering?” | |
Risk Mitigation | “What risks or biases might arise from using [specific feature] in our model?” | |
Trend Analysis | “What recent advancements in feature engineering are being used in [industry]?” | |
Step 6: Explore Analytic Algorithms | Solution Ideation | “What algorithms are best suited for limited data in [specific use case]?” |
Trend Analysis | “What recent advances in machine learning could improve [specific outcome]?” | |
Risk Mitigation | “What are the limitations of [specific algorithm], and how can we address them?” | |
Scenario Planning | “What alternative algorithms could we explore if initial results are unsatisfactory?” | |
Step 7: Map Scores to Recommendations | Stakeholder Empathy | “How can we present predictive scores in a way that makes them actionable for [stakeholder group]?” |
Solution Ideation | “What types of decision support tools could make [specific recommendation] more impactful?” | |
Feedback and Learning | “How can we incorporate user feedback into our decision-making framework for continuous learning and improvement?” | |
Evaluation and Prioritization | “Which recommendations will have the greatest ROI and impact for [stakeholder group]?” | |
Step 8: Create Learning-Based UEX | Feedback and Learning | “What mechanisms can capture user input effectively to improve our models over time?” |
Scenario Planning | “What if users reject certain recommendations—how should the system adapt?” | |
Evaluation and Prioritization | “Which UEX elements should be prioritized to maximize user engagement with AI recommendations?” | |
Trend Analysis | “What UEX trends in [industry] could we adapt for [specific system]?” |
Table 1: TLADS Innovation Prompts
You can even ask ChatGPT to identify what additional innovation prompts you should explore. Couple the nine categories of innovation prompts with the Socratic Method and you are ready to rock and roll.
💥🎁An Offer You Can’t Refuse💥🎁
The Thinking Like a Data Scientist (TLADS) methodology is a powerful, enabling framework for maximizing the value of tools like ChatGPT, CoPilot, and Gemini. By combining TLADS with the Socratic Method and the nine categories of innovation prompts, you can uncover new opportunities, expand your ideation and discovery process, and deliver impactful results that matter.
If you’d like to explore the TLADS methodology further, DM me on LinkedIn, and I’ll send you a free PDF copy of “The Art of Thinking Like a Data Scientist” (Second Edition) eBook. This eBook details the TLADS process, includes detailed explanations of the underlying data science and design thinking concepts, and examples and templates. You can upload the eBook into your ChatGPT thread to give it a ready-made framework for guiding your GenAI-fueled exploration, learning, and discovery.
What’s the catch? None, except this: share and give back what you learn to our larger community. We are better when we collaborate, share, learn, and grow together.
Love this take! Excellent insights – thanks for sharing.