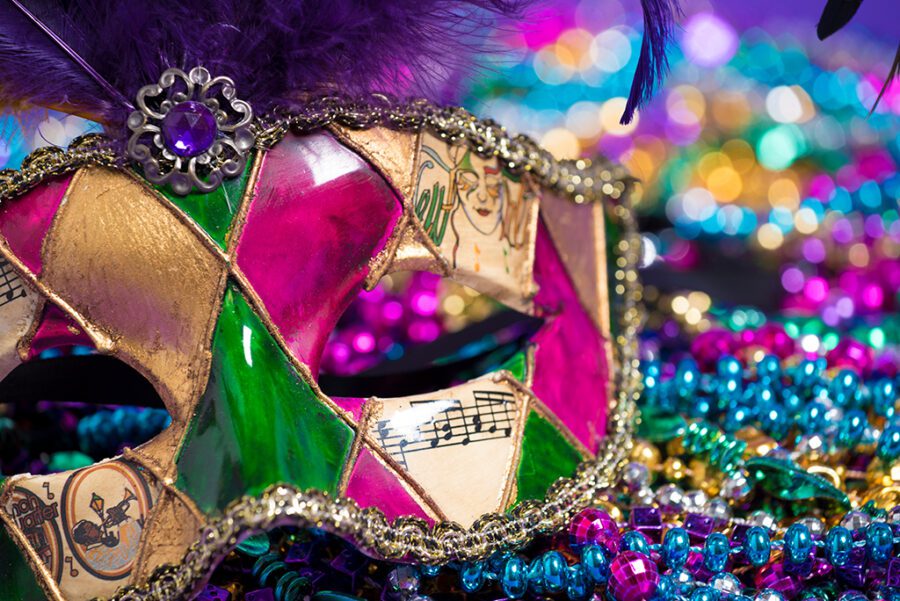
After talking to many customers recently at Dell Technologies World, I am very, very (very!) concerned how many organizations are putting their Data Strategy success into the hands of Data Meshes.
Sorry, but I think the way that IT organizations are thinking about a Data Mesh is fool’s gold.
I think the data mesh (along with other technology-lead “solutions”) distracts from the hard work that organizations must do to identify and build organizational alignment around the organization’s business and operational processes (use cases) where the application of data and analytics can derive and drive new sources of customer, product, service, and operational value. These technology-lead solutions just give folks an excuse not to do the hard work of collaborating with the business stakeholders to identify, validate, value, and prioritize the business and operational use cases where data and analytics can have a material impact.
An organization’s data strategy should NOT start with data. When you start your data strategy with data, then one is drawn to generalized technology capabilities that promise all sorts of magical outcomes without the prerequisite hard work and business stakeholder collaboration.
Instead, an organization’s data strategy should start with 1) understanding how the organization produces, manufacturers, and measures value creation, and 2) the role of data and analytics to support the organization’s value creation processes.
So, let’s dive in, and sharpen your knives. I’m ready!
Data Mesh Primer
A data mesh supports a distributed, domain-specific data structure that views “data-as-a-product,” with each domain handling their own data pipelines. The tissue connecting these domains and their associated data assets is a universal interoperability layer that applies the same syntax and data standards[1] (Figure 1).
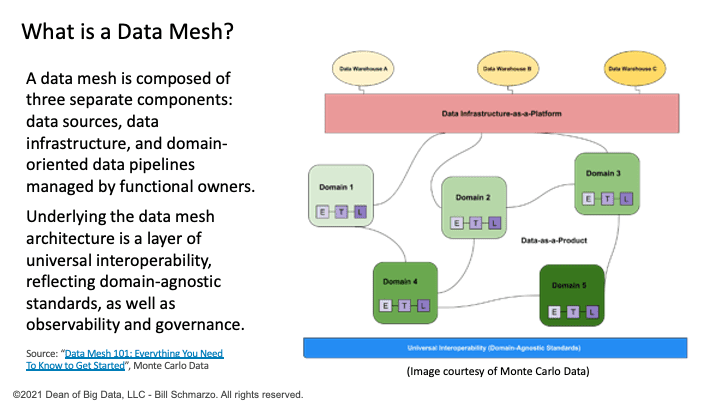
Figure 1: “What is a Data Mesh?” Courtesy of Monte Carlo Data
In the blog “Are Data Meshes Really Data Marts with Conformed Dimensions?”, I discussed how the Data Mesh sounds like a modern-day version of the work that Ralph Kimball and Margy Ross pioneered in the 1990’s creating “Business Process-centric Data Marts connected with Conformed Dimensions and the Enterprise Data Warehouse Bus Architecture” (Figure 2).
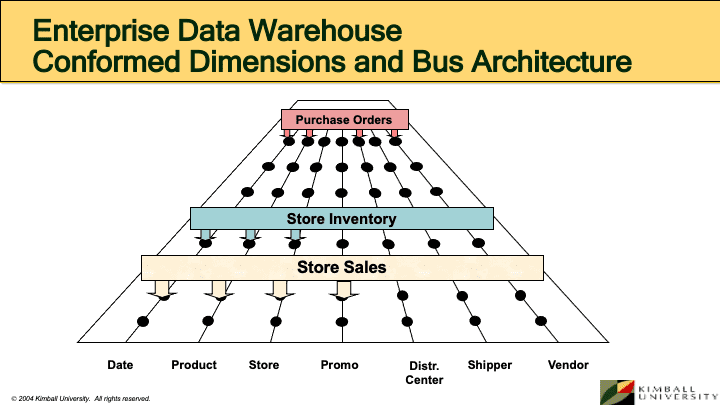
Figure 2: Conformed Dimensions as the Foundation for Agile Data Warehousing
Based upon learnings from building these conformed dimensional models, the requirements for Data Mesh success include:
- Incrementally Build Business Unit data and analytic capabilities driven by business use cases
- Connect the Business Unit data and analytic capabilities with Conformed Dimensions or Master Files
- Understand the critical importance of Master Data Management to tie together these Business Unit data and analytic capabilities
But even with these valuable lessons, one important lesson is still missing.
Data Mesh Challenge: Enabling Enterprise Data Management and Governance
If data is a corporate asset, then it needs to be managed as such. The challenge of letting each department or business unit optimize their individual use and management of data and analytics hinders the organization’s the ability to optimize the use of data and analytics across the larger enterprise mission.
I am concerned that the Data Mesh concept puts the organization’s overall data management strategy into the hands of people whose primary focus, priorities, and loyalty are to the business unit, not the enterprise. Consequently, we could end up optimizing data management and data governance execution at the Business Unit level while impairing data management and data governance at the enterprise mission level. Organizations still need an overarching enterprise data management and data governance strategy to which the business units must adhere.
For example, what happens if another department needs additional data from the sourcing department to support its operational KPIs? Let’s say our Call Center manages only the data it needs to optimize the KPIs against which it measures its success and determines performance bonuses. What happens if Marketing and Product Development need additional data elements to be captured during the call center interaction in order to optimize their KPIs and their performance bonuses? Do Marketing and Product Development “contract” with Call Center operations for the capture and management of that extra data? And what if the time and effort to capture of that additional data runs counter to optimizing the KPIs against which the Call Center is determining its success and performance bonuses (Figure 3)?
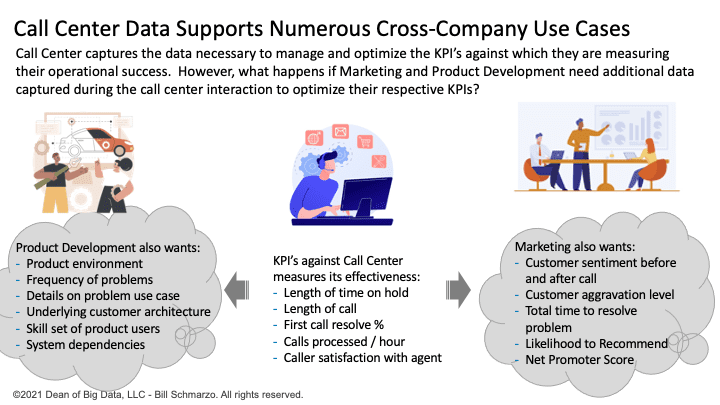
Figure 3: Departments Optimizing Performance (and Bonuses) at the Expense of the Enterprise
Another example of the importance of an enterprise view of data is the Order-to-Cash Process. Order-to-Cash is an orchestrated series of use cases or actions across departments that involve receiving and fulfilling customer requests for goods or services. The Order-to-Cash process requires departments to capture data that may not be necessary for optimizing their own operations but are critical for optimizing upstream and downstream use cases (Figure 4).
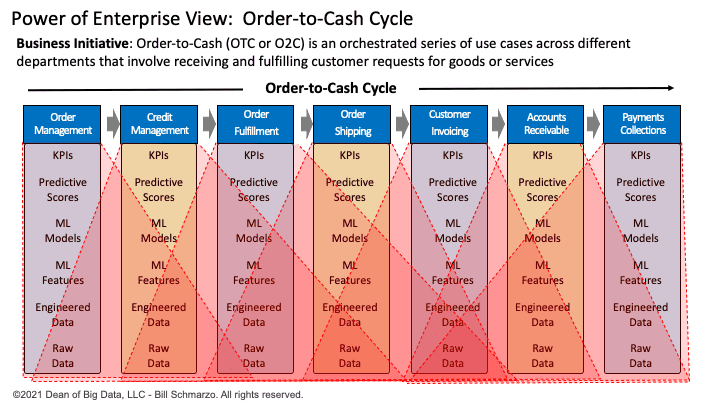
Figure 4: Power of Enterprise View of Data to Optimize Order-to-Cash Cycle
Another example is a financial services firm with multiple business units (checking & savings, credit cards, car loans, mortgages, children’s college accounts, retirement accounts, small business accounts, and wealth management), each trying to optimize the customer lifetime value for its business unit.
But what happens if the corporation wants to increase the lifetime value of its customers through an orchestrated set of enterprise-wide marketing treatments, product offers, and services? If each Business Unit is trying to optimize the customer lifetime value for its business unit, then trying to maximize the customer individual lifetime value score across the entire enterprise becomes onerous (Figure 5).
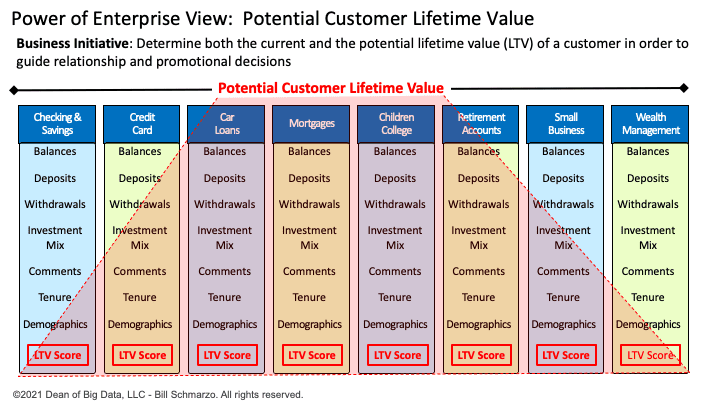
Figure 5: Enterprise Perspective – Increasing Potential Customer Lifetime Value
An organization’s Data strategy must start with an enterprise view of data management and value creation. I mean, what’s the use of having a data strategy that optimizes each business unit while the whole enterprise withers away?
Is Data Mesh Fool’s Gold? No, but it’s Only Part of Your Data Strategy
The Data Mesh is more of a data management and governance approach than it is a data and analytics architecture. And the data mesh, much like the “Business Process-centric Data Marts connected with Conformed Dimensions and the Enterprise Data Warehouse Bus Architecture” that came before it, can provide the foundation for business-centric data strategy as long as one does not lose sight of the over-arching enterprise data, analytics, and value creation needs of the enterprise.
The key to data mesh success? Create a business-driven data strategy (versus a data-driven data strategy) that addresses the following concerns:
- Concern #1: Data-driven Data Strategy is a Field of Dreams Approach. Loading a data repository with all the organization’s data is more likely to draw crickets than rave reviews. Don’t build the data repository and hope that your business stakeholders can find value from the data. Hope is only a business strategy if you’re in the cosmetics industry.
- Concern #2: Don’t start your Data Strategy with Data. Start your data strategy by understanding how your organization produces and measures value creation. This provides the guidelines for determining where and how to apply data and analytics to optimize and/or accelerate the organization’s value creation capabilities.
- Concern #3: Data-driven isn’t an End, It’s a Means. Just like “360 degrees of customer”, we sometimes confuse means with ends. The end is using data and analytics to derive new sources of customer, product, service, and operational value. The desired end is becoming value-driven. Heck, I’m not even sure what it means to be “data-driven, which is probably why we are seeing organizations drastically fail in achieving that means (see Figure 6).
- Concern #4: Embrace an Enterprise View of Data-driven Value Creation. You don’t want to optimize the performance of each business unit, while the overall organization goes bankrupt. The data management, analytics development, and value creation needs of the enterprise must be served. And if there are business conflicts between the enterprise and the individual business units, do NOT expect that your data management will magically solve that cultural and business problem.
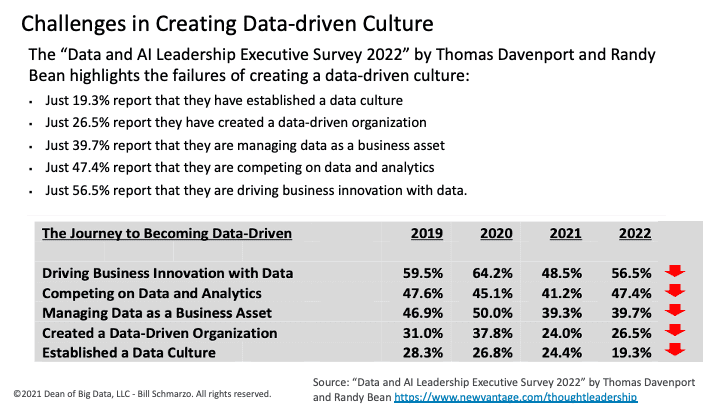
Figure 6: The Data-driven Culture Failure
So, I guess I wasn’t so hard on the data mesh after all. We just need to remember to not let technology-lead “solutions” distract us from the hard work to identify and build organizational alignment around the organization’s business and operational processes (use cases) where the application of data and analytics can derive and drive new sources of customer, product, service, and operational value.
[1] Source: “Data Mesh 101: Everything You Need To Know to Get Started”, Monte Carlo Data