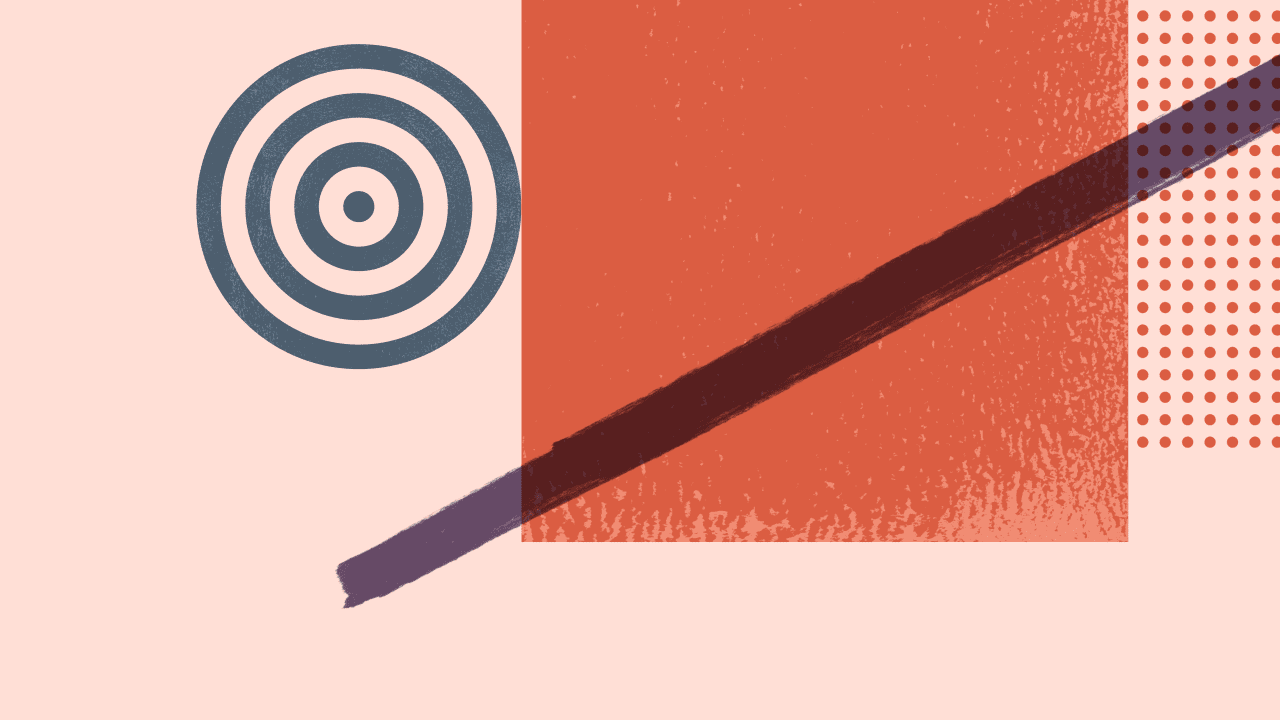
In the data-driven world of modern business, the quality of data flowing through your pipelines is just as critical as the data itself. High-quality data is the lifeblood of insightful analytics and informed decision-making. However, ensuring this level of quality within a data pipeline presents a complex challenge, often overlooked in the rush to harness big data’s potential. Data quality is not just about having accurate data; it encompasses completeness, consistency, reliability, and timeliness – all crucial for deriving meaningful insights. This article offers an in-depth guide to improving data quality throughout your pipeline. We will delve into where strategies and best practices are designed to ensure that your data is abundant and of high quality but also pristine, accurate, and ultimately reliable for making critical business decisions. Navigating the nuances of data quality can transform your data pipeline into a powerful asset for your organization.
Understanding data quality in the context of a data pipeline
Data quality within a data pipeline is a multifaceted concept beyond mere accuracy. It involves ensuring that data is complete, consistent, reliable, and timely as it moves through various collection, processing, and analysis stages. Quality is crucial in a data pipeline because it directly impacts the validity of business insights derived from the data. Poor data quality can cause wrong decisions, slow work, and missed chances. For instance, complete data sets may result in biased analytics, while consistent data can cause confusion and mistrust among users. Furthermore, the speed at which data is processed and made available for decision-making, referred to as timeliness, is essential in fast-paced business environments where real-time data is increasingly becoming the norm. Understanding these dimensions of data quality is the first step in ensuring that your data pipeline is not just a conduit for data but a reliable source of actionable business intelligence.
Assessing your current data quality
Before enhancing the data quality in your pipeline, it’s crucial to assess its current state. Begin by examining the data for accuracy, completeness, and consistency. Utilize tools to analyze data patterns, identify anomalies, and flag data deviating from established norms. This assessment should also review how current data management practices impact data quality, including data entry processes and automated data collection methods. Identifying the primary sources of poor data quality – be it through human error, system glitches, or inadequate data processing methods – is essential. This initial evaluation forms the foundation for targeted improvement strategies, ensuring a focused approach to enhancing data quality.
Implementing data cleansing techniques
Data cleansing is a critical step in improving data quality within your pipeline. This process involves identifying and rectifying data inaccuracies, inconsistencies, and redundancies. Start by removing duplicate entries to avoid skewed analysis. Address inaccuracies by cross-verifying data against reliable sources and correcting any errors found. Standardize data formats to ensure consistency across the dataset. For instance, unify date formats, categorize similar data types, and align disparate data sets. Employ feasible automation tools to streamline this process, ensuring continuous and efficient data cleansing. Regularly implementing these techniques is critical to maintaining the integrity and reliability of data throughout its lifecycle in the pipeline.
Data validation and verification strategies
Implementing robust validation and verification strategies is essential to ensure data integrity in your pipeline. Data validation involves checking incoming data against predefined rules and criteria to ensure it meets quality standards. This could include verifying data formats, ensuring mandatory fields are not empty, and checking for logical consistency in data sets. Verification is cross-referencing data with external authoritative sources or historical data to confirm accuracy. Automating these processes can significantly enhance efficiency. Regularly applying these strategies as data flows through the pipeline ensures that only valid, accurate data is used for decision-making and analysis.
Regular data quality audits
Conducting regular data quality audits is crucial for maintaining the integrity of your data pipeline. These audits entail a detailed examination of the data within your system to ensure it adheres to quality standards and business requirements. Set periodic intervals for these audits to examine the data’s accuracy, completeness, and consistency. Use a mix of manual checks and automated tools for a thorough evaluation. Audits help identify emerging issues, such as new types of data errors or gradual changes in data quality. The knowledge acquired from these audits empowers you to make well-informed modifications to your data processing methods, continually enhancing the overall quality of data in your pipeline.
Leveraging automation for continuous data quality improvement
Automating data quality processes is critical for continuous improvement. Utilize software that routinely scans, cleans, and validates data, identifying and rectifying real-time issues. This increases efficiency and ensures consistent data quality standards are maintained throughout the data pipeline, reducing the risk of human error.
Best practices for ongoing data quality management
For effective data quality management, establish a continuous monitoring and improvement culture. Regularly update and refine data quality metrics and standards—train staff on data accuracy and consistency. Encourage proactive reporting of data issues and foster collaboration between teams to maintain high data quality standards throughout the pipeline.
Final words
Enhancing data quality in your pipeline is not a one-time effort but an ongoing commitment. By assessing the current data quality, implementing cleansing techniques, validating and verifying data, conducting regular audits, and leveraging automation, you can significantly improve the reliability and usefulness of your data. Adopting best practices for data quality management ensures that these efforts are ingrained in your organization’s culture. High-quality data is a valuable asset in today’s data-driven world, and investing in these processes will pay dividends in the form of more accurate insights, better decision-making, and, ultimately, a more decisive competitive edge in your business operations.