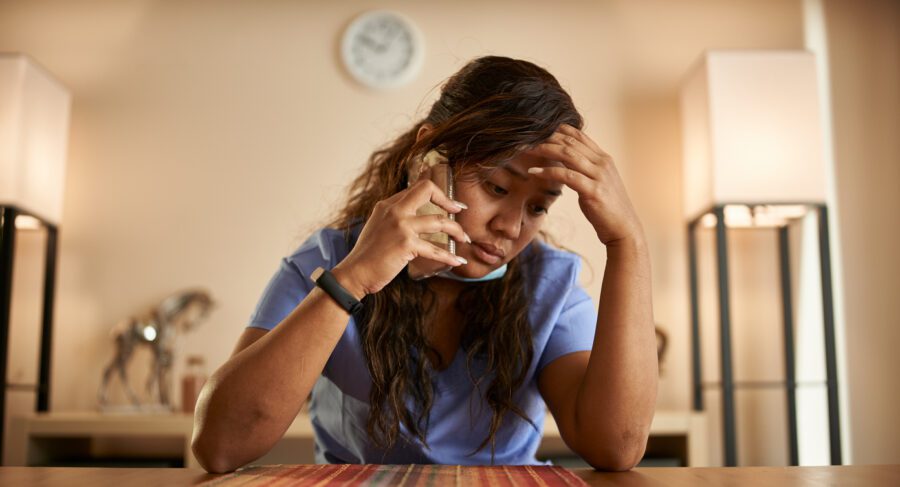
Medical identity theft occurs when someone fraudulently uses another person’s information to obtain medical services or goods. This type of theft can lead to significant financial loss, incorrect medical records, and compromised patient safety.
Combating medical identity theft is crucial in the healthcare industry to protect patient data, ensure accurate medical records, and prevent financial losses for patients and providers. Machine learning (ML) is vital in this fight, as it leverages advanced algorithms to detect unusual patterns, predict potential fraud and monitor real-time activities. It enhances the industry’s ability to prevent and detect medical identity theft.
What is medical identity theft?
Medical identity theft involves the fraudulent use of someone’s personal and insurance information to obtain medical services, prescription drugs, or medical devices. For instance, an individual might use another person’s insurance details to receive costly treatments. This scenario leaves the victim with inaccurate medical records and unexpected bills. Healthcare providers also suffer from increased operational costs and potential legal issues.
Between the third quarter of 2023 and the second quarter of 2024, the Federal Trade Commission received over 10,000 reports of medical identity theft, highlighting its prevalence. The expense of these crimes can reach billions annually, emphasizing the urgent need for robust preventive measures in the healthcare sector.
1. Anomaly detection in medical billing
ML algorithms can identify unusual billing patterns by analyzing vast amounts of billing data and detecting anomalies that deviate from established norms. These algorithms use historical data to learn typical billing behaviors and flag irregular transactions. These include duplicate charges or services that do not match a patient’s medical history.
Continuously learning and adapting allows ML systems to improve accuracy over time, making them highly effective in identifying potential fraud. Implementing AI — including ML — in billing processes could reduce error costs by 8%, translating to savings of $96 million. It demonstrates the financial and operational benefits of adopting advanced technology in healthcare billing.
2. Real-time fraud detection systems
Implementing ML models for real-time monitoring and alert systems enables healthcare providers to detect and respond to suspicious activities instantly. These models continuously analyze real-time data from sources like patient records and billing information to identify patterns and anomalies indicative of fraud.
When the system detects irregular activities — such as unexpected changes in patient information or unusual billing claims — it immediately generates alerts for further investigation. This proactive approach helps in the early detection and prevention of medical identity theft and enhances healthcare systems’ overall security and integrity.
3. Patient behavior analysis
Using ML to monitor and analyze patient behavior and usage patterns allows healthcare providers to detect inconsistencies indicating fraudulent activities. ML algorithms can track normal patient behaviors and usage trends — such as appointment frequencies and treatment types — and identify deviations suggesting potential identity theft.
Accurately distinguishing between legitimate and suspicious activities allows ML to help in early fraud detection and reduce the strain on financial and labor resources. Healthcare fraud drains these resources by diverting experienced staff from more pressing and critical tasks. Implementing ML enhances security and ensures valuable medical personnel can focus on delivering quality care rather than being bogged down by fraudulent distractions.
4. Predictive analytics for risk assessment
Utilizing ML to assess the risk of medical identity theft for individual patients involves analyzing data points such as demographic information, medical history, and behavioral patterns to create a risk profile for each patient. ML algorithms can identify high-risk patients by detecting anomalies and patterns suggesting potential identity theft, allowing healthcare providers to implement additional security measures.
This targeted approach prevents unauthorized access and misuse of personal health information, which is critical given the severe consequences of data breaches. For example, the disclosure of personal health information can result in a $50,000 fine and up to a year in prison. By leveraging ML, healthcare providers can better safeguard patient data and mitigate the risks of medical identity theft.
5. Natural language processing for document verification
Using natural language processing (NLP) to verify the authenticity of medical documents and records allows healthcare providers to detect inconsistencies and potential fraud. NLP algorithms can analyze the text within medical documents, comparing it to known patterns and standards to identify anomalies such as forged signatures, altered data, or fabricated information.
This technology can cross-reference information across various sources to ensure consistent and legitimate records. By automating the verification process, NLP enhances accuracy and reduces the time and effort to audit documents manually. This approach maintains the integrity of medical records, protects patient information, and ensures compliance with regulatory standards.
Challenges and considerations
Data privacy and security concerns are paramount in the healthcare industry, especially given that hackers breached over 51 million data records in the U.S. in 2022. Integrating ML systems with existing IT infrastructure presents opportunities and challenges. While ML can enhance security measures and fraud detection capabilities, it also requires robust data protection protocols to prevent unauthorized access.
Additionally, the successful implementation of ML systems depends on their seamless integration with current IT setups, necessitating careful planning and coordination. Continuous monitoring and updating of ML models maintain their effectiveness, as these models need to adapt to evolving threats and new patterns of fraudulent activity.
Machine learning’s future in medical identity theft
The future of machine learning in combating medical identity theft looks promising, with advancements continuously enhancing detection accuracy and fraud prevention capabilities. Health care providers must embrace ML technologies to protect patient data, reduce financial losses, and ensure the integrity of their systems.