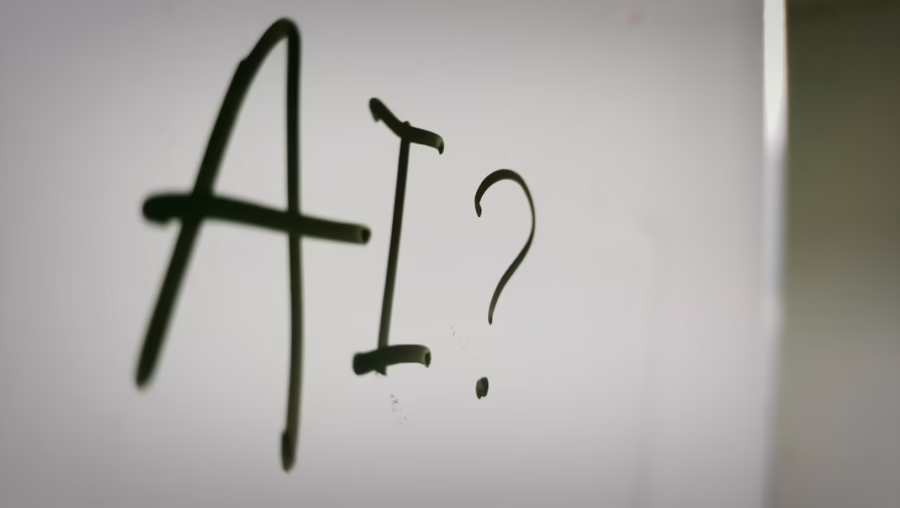
Software development cycles accelerate constantly, pushing quality assurance teams to keep pace. However, the pressure engineering leaders face to ensure quality under the speed and complexity modern pipelines require is also immense. And simply doing more of the same old way of things isn’t enough with the advancing user demands.
Interestingly, while much of the focus has been on accelerating coding or transforming creative workflows, GenAI is profoundly reshaping quality assurance. Because it’s not just augmenting existing tools; it’s fundamentally altering how quality is approached.
As teams strive for faster release cycles without compromising stability, traditional QA strategies are being challenged and augmented in ways that were previously unimaginable, says Mayank Bhola, co-founder and head of the product at LambdaTest, a scalable test execution platform. “This isn’t merely about automation; it’s a fundamental shift in how quality is approached, managed, and executed throughout the development lifecycle.”
From his vantage point, leading initiatives like Kane AI, a native GenAI test agent by his team at LambdaTest, Bhola observes that Gen AI holds the promise of breaking down long-standing bottlenecks, improving test coverage, and freeing up valuable human capital for more strategic endeavors. “It’s pushing the QA function beyond its historical boundaries, enabling new capabilities and fostering greater collaboration across teams,” he affirms.
To understand these shifts, I turned to industry leaders for practical insights on how generative AI is reshaping traditional QA strategies.
Automating the foundational tasks
One of the most immediate and impactful areas where Gen AI is reshaping QA is in automating fundamental, often time-consuming tasks. Creating test cases and managing test data have historically been significant bottlenecks that otherwise require meticulous manual effort. Generative AI is changing this equation. Bhola notes that AI is primarily being used “mostly helping developers build the test case generations. And second, generating the test data.” Generating test data is a critical challenge for developers who might not know what production data looks like, and Gen AI helps overcome this by creating data sets required for testing scenarios.
AI is accelerating processes for test case generation. It generates several test scenarios faster, creating more accurate, detailed, and comprehensive tests from sources like user stories in tracking systems. But it also isn’t just about speed. Michael Kwok, Vice President, IBM watsonx Code Assistant, and Canada Lab Director, tells TechTarget how at IBM, Gen AI has automated test case creation for complex applications, not only “drastically reducing the time required” but also significantly “increasing test coverage.
Specialized AI tools are pushing these boundaries further, particularly in areas like unit testing. An AI approach combining generative AI with reinforcement learning has shown impressive results. For example, benchmarking indicates that these tools can generate significantly more unit tests and achieve substantially higher code coverage compared to general-purpose coding assistants. Not only that, but these specialized tools often produce tests with a very high success rate for compiling and passing on the first attempt. And customers are seeing real-world impact, with some reporting the ability to complete extensive unit test writing rapidly, saving considerable manual effort over large codebases. This is helping teams handle the sheer volume of testing needed in modern development.
Beyond initial creation, maintaining test assets is another area benefiting from AI. Bhola highlights that Gen AI helps with the maintenance of existing test environments and test cases, making it easier for new team members to understand what’s already covered. Or basic data creation and script-based automation maintenance can become tasks that humans spend less time on. Gustavo Daniel Pozzi, Project Manager at BairesDev, adds that Gen AI-powered tools can even help test scripts automatically adapt to minor UI changes, dramatically reducing maintenance effort. Leveraging AI for these foundational tasks frees up valuable human time, allowing quality professionals to focus on more strategic activities. But how is Gen AI impacting the actual process of finding and managing defects?
Enhancing defect detection and management
Generative AI is also proving valuable in the downstream activities of the QA process, specifically in detecting issues and streamlining their resolution. Kwok also shares that (at IBM) Gen AI has “enhanced our defect detection capabilities, spotting issues QA engineers could not find easily.” AI can augment human skills, uncovering subtle or complex bugs that might evade traditional or manual methods.”
Not only can AI help find bugs, but it can also significantly speed up the process of reporting and triaging them. Pozzi remarks how AI can “generate a bug report faster, more detailed, and with a standard format,” pointing to tools like the “Ask AI” feature in Chrome DevTools as an example of this capability. This standardization and detail can make the subsequent steps of analysis and fixing much more efficient.
Bhola provides a tangible impact here, stating that using AI-generated test cases and data “reduces the bug trig and bug troubleshooting processes up to 15% or 20% in some cases.” This saved time means teams can deploy resources elsewhere, potentially on developing new features instead of lengthy debugging sessions. Or manual bug triaging is one of the traditional tasks that is becoming less relevant, as the automation and detailed reporting provided by AI streamline this phase significantly.
These advancements in detection and reporting allow teams to address quality issues more rapidly and effectively. And they also hint at a broader shift in who can participate in the testing process itself.
Democratizing testing & shifting left
Generative AI is also breaking down silos and enabling individuals beyond the dedicated QA team to contribute to quality directly. Pozzi notes that “GenAI is democratizing testing activities across organizations, allowing non-QA individuals to participate in ways that were previously impossible.”
But who is getting involved? Experts point to roles like developers, product managers, and business analysts. Gen AI tools are making it feasible for them to create and execute tests without needing deep coding expertise. Pozzi highlights the product managers and business analysts who “can create functional tests by describing scenarios in natural language or Gherkin,” which AI then translates into executable scripts. This ensures tests align with business expectations from the outset.
And specialized AI tools for unit testing also support a “shift left” by integrating directly into the developer’s workflow. Andy Piper, VP of Engineering at Diffblue, explains that rather than QA testers writing unit tests for someone else’s code, specialized AI agents can be used as a plug-in so developers generate these tests as new code is written. This puts the test creation in the hands of the person who understands the code best.
This trend towards involving non-QA personnel fosters more cross-functional teams. Stakeholders get involved earlier, building a shared responsibility for quality across the development lifecycle. According to Kwok, this shift “has fostered a more collaborative approach to testing, involving multiple stakeholders. We’ve observed improved communication and a more comprehensive understanding of the software application across the team.”
While the potential for non-technical roles like Product and Support personnel to act as “citizen testers” by creating tests in natural language exists, Marcus Merrell, Principal Technical Advisor at Sauce Labs, points out that this is currently “bigger on promise than on reality.” But the direction is clear: quality is becoming a team sport, with AI as the enabler. This democratization goes beyond simple efficiency gains, pointing towards entirely new ways of ensuring quality, and it means QA professionals are evolving, moving into roles as facilitators and educators, helping others leverage these new tools and defining the overall testing strategy.
Unlocking new strategic capabilities
Gen AI is introducing strategic advantages and testing capabilities that weren’t readily feasible before, moving QA beyond just checking boxes faster. Kwok in our email interview highlighted the ability to perform exploratory testing at scale. Gen AI-powered tools can simulate real-world user behavior, helping teams “identify issues that traditional testing methods might miss.” It also supports continuous testing by executing tests throughout the development cycle, providing real-time feedback and identifying code paths “which may have been missed before, significantly enhancing test coverage.”
Gen AI brings enhanced context awareness into testing, which is a new level of strategic capability. Bhola explains that AI models are more aware of the context in which tests are running. Based on that context, they can generate more relevant data, scripts, and validations. This context awareness extends to understanding different domains and languages. He points out that AI tools “can also encapsulate the testing. For multilingual and domain-specific use cases,” making it easier to ensure quality across global applications. And this awareness can also be applied to enforce specific rules and regulations. He also sees Gen AI assisting in writing “real-time, risk-based test cases or real-time, compliance-based test cases,” something previously very difficult unless the QA was manually aware of every specific regional or financial regulation.
Pozzi underscores another strategic capability enabled by AI: risk-based regression testing. By analyzing code changes, user behavior patterns, and historical bug data, AI helps eliminate wasteful testing of unaffected functionality while ensuring critical paths receive appropriate coverage. Beyond purely testing activities like risk-based regression, some see Gen AI as a powerful tool for simply helping people get their jobs done when they’re stuck. Merrell suggests the most profound advantage isn’t always a specific QA task but how AI helps people get unstuck from any number of situations,” acting more like a helpful assistant to overcome technical or planning roadblocks in the testing workflow.
Leveraging these new capabilities allows QA teams to become more proactive, strategic, and integrated into the broader development goals. But with these new capabilities come changes in what skills and practices are needed.
Which traditional practices become less relevant?
There’s a strong consensus among experts that purely manual, repetitive, and predictable tasks are on the decline. Kwok states that traditional practices such as “manual test case creation, test data management, and bug triage will become obsolete.” Similarly, Bhola points to “manually exploring the scenarios, manually writing the test cases, and manual bug triaging scenarios” as traditional ways that will likely be obsolete once companies adopt AI testing.
Pozzi reinforces this view, listing “Repetitive and predictable tasks” like manual test case generation, repetitive regression testing, and basic test data creation as expected to become less relevant. He includes detailed test documentation, maintenance, and script-based automation maintenance in this category. These tasks, often seen as tedious or toil, are prime candidates for AI automation.
Piper asserts, “Extremely time-intensive, tedious tasks like unit testing will become obsolete” for human developers, arguing that developers thrive on innovative code, not the drudgery of writing, debugging, and maintaining tests.
But it’s important to distinguish between practices and tasks. Merrell offers a crucial perspective here. He doesn’t believe the core practices of QA – the craft learned over the years aimed at reducing risk and increasing user happiness – will become obsolete. “Anyone who says otherwise is simply trying to cut costs,” he argues. Instead, he sees specific tasks going away. He lists tasks that should be obviated by Gen AI, including “Gathering data for reports, justifying project budgets, filing bugs, measuring test coverage, toiling with test scripts, configuring platform matrices.”
And the shift isn’t about replacing the QA professional’s critical thinking or strategic role, but about automating the busywork. Traditional static approaches, like predefined regression pipelines that run on a fixed schedule, may also become obsolete. Bhola suggests these static pipelines will be replaced by dynamic testing based on load and traffic, while Kwok sees Gen AI driving a broader shift away from methodologies like waterfall towards more agile and continuous testing. The message is clear: QA professionals need to pivot away from tasks AI can handle and focus on the higher-value, strategic aspects of their role. But this transition isn’t without its difficulties.
The challenges and necessary adaptations
While Gen AI offers tremendous potential to automate and enhance QA processes, its integration, particularly in the development workflow, also introduces new challenges. David Smooke, Founder & CEO at HackerNoon, offers a cautionary view on this, arguing that “generative AI is better at generating something from nothing than it is at moving something that’s almost ready in production.” This disparity creates work downstream.
According to Smooke, Quality assurance is now “absorbing a ton of extra pressure to patch together, clean up and prevent explosions from the boom of AI-generated code by their colleagues.” He highlights issues with developers’ “vibe coding,” where they prompt the AI agent to fix errors instead of simply rolling back to a previous, working version. And even if AI-generated code appears to work in a test environment, he asks, “Who knows what could happen when it goes live?” If the human developer doesn’t understand why the AI’s code isn’t working locally, then it becomes incredibly difficult for a colleague, including QA, to understand how to fix it in production.
Ultimately, this means QA professionals must adapt their stance. Smooke believes that for QA professionals to level up in the age of generative AI, “they need to more sternly draw the lines of what is passable and what is not.” They need to maintain robust quality gates and criteria for accepting code, regardless of how it was generated.
And while the potential for AI to fundamentally change core QA processes like test case or test data generation is significant, Merrell notes that concrete, quantifiable examples of this scale of change aren’t yet universally apparent across the industry. Navigating these challenges requires QA teams to be vigilant, set clear standards, and evaluate AI tools critically. But these challenges also point towards a fundamental shift in the identity and responsibilities of the QA professional itself.
The evolving role of the QA professional
With Gen AI handling more of the repetitive and predictable tasks, the human element of QA is pushed towards higher-order activities. Pozzi describes this evolution, stating that the QA role is moving away from traditional manual or automation specialists into “AI-augmented testing strategists.” This means delegating routine testing tasks to AI systems so QA engineers can focus on higher-value activities like risk assessment, complex scenario design, and strategic quality planning that leverage uniquely human critical thinking and domain expertise.
Kwok agrees, noting that automating manual tasks allows “QA engineers to focus on higher-value activities like test strategy and exploratory testing.” It isn’t about making the QA role obsolete; it’s about elevating it. The core craft of QA – understanding user needs, identifying risks, and ensuring a quality product – remains vital, Merrell emphasizes. The shift is simply freeing QAs from the “toil” of tasks AI can handle.
As testing becomes more democratized and integrated into the development workflow, the QA professional’s role also expands to that of an enabler and educator. Pozzi sees QAs evolving into “facilitators, training non-QA team members on AI tools and defining testing frameworks.” They become the experts guiding others in leveraging AI effectively for quality.
And in the face of potential issues introduced by AI-generated code, Smooke highlights the crucial need for QAs to maintain their role as the arbiters of quality, advocating for stricter adherence to standards and clearly defining what meets the bar for production readiness.
Bhola believes these shifts mean the QA professional is becoming less of a gatekeeper performing manual checks and more of a strategic advisor, a skilled collaborator, and a critical evaluator, ensuring that AI is used effectively to enhance, not compromise, overall software quality.
So, what does all this mean for QA?
The impact of generative AI stretches well beyond simply doing the old things faster. It is enabling entirely new strategic capabilities, facilitating scaled exploratory testing, supporting continuous feedback loops, and bringing unprecedented context awareness, risk assessment, and compliance checking into our test suites. And it’s also breaking down traditional barriers, inviting developers, product managers, and others to participate more directly in ensuring quality earlier in the development cycle.
Of course, this shift isn’t without its complexities. Dealing with AI-generated code introduces new pressures and requires QA professionals to sharpen their skills in critical evaluation and maintain rigorous quality standards. And not every promised capability is a universal reality yet.
But the overall trajectory is clear. The QA professional’s role is evolving, moving away from being defined by manual execution or even script maintenance towards becoming strategic navigators, skilled enablers of quality practices across the team, and expert evaluators of increasingly sophisticated systems. For teams looking to thrive in the future of software development, understanding and strategically adopting Gen AI into their quality processes isn’t just an option; it’s now essential.