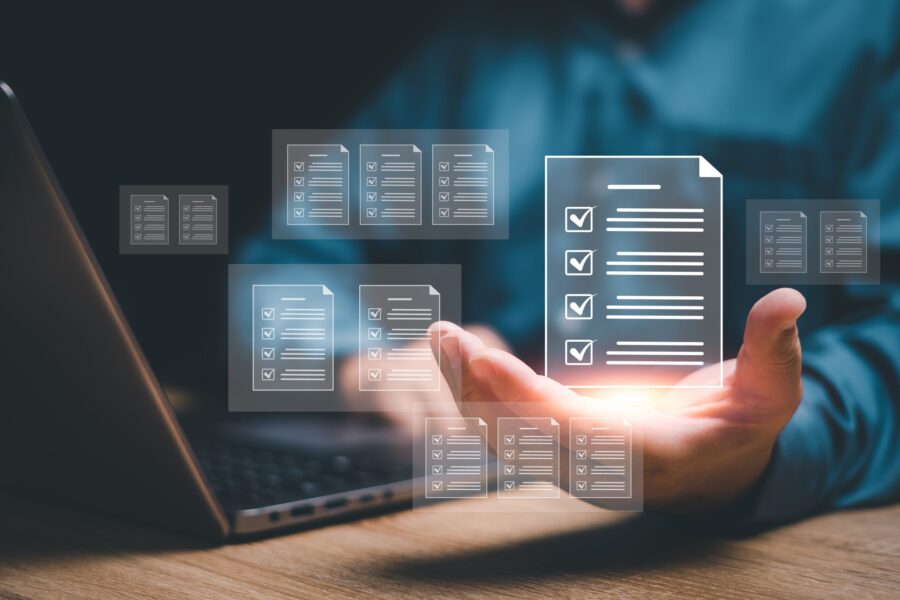
Data Engineers:
We look into Data Engineering, which combines three core practices around Data Management, Software Engineering, and I&O. This focuses primarily on reconstituting data into usable consumer forms by building and operationalizing data pipelines across various data and analytics platforms.
Data Engineers, aka producers of the data, must be robust across data modeling, pipeline development, and software engineering and ensure that data always is reliable and made available per business service level agreement. This commitment requires executing a variety of checks across a large amount of data at speed and preventing any insufficient data from reaching downstream.
Due to the scale of data, this engineer-focused validation is wide and shallow and includes validation at the surface or metadata levels of data. These verification methods spread from API checks preventing issues before data lands (Shift Left) to detecting problems at the warehouse or lake house using anomaly monitoring (Shift Right). Both of these approaches revolve around metadata or custom-level SQL checks using observability to prevent or detect unknown issues before consumers use the data or meet agreed-upon Data Contracts/ SLAs with consumers/internal/external stakeholders.
Data Scientists or Data Analysts:
While in the world of Data Scientists or Data Analysts aka consumers of the data, the most common statement one would hear is “Garbage in, garbage out”. In other words, the model or report developed is only as good as the data that is being fed in. Here the data quality assessments are defined primarily by the use case and vary for each model and report generated.
The data quality checks performed are more business-centric and have to be measured continuously to manage, and resolve any known issues using the data context. Primarily, the consumers of the data or downstream applications want to ensure that the data they use for building reports and analytical models are business fit.
This requires performing narrow and deep business checks with aggregate analysis, deterministic rules, statistical measures, data accuracy, integrity, custom checks, and other dimensions of data quality that are necessarily not the focus of upstream or producers of data.
E.g., a data scientist building the next big model for optimizing route planning for the distribution of materials produced may be interested in certain characteristics of the data that may not be critical for a marketing function that is focused on targeting the younger generation demographic for new revenue.
This evaluation and need for data quality change from time to time and also dictates the need for decentralized data ownership or evaluation of data quality in their context.
Data Stewards:
Now, let’s not forget about the Data Stewards. This role is very confusing in the world of the modern data landscape and is even turned more confusing with decades of broken promises from Data Governance platforms. The word “Stewardship” means “supervising or taking care of something” and in this case, yes even in decentralized data ownership, there are a set of business terms or critical data elements (business terms) that needs to be maintained consistently to maintain business integrity e.g., Bank Routing Number, SSN, Customer ID.
This requires consistent data quality checks applied across the organization’s varying data assets based on business context (semantics) irrespective of where and how it is defined in the technical metadata. These checks require the automated discovery of semantics and a consistent measure of quality checks is a must and a need for “Agile” Data Stewardship or Federated teams.
Data Leaders:
Last but not least, Data Leaders aka Business Stakeholders are focused on what could make their next big win and want to understand how they could use the data on hand to enable new or improvised strategies toward positive business outcomes. A Gartner study shows that by 2025, 80% of data and analytics governance initiatives shall focus on business, and through 2024, 50% of organizations will adopt modern DQ solutions to better support their digital business initiatives.
This clearly shows how business leaders are focused on identifying key business processes, their KPIs and KRIs, and underlying data or metric assets that have a direct linkage to organizational mission-critical priorities. Having baselined values of business KPIs/KRIs before starting DQ initiatives and measuring continuously shall help to have the leaders a top-to-bottom-down view and more importantly which domains, and/or applications need to improve data quality. This view of “Top-down” business quality checks is essential for assessing key performance indicators, and benchmark measures for data quality against any initiative’s outcomes. Also, Privacy, Security, and Compliance Leaders need at a minimum continuous validation and assurance depending on the classification or type of data to ensure 100% regulatory compliance.
Get Started today with DQLabs and explore our platform!