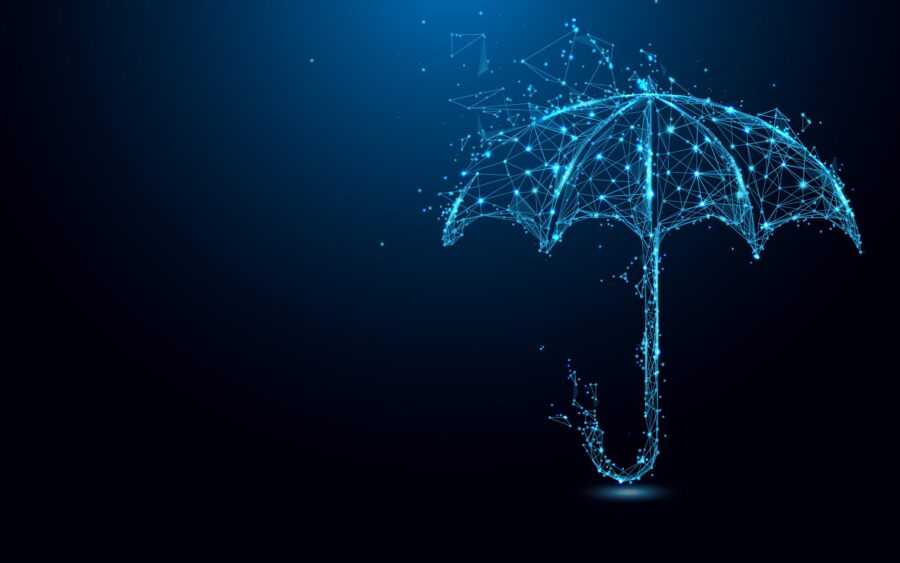
In the era of big data and AI, harnessing weather data to predict, plan, and optimize various industries has become an indispensable practice. Today, we will delve into the fascinating process of turning this voluminous weather data into actionable insights. By combining cutting-edge technology, analytical models, and industrial applications, we’ll explore how weather data can be converted into valuable strategies for business and life.
Gathering weather data: A tech-filled task
The first step to creating actionable insights from weather data is gathering the data itself. There’s a myriad of tools that meteorologists and data scientists utilize for this purpose. Doppler radars, for instance, are used to measure precipitation movement, intensity, and the velocity of droplets in the air.
Weather satellites orbiting the Earth provide essential data for a global overview of the atmosphere, tracking weather systems, and measuring cloud properties. Weather balloons or radiosondes are launched twice daily across the globe, yielding crucial information about the upper atmosphere, including temperature, humidity, and pressure (source: NOAA).
The science of transforming weather data into actionable insights
Once the data is collected, it needs to be cleaned, processed, and interpreted. This is where data science comes into play. Companies like Tomorrow.io leverage Machine Learning (ML) algorithms to interpret and predict weather patterns. Recently, the company boosted their data gathering capabilities by launching their own satellite (source: Geospatial World).
Tomorrow.io, a leader in weather data collection, approaches weather data with a rigorous multi-step process that leverages the latest technologies in data ingestion, analysis, and interpretation.
- Data ingestion: Data ingestion is the first crucial step, where data from multiple sources are collected. One significant source is their satellite, which orbits the Earth, recording various atmospheric parameters. This data is complemented with information from ground weather stations, other satellites, weather balloons, and buoys. By doing so, they ensure a broad coverage of data, capturing the dynamic complexities of the weather.
- Data cleaning & preprocessing: Once ingested, the raw data undergoes an essential cleaning and preprocessing stage. This involves checking for missing values, dealing with outliers, normalizing different data scales, and resolving discrepancies between different data sources. The goal here is to create a coherent, consistent dataset that is ready for further analysis.
- Data structuring: The clean data is then structured and stored in a format that enables efficient access and computation. Given the massive volume and velocity of weather data, Tomorrow.io employs distributed storage and big data technologies to handle this efficiently.
- Data analysis & interpretation: This is the most critical phase where the real magic happens. Tomorrow.io uses a combination of traditional statistical methods and advanced Machine Learning (ML) techniques to analyze and interpret the data. Statistical methods help in understanding the relationships between different weather parameters and identifying patterns and trends in the data.
The ML component takes this a step further by learning from historical data to predict future weather patterns. These ML models employ complex algorithms that can capture non-linear relationships and subtle patterns in the data, often beyond human comprehension. Models are continuously trained and updated as new data flows in, thereby improving their predictive accuracy over time. - Generation of actionable insights: The output from the data analysis and ML models is then transformed into high-resolution, hyperlocal weather forecasts. These aren’t just generic forecasts but tailored insights that cater to specific geographical locations and timeframes. The result is reliable, actionable weather information that users can apply in their decision-making process, whether they’re planning their daily commute or strategizing their crop planting schedule.
Source (tomorrow.io)
In essence, the Tomorrow.io approach demonstrates the immense potential of data science in converting raw, seemingly chaotic weather data into reliable, precise, and actionable insights. As technology advances and the field of data science matures, we can expect even more accuracy and granularity in our weather forecasts.
The far-reaching value of weather data
The practical applications of these insights from weather data are wide-ranging and influence many sectors.
- Agriculture: Farmers can optimize their sowing, irrigation, and harvesting schedules based on accurate weather forecasts.
- Aviation: Airlines can plan their flight schedules and routes more efficiently by anticipating weather disruptions.
- Energy: The energy sector can accurately forecast the availability of wind, solar, or hydropower, enhancing their production efficiency and reducing wastage.
- Retail: Retail businesses can optimize their inventories based on weather predictions. Accurate weather data can help businesses forecast consumer behavior and align their offerings accordingly.
By harnessing weather data effectively, businesses can significantly improve their operational efficiency, reduce costs, and boost revenue.
The future of weather data
With advances in technology, the future of weather data looks promising. Improved satellite technology, better data collection methods, and more sophisticated machine learning algorithms will allow for even more precise and localized weather predictions.
- Improved Satellite Technology: New-generation satellites, such as those recently launched by Tomorrow.io, provide superior data collection capabilities. They come equipped with advanced sensors, enabling them to capture a broader range of atmospheric data with higher resolution. These advancements facilitate a granular view of weather phenomena, leading to improved precision in local weather forecasts. Moreover, with modern satellites’ reduced latency, we can access almost real-time data, making forecasts more timely and relevant. The combination of increased volume, precision, and timeliness of data will significantly enhance our ability to derive valuable insights from weather data.
- Advanced Data Collection Methods: Besides satellites, other data collection methods are also witnessing improvements. For example, IoT (Internet of Things) devices and sensor networks are increasingly used to capture hyperlocal weather data. Advanced radar systems are being developed to provide more detailed information about precipitation and wind patterns. These advanced data collection methods, in conjunction with improved satellite technology, promise a future where weather data is not only more voluminous but also more diverse, covering various facets of the weather.
- Enhanced Machine Learning Algorithms: The improvements in data collection and volume would be ineffective without sophisticated tools to analyze this data. Fortunately, the field of machine learning is progressing rapidly. Advanced deep learning models, like convolutional neural networks (CNNs), are being used to interpret satellite imagery more accurately. Time series forecasting models, such as LSTM (Long Short Term Memory), can handle the temporal aspects of weather data more effectively.
Moreover, ensemble methods that combine multiple machine learning models are being employed to make more robust and accurate predictions. Techniques such as reinforcement learning are being explored to create adaptive weather models that learn and improve with each forecast. - The Role of High-Performance Computing: To handle the surge in data volume and the computational requirements of advanced ML models, high-performance computing (HPC) solutions are crucial. The use of HPC, along with cloud-based technologies and GPU-accelerated computing, will allow for faster analysis of massive weather datasets and real-time or near-real-time predictions.
The transformation of weather data into actionable insights has immense potential to impact industries, economies, and everyday life. While we’ve come a long way in weather prediction and data analysis, the future is ripe with even more possibilities. As businesses, data scientists, and decision-makers, our task is to harness these advancements effectively, driving growth, sustainability, and innovation. The weather data revolution is here, and the time to act is now.