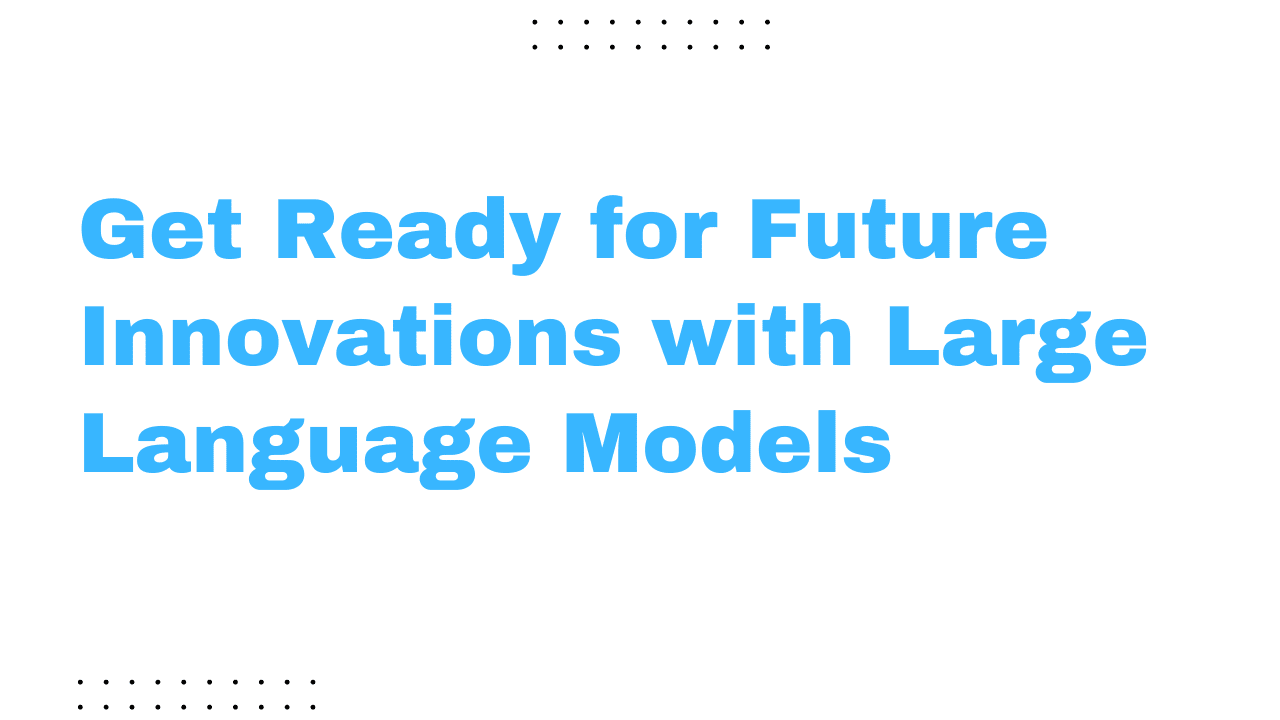
Nowadays, almost all businesses use generative AI and large language models after realizing their ability to boost accuracy in various tasks. These AI models have become the topic of social media discussions nowadays.
This blog explores more on the business and commercial uses of LLMs and genAI along with the differences between them.
What is generative AI in simple terms?
Generative AI is a type of machine learning model focused on the capability of dynamically producing results once it has been trained.
This capability to produce complicated forms of results, such as code or sonnets, is what differentiates generative AI from k-means clustering, linear regression, and different kinds of machine learning.
Generative AI models can only “produce” results as predictions according to the set of new data points.
After training a linear regression model to forecast test scores according to the number of hours spent researching, for instance, it can produce a new prediction if you give it the number of hours a fresh student spent researching.
In this case, you couldn’t rely on prompt engineering to understand the connection between these two values, which is possible with ChatGPT.
Model AI can create original and fresh content from the start when compared to traditional models, as they depend purely on already existing data to forecast outcomes.
After completion of these models’ learning processes, they produce statistically possible results when prompted and can be used to perform different tasks, such as:
- Image generation according to present ones or using the style of one image to change or generate a fresh one.
- Speech tasks include query/answer generation, meaning of the text or interpretation of the intent, transcription, and translation.
What is generative AI vs “normal AI?”
It’s necessary to know GenAI vs. AI before implementing it in businesses. To use normal AI, specialized skills and knowledge are required, whereas anyone can use generative AI. When you are having a discussion about generative AI, then the question “What is the difference between generative AI and discriminative AI?” strikes your mind. Here are the major differences between generative AI and normal AI or discriminative AI:
Generative AI: understands intent and generates content in human tone (e.g., audio, video, text, code, music, and data).
Normal (traditional) AI: Forecast results for particular use cases according to past trends in data.
Generative AI: applies to different applications (e.g., answering complicated questions, creating audio and video, and creating new images) and use cases.
Normal AI: Closely defined, use-case-oriented (e.g., identify an anomaly in a photo, identify fraud, play chess).
Generative AI: data collected through the internet.
Normal AI: accurately chosen data for particular reasons.
Generative AI: More user interfaces (e.g., chat interfaces via web browsers and apps).Normal AI: specialized use-case-oriented applications (e.g., call centre screens, dashboards, and BI reports).
Is GPT a Generative AI?
Yes. GPT models belong to a category of models that are usually called “foundation models.” They can generate human-like content as they are trained on massive amounts of data and can predict the hidden words. Like this, they can usually perfectly forecast the next word, as they are probabilistic models.
What is LLM in simple words?
LLMs (Large Language Models) represent the best form of generative AI. Large language models are modern artificial intelligence systems that have the potential to produce meaningful and contextually valid content.
These models can understand complex trends and language structures as they go through training through huge amounts of datasets gathered from books, articles, websites, and so on.
Based on this, LLMs can progressively produce human-like text, respond to queries, perform particular tasks, and engage in conversations without losing fluency and expertise. Renowned and top large language model examples include Llama (Meta), BERT (Bidirectional Encoder Representations from Transformers), Bard (Google), and GPT-3 (Generative Pre-trained Transformer 3).
GPT-3, introduced by OpenAI, can execute tasks such as creative writing, generating codes, and translation. Google launched BERT, which can understand the search intent and is a base for search engine algorithms.
What is the architecture of an LLM model?
Today, in the natural language processing domain, collecting a dynamic list of large language models describes the expansion of AI-driven linguistic potentials.
When you start researching large language models, you’ll notice that they’re usually constructed using modern deep-learning techniques with a clear concentration on a neural network architecture called a transformer. Transformers are specially designed to process consecutive data, including text, by focusing on various elements of the input and capturing long-range dependencies.
Through this process, LLMs can understand the context and meaning associated with the words and sentences to create logical responses.
How do large language models (LLMs) work?
There are two steps, like pre-training and fine-tuning, that define the working procedures of large language models.
Pre-training: In this stage, the model learns the linguistic structures and statistical trends that exist in the text data, which allows it to produce a common grasp of language. This stage needs an extensive amount of information, frequently in the range of billions of words, to make sure the model records a broad range of contexts and language patterns.
Fine-tuning: In this stage, the LLM is trained on a particular dataset, personalized to the preferred task or application. This dataset is generally more focused and smaller, enabling the model to specialize in a specific task or domain. Additionally, current large language models can be used in online search, chatbots, DNA research, sentiment analysis, and customer service.
Types of large language models in machine learning
Raw or generic language models forecast the subsequent word according to the language in the training data. These models can perform data-recovery tasks.
Instruction-based language models are clearly trained to predict answers to the directions provided in the input. This lets them conduct sentiment analysis or produce code or text. Dialog-focused language models are properly trained to have a dialog by forecasting the succeeding response.
Generative AI vs LLM
It’s important to understand the concept of large language models vs. generative AI, as they both use neural networks and deep learning. Large language models have become popular with the introduction of modern generative tools such as Google’s Bard and ChatGPT.
Generative AI refers to artificial intelligence models that have the potential to produce content like text, images, music, video, and code. Generative AI examples are Midjourney, ChatGPT, and DALL-E.
Large language models are a type of generative AI that are trained on text and create textual content. A common example of generative text AI is ChatGPT. Generative AI acts as a platform for all large language models.
AI vs. LLM
Artificial intelligence technology has existed on the market since 1950 and focuses on developing machines that can mimic human intelligence.
Popular technologies like ML, generative AI (GAI), and large language models have been listed under AI.
Large language models have been developed from generative AI subsets. They can produce text in a conversational tone (human-like) by forecasting the possibility of a word based on the previously used words in the text.
AI represents a large field of study with GPT, ML, GAI, and LLMs, each of which has its own applications, characteristics, and related companies.
What are the components of a large language model?
Large language models include different neural network layers. Attention layers, embedding layers, recurrent layers, and feedforward layers cooperate to process the input text and produce output content.
“The attention layer” allows a language model to concentrate on single portions of the input text that are related to the present tasks. The responsibility of this layer is to produce perfect output.
Embeddings from the input text are created using “the embedding layer.” This portion of the large language model can capture the syntactic and semantic intent of the input.
“The feedforward layer (FFN)” consists of numerous completely connected layers that modify the input embeddings. While doing this, these layers let the model to fetch higher-level abstractions. “The recurrent layer” sequentially simplifies the words in the input text. It understands how words connect in a sentence.
Foundation model vs LLM
Even though both foundation models and LLMs are listed under AI models, they have their weaknesses and strengths. Foundation models are less data-intensive and have a more general purpose, whereas LLMs are more data-intensive and specialized. The excellent model to use for a specific task will depend on the requirements of that particular task.
Let’s discuss their major differences in detail:
Foundation models are generic
It means that these models can be used for all types of tasks. For instance, a foundation model can be used to develop a chatbot, write engaging content, and translate languages.
A large language model is usually only used for one or two tasks, including language translation or text generation.
LLMs are properly trained in language data
As already explained, LLMs are trained in such a way that they can understand the variations of language. It means they are experts in creating semantically relevant and grammatically accurate text. For instance, a LLM can be used to produce text that is both informative and engaging.
A foundation model may not be perfect enough at producing grammatically perfect text because it’s not purely trained on language data.
Foundation models are undeveloped
Foundation models are still immature, whereas large language models are developed and extensively used. This shows foundation models are likely to produce incorrect outputs.
On the other hand, large language models are more reliable and stable, but they might not be as creative as foundation models.
What is the future of LLM?
The new generation of LLMs will successively refine and get “smarter.” They will progressively grow in terms of managing more business applications. Their capability to translate content across various contexts will expand further, making them usable by business users of all levels of technical expertise.
Allowing more accurate data via domain-oriented LLMs developed for specific sectors or functions is another direction for the upcoming large language models. In other words, the use of these models could lead to new examples of shadow IT in companies.
Conclusion
In recent years, both generative AI and large language models have become more powerful. In the upcoming years, businesses not only use large language models for sentiment analysis and text generation, you can see almost all applications you use will be built on LLMs.
Originally published here