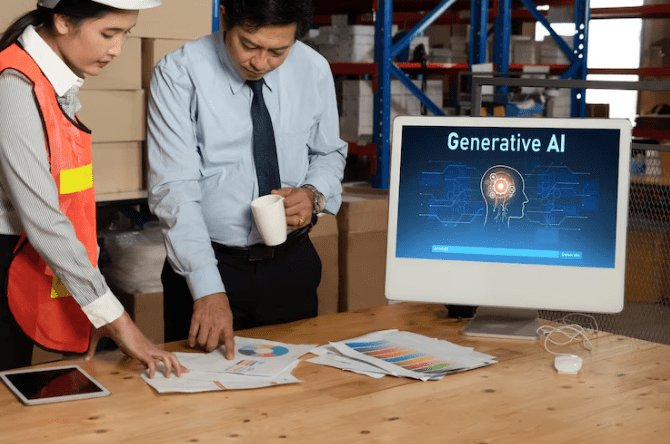
Generative AI is a type of artificial intelligence. It creates new content based on the data it learns. This technology is powerful and versatile. In supply chain management, Generative AI is making big changes. It helps businesses plan better. It reduces mistakes and improves efficiency.
Supply chains are complex systems involving many steps, such as sourcing, production, and delivery. Managing these steps can be tough, but generative AI makes this easier.
This technology uses data to predict and plan. It can suggest the best ways to manage inventory. It can also find faster routes for deliveries. Generative AI is not just a tool for big companies. Small and medium businesses can benefit too. It helps them save money and time.
In this article, we will list and explain the top 5 potential generative AI supply chain use cases.
What is generative AI in the supply chain?
The GenAI method for consumers is just entering a command or query into a text, image, or video field, which causes the AI to produce new content. Large-scale data sets are usually used to train GenAI models, and when a user enters new data, the program leverages both the new data and what it has already learned to produce new content.
Every facet of the supply chain, including demand, logistics, and inventory, can be used to train GenAI. GenAI can enhance supply chain management and resilience by evaluating the organization’s data.
Top 5 use cases for GenAI in the supply chain
Supply chain executives should evaluate if GenAI’s capabilities match business goals and weigh the potential advantages and disadvantages before implementing GenAI applications in the supply chain.
These are a few supply chain management application cases that show promise.
1) Predictive maintenance
Equipment failures and unscheduled downtime can severely interrupt supply chain operations, often resulting in significant financial losses. In this scenario, Generative AI in predictive maintenance is a key tool for putting predictive maintenance plans into action. This is performed by analyzing information such as sensor data, previous maintenance records, and equipment performance indicators.
Generative AI systems that detect patterns and irregularities in data can predict when maintenance is needed. As a result, firms can reduce downtime, extend equipment life, improve operational efficiency, and save money on maintenance by planning repairs or replacements ahead of time.
2) Supply chain optimization
Generative AI models may analyze a variety of visual or textual data sources, including traffic conditions, fuel prices, and weather forecasts, to determine the most effective transportation routes and schedules. The AI can develop several scenarios and, based on the required optimization parameters, provide the best solutions for cost reductions, shorter lead times, and increased operational efficiency throughout the supply chain.
Unilever, a multinational consumer goods company, has integrated artificial intelligence into its supply chain to reduce supplier risk. Unilever uses machine learning and generative AI models to monitor external events such as political turmoil, natural disasters, and market developments that may affect its suppliers. This method assigns risk rankings to each supplier and provides other sourcing choices when disruptions are expected.
During the COVID-19 pandemic, Unilever’s AI-driven risk management system allowed it to quickly modify sourcing methods and ensure supply chain continuity, particularly for crucial items such as cleaning products.
3) Inventory evaluation
GenAI may also aid with inventory management. GenAI which has been trained on crucial data such as stock levels, warehouse capacity, and manufacturing time can identify strategies to optimize inventory procedures, such as when to refill or remove stock, thereby reducing extra storage. Companies pay more to store extra items, so eliminating excess stock could save money.
4) Transportation and route optimization
Generative AI has the potential to significantly improve transportation and routing optimization within supply chain management. By analyzing massive volumes of data from diverse sources, AI can produce efficient transportation plans, save time, and increase overall supply chain logistics efficiency.
Generative AI enables:
- Route optimization results in lower costs and faster deliveries.
- Vehicle and fleet optimization, including vehicle wear and tear and resource utilization.
- Dynamic routing that adapts to disturbances and delays.
With these creative technologies, it is possible to maintain a resilient supply chain.
5) Supplier-customer communication
Frequent contact between a company’s suppliers and consumers is essential for an efficient supply chain, but making communication as effective as possible can be difficult.
GenAI can send out communications automatically, eliminating the need for employees to do so. Large language models and natural language processing may ingest data from sources such as market events that may affect suppliers and traffic delays concerning specific shipments, allowing GenAI chatbots to alert suppliers to risks. GenAI chatbots can also manage certain consumer inquiries, such as processing returns or tracking deliveries.
Challenges in Adopting Generative AI for Supply Chains
Adopting Generative AI for supply chains is not always easy. Businesses face several challenges when they try to use this advanced technology. Let’s look at the main ones.
1. High initial costs
Setting up Generative AI systems can cost a lot of money. Businesses need to invest in software, hardware, and training. Small companies may find it hard to afford these costs. This makes adoption slower for some industries.
2. Training employees
Employees need to learn how to use Generative AI tools. This takes time and effort. Not everyone is comfortable using new technology. Companies must spend money and time to train their teams. Without proper training, AI tools may not be used effectively.
3. Data privacy concerns
Generative AI relies on large amounts of data. Sharing and storing this data can raise privacy concerns. Companies need to ensure their data is secure. Any breach of sensitive information can harm the business and its reputation.
4. Integration with current systems
Many businesses already use older systems for supply chain management. Generative AI may not fit well with these systems. Updating or replacing old systems is expensive and time-consuming. Integration issues can delay the benefits of AI adoption.
5. Complexity of implementation
Setting up Generative AI systems requires skilled experts. Businesses need help from AI specialists to configure and maintain these tools. Finding the right experts can be difficult and costly.
6. Resistance to change
People often resist change. Employees may worry that AI will replace their jobs. This fear can create resistance to adopting the technology. Businesses need to communicate clearly that AI is here to assist, not replace, workers.
7. Uncertain ROI (Return on Investment)
Some companies worry if AI will provide enough benefits for its cost. The return on investment is not always clear at the start. This uncertainty can make companies hesitant to adopt the technology.
These challenges can slow down the adoption of Generative AI in supply chains. However, with the right planning and support, businesses can overcome them and unlock the full potential of this technology.
Final words
Generative AI is transforming supply chain management. It helps businesses make better decisions. It improves demand forecasting, inventory management, and supplier coordination. With its power, companies can save time cut costs, and reduce waste.
Using Generative AI can also help in responding to unexpected challenges. It enables smarter logistics and production planning. Businesses can become more sustainable and efficient.
However, adopting Generative AI comes with challenges. Initial setup costs and training are necessary. Data security is another important factor. But with the right approach, these challenges can be overcome.