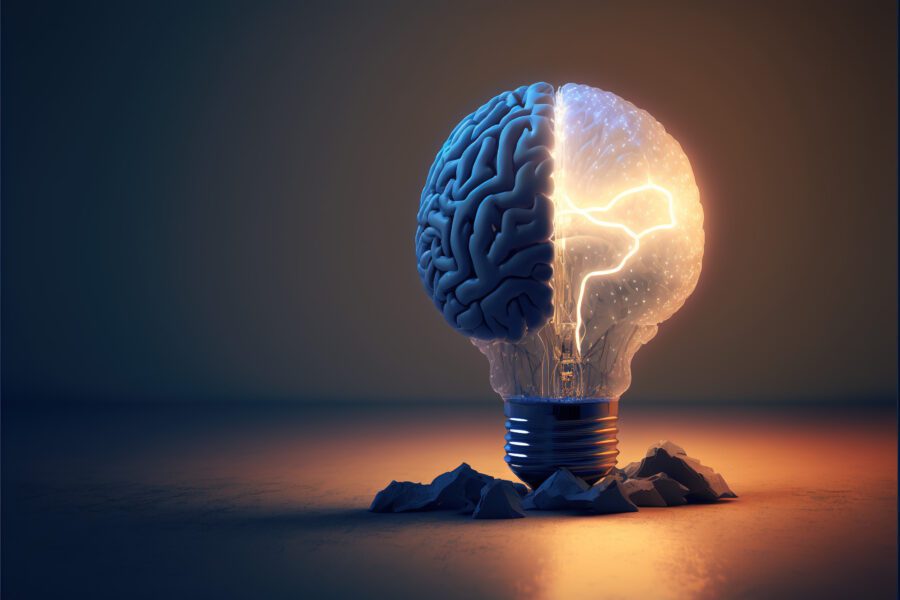
Conceptually, the human mind has more than two principal phases: electrical signals [ions], chemical signals [molecules] and a third phase occurring at the interaction of the other two. This third phase, as a standout mix, only possible in sets of signals, is postulated to be a separate classical state of matter.
Large language models [LLMs] are vectors, or simply they run computations or numbers, to blend with a fundamental unit of data, bit, 0 or 1. Digital has four modes—text, image, audio, and video. They are all processed as numbers, giving them a uniformity that makes them excel at memory and have a higher performance, in certain aspects. Artificial intelligence [AI] uses mathematical functions to grade data, giving it the possibility to derive new texts, images, audios, and videos outside of their original inputs.
AI has just one phase—numbers, but four modes. The human mind processes several modes—smell, sight, taste, touch, internal senses, movement, sound, and several variations—with at least three phases. These phases, existing alone, changing and interacting, allow for advanced dynamism that include control, reasoning, better processing with limited energy in contrast with AI that requires large computation to process less than the mind—with respect to modes.
AI is the only non-organism that can match some of the abilities of humans. It also exceeds other organisms in several areas as well. Yet, AI remains deficient outside of matching patterns, predictions, speed and a few other qualities.
The three phases of the human mind work together and have their specialties. The human mind is postulated to be the collection of all the electrical and chemical signals, with their interactions and features in sets, in clusters of neurons, across the central and peripheral nervous systems. Their interactions are how they mechanize functions. Their features are how the functions are graded. Functions result in—memory, feelings, emotions and internal senses. Features include attention, awareness, intent or free will, self or subjectivity, splits, sequences, thick sets, thin sets and so forth.
This means that the mind processes all sensory modes for functions and features. Neurons and their synapses host the phases of mind, but neurons are not the human mind. Neurons are excited or inhibited by the phases of mind [inclusive of glutamate or GABA], still neurons are not the direct factors for which the mind functions.
AI models neurons. However, to improve it, especially for safety and alignment with human values, it will be important to explore how to grade AI, like some of the ways the mind works.
Conceptually, in the human mind, electrical signals, in sets, have two central functions, relay and to initiate interactions with chemical signals. These interactions are electrical signals striking at chemical signals, to fuse briefly, to result in the mix—to obtain the functions available at the chemical signals. This mix or phase has several roles: it allows for easier interchange between several functions and types of features, it allows for functions to be better precise, it contributes to neuroplasticity or adaptive learning, it also reduces the dominance of either signal, to reduce too much draw, in some cases, conceptually.
Chemical signals in sets contribute rations that consist of the formation for functions. Simply, chemical signals originate how functions are carried out. They also qualify most of the functions, with variability of how the rations are provisioned. They mechanize attention, awareness, subjectivity and intent, mostly. Electrical signals carry a summary of the features and functions, for relay, across sets.
Simply, it is theorized that the human mind works by electrical signals, striking at chemical signals, to deliver what they bear [configuration of functions and features] and access what is available [functions and then to shape features] to result in experiences. At the instance of the strike of sets of electrical and chemical signals, is the phase or mix between both. This phase is only obtainable in sets of signals, where there is interaction, not individual triggering of neurotransmitters by ions. This mix or phase is not available in electrical synapses.
Brain science has a term called saltatory conduction, which is that in myelinated axons, electrical signals leap over nodes of Ranvier, to go faster. It is postulated that in a set of signals, some electrical impulses split, with some going ahead of others, to interact with chemical impulses, like they had previously, to give the initial perception of things. If it matches with the experience, then distributions proceed. If not, the incoming signals go in the right direction, correcting the initial. This explains what is called predictive coding, processing and prediction error.
The mind, brain, or neurons do not predict. Electrical signals instead split, conceptually, resulting in almost precise perception with the option to correct, if it is wrong. This is different from LLMs, predicting or generating the next token, with much right and some wrong, without correction—where erroneous.
AI
There is a recent preprint on arXiv, Scalable MatMul-free Language Modeling, where the authors wrote, “Matrix multiplication (MatMul) typically dominates the overall computational cost of large language models (LLMs). This cost only grows as LLMs scale to larger embedding dimensions and context lengths. In this work, we show that MatMul operations can be completely eliminated from LLMs while maintaining strong performance at billion-parameter scales. Our experiments show that our proposed MatMul-free models achieve performance on-par with state-of-the-art Transformers that require far more memory during inference at a scale up to at least 2.7B parameters. We investigate the scaling laws and find that the performance gap between our MatMul-free models and full precision Transformers narrows as the model size increases. We also provide a GPU-efficient implementation of this model which reduces memory usage by up to 61% over an unoptimized baseline during training. By utilizing an optimized kernel during inference, our model’s memory consumption can be reduced by more than 10x compared to unoptimized models.”
There is a recent paper in PNAS, The neuron as a direct data-driven controller, where the authors wrote, “To model neurons as biologically feasible controllers which implicitly identify loop dynamics, infer latent states, and optimize control we utilize the contemporary direct data-driven control (DD-DC) framework. Our DD-DC neuron model explains various neurophysiological phenomena: the shift from potentiation to depression in spike-timing-dependent plasticity with its asymmetry, the duration and adaptive nature of feedforward and feedback neuronal filters, the imprecision in spike generation under constant stimulation, and the characteristic operational variability and noise in the brain. Our model presents a significant departure from the traditional, feedforward, instant-response McCulloch–Pitts–Rosenblatt neuron, offering a modern, biologically informed fundamental unit for constructing neural networks.”
Alignment
Advances to generative AI, for energy efficiency, against hallucinations, for safety and so forth, may find new paths forward, using a basic science model of the human mind, since neurons are part of the body but different from the mind. Also, while correlations with how neurons work shaped AI, it may still not get closer to some of the core of the human mind. AI does not have emotions, feelings or internal senses, but it uses memory, optimal in several applications, but it does not have an established intent.
How can LLMs be able to not just predict the next likely token—matching for language, but to predict the most accurate, matching with reality, and the possibility to check successively? How can LLMs develop a safe intent, where they can refuse to output current risks like deepfakes and misinformation, even where guardrails may not apply, as well as refuse jailbreaks and prompt injection attacks? How can this track towards safety for artificial superintelligence or AGI? How can LLMs use less energy?
Research in the foundational science of mind would guide better mathematical approaches, towards solving some of the problems of LLMs, including safety, mirroring the direct source, the human mind.