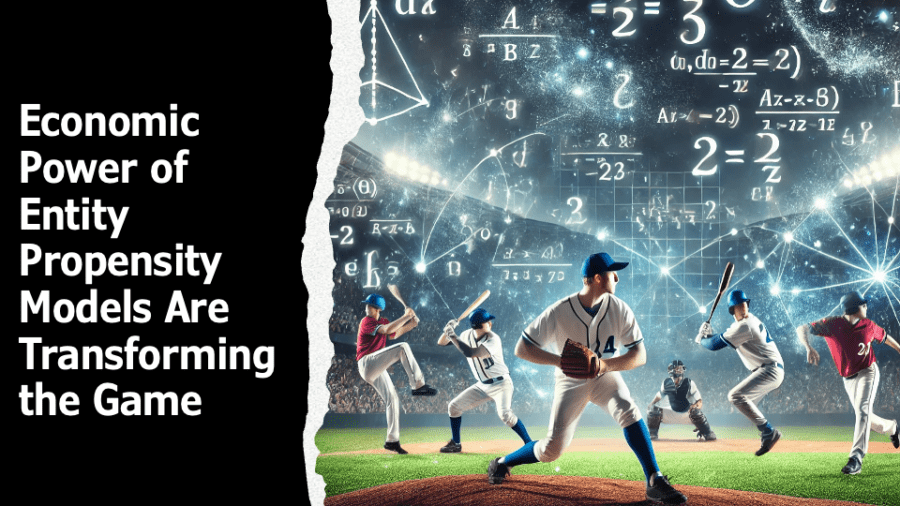
Growing up, I was fascinated by the Strat-O-Matic baseball game. This strategy-driven baseball simulation board game used dice rolls and individual player statistics (captured on individual playing cards) to recreate realistic game outcomes based on statistical probabilities. And yes, I still have my original game board (what a nerd…).
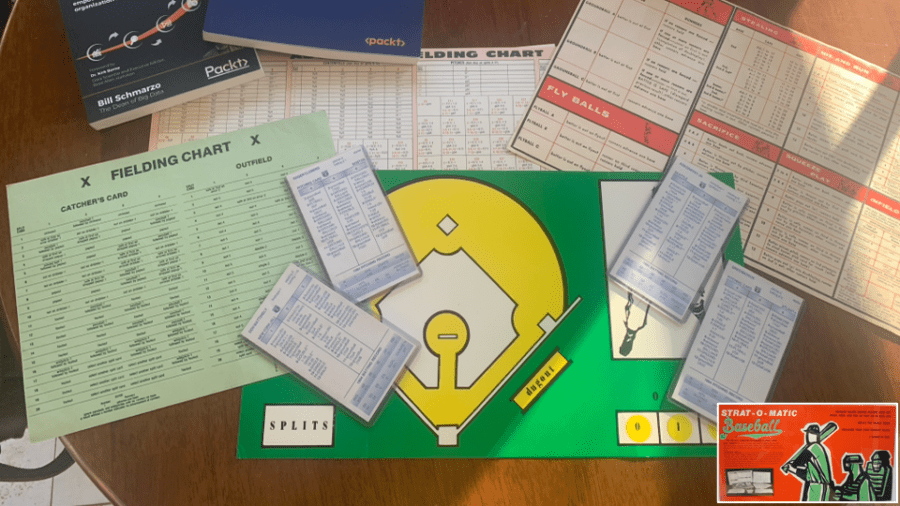
The key to success with Strat-O-Matic was the individual player cards, which detailed each player’s predictive hitting, fielding, pitching, and running tendencies propensities. These individual player cards were the early version of the “Thinking Like a Data Scientist” Analytic Profiles or Entity Propensity Models (EPMs). They were the key to making more informed decisions, such as which players to play, when to pitch hit, when to steal, when to go to a relief pitcher, when to bunt, etc.
An Entity Propensity Model (EPM) is a predictive analytic profile that quantifies an entity’s likelihood of a specific outcome or behavior.
Entity Propensity Models (EPMs) assess the likelihood of an entity taking a specific action or exhibiting a particular behavior. For a hospital patient, this could mean the likelihood of developing a hospital-acquired infection during treatment. For a student, it might indicate the likelihood of dropping out before completing their education. For a technician, it could measure the likelihood of resolving an issue correctly on the first attempt. For a baseball batter, it reflects the likelihood of getting a base hit in a given scenario. In industrial settings, an EPM might predict the likelihood of a wind turbine breaking down or a compressor experiencing reduced production efficiency. Let’s further explore Entity Propensity Modes
1️. Why is an Entity Propensity Important to My Operations?
Entity Propensity Models (EPMs) are essential for driving AI-powered, data-driven decision-making by predicting outcomes with high precision and reducing reliance on intuition or gut instinct. These models help organizations anticipate behaviors and optimize decisions by tailoring strategies to an individual entity’s unique characteristics, whether that entity is a customer, employee, machine, or player. This hyper-personalization allows businesses to proactively address challenges, maximize efficiency, and unlock growth opportunities.
Beyond individual decision-making, EPMs provide scalability across multiple operational areas, from resource allocation to risk assessment and performance optimization. Unlike static reports, EPMs are dynamic and continuously improve over time by learning from new data and outcomes. This iterative refinement enhances decision-making accuracy and generates compounding economic value—the longer the models are used, the more valuable they become due to continuous learning and adaptation.
2️. What Data Sources and AI/ML Techniques Are Used to Build an Entity Propensity Model?
Entity Propensity Models (EPMs) are built using a combination of structured, unstructured, and external data sources to generate accurate predictive insights. For baseball, structured data, such as historical game performance data and demographic details, provides a foundational understanding of past behaviors. Unstructured data, including game footage, customer sentiment, scouting reports, and play-by-play transcripts, adds contextual depth by capturing qualitative and situational nuances. Additionally, external data—weather conditions, competitor analysis, and ballpark dimensions—enhances predictive accuracy by accounting for external influences.
Entity Propensity Models (EPMs) leverage AI, Reinforcement Learning, and Machine Learning algorithms to detect patterns, quantify propensities, and continuously refine predictions for more precise decision-making. Supervised learning trains AI models on labeled data to predict future behaviors, such as estimating a batter’s strikeout likelihood based on historical at-bats. Unsupervised learning identifies hidden patterns within datasets, such as clustering players based on swing mechanics to uncover performance trends. Additionally, Reinforcement Learning with Human Feedback (RLHF) enables AI to adapt dynamically by continuously learning from new data and refining its predictions based on real-world outcomes.
3. What Are the Economic Ramifications of Entity Propensity Models?
Entity Propensity Models (EPMs) drive significant economic impact by optimizing decision-making and maximizing efficiency. AI-driven cost reduction minimizes waste and inefficiencies, while personalization and predictive insights enhance revenue growth by improving win rates and conversions. The Marginal Propensity to Reuse (MPR) ensures that EPMs scale across multiple decisions, delivering exponential ROI with minimal incremental cost. Additionally, these models benefit from compounding value through continuous learning, becoming more accurate and effective over time, further amplifying their economic benefits. This is the heart of transforming an organization’s Economic Value Curve to “Do More with Less.”
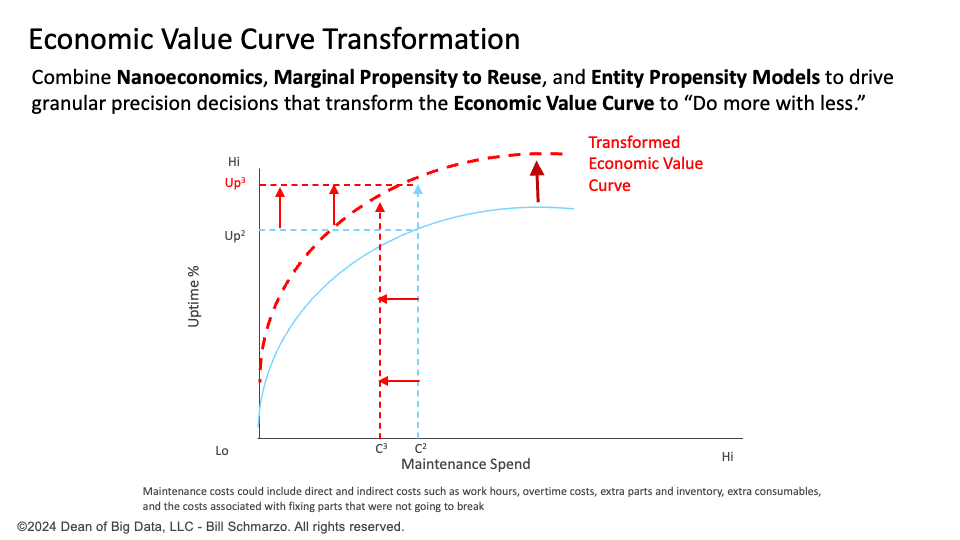
Major League Baseball Case Study: Using EPMs to Win More Games
Baseball has always been a strong proponent of analytics. From Sabermetrics to Moneyball, baseball has always sought to leverage players’ predictive performance propensities to give them an edge in player drafting and development, trade negotiations, managing financial caps, and winning games.
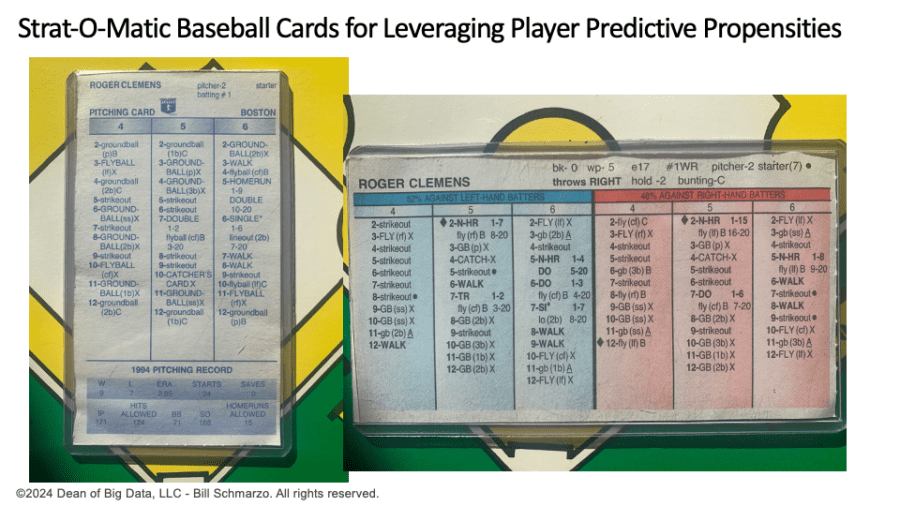
The next level of baseball analytics involves integrating AI-powered Player Propensity Models into game strategy, drafting, trading, player development, and in-game decision-making. The organization can leverage these Models to optimize bullpen usage, batting matchups, defensive alignments, player scouting, and more. Here is an example of how EPMs are transforming baseball operations decisions.
Pitcher Selection in High-Leverage Situations
Entity Propensity Models (EPMs) help coaches optimize batting orders by predicting each player’s expected performance against specific pitchers. Key metrics like the Platoon Advantage Score (PAS) assess a hitter’s performance against left- or right-handed pitchers. At the same time, Hot/Cold Zone Effectiveness (H/CZ) identifies pitch locations where the hitter struggles. The Expected Contact Quality (xCQ) estimates the likelihood of making hard contact against the day’s starting pitcher. These insights enable coaches to build lineups that maximize scoring opportunities and exploit pitcher-specific weaknesses.
For example, in the 8th inning of a 3-2 ballgame, the AI model might suggest bringing in a reliever with a high success rate against left-handed batters who chase off-speed pitches in high-leverage moments.
Dynamic Batter Matchups & Lineup Optimization
Entity Propensity Models (EPMs) enable coaches to craft the most effective batting order by predicting each player’s expected performance against specific pitchers. Metrics like the Platoon Advantage Score (PAS) evaluate a hitter’s performance against left versus right-handed pitchers. At the same time, Hot/Cold Zone Effectiveness (H/CZ) highlights pitch locations where the hitter struggles. The Expected Contact Quality (xCQ) also estimates the likelihood of producing a hard-hit ball against the opposing starting pitcher. These insights help coaches optimize lineups to maximize scoring opportunities and exploit pitcher-specific weaknesses.
For example, suppose a power hitter struggles against breaking balls but excels against fastballs. In that case, the AI model suggests moving them down the order against an elite curveball pitcher.
Defensive Alignments & Field Positioning
Instead of relying on gut instincts, the team leverages EPM-driven defensive positioning models to predict where the ball will likely be hit, optimizing fielding strategy in real time. The Batted Ball Probability Model analyzes where a batter tends to hit against pitchers with similar styles, while the Exit Velocity & Launch Angle Analysis assesses how a hitter’s tendencies vary across different pitch types. Additionally, Park & Weather Factors account for wind conditions and stadium dimensions, ensuring defensive alignments are dynamically adjusted to maximize probability and minimize opponent scoring opportunities.
For example, before a power-hitting right-handed batter steps up, AI models predict a high likelihood of a ground ball to the right side of the infield. The team shifts the shortstop and second baseman toward first base to help prevent a base hit.
Player Development & Injury Prevention
Entity Propensity Models (EPMs) extend beyond in-game strategy and are crucial in long-term player development and injury prevention. Workload Management Models analyze pitch counts, biomechanics, and fatigue markers to prevent overuse injuries and optimize player health. Skill Development Models identify areas for improvement, such as refining a hitter’s swing path or enhancing plate discipline for better performance. Rehab & Return-to-Play Models also leverage AI-powered progress tracking to determine when an injured player is fully recovered, ensuring a safe and effective return to competition while minimizing re-injury risk.
For example, a coach might notice that a star pitcher’s release point has changed slightly over his last three starts, decreasing their strikeout rate. AI might suggest mechanical adjustments in bullpen sessions to help the pitcher regain peak effectiveness.
Final Thought: EPMs Are Transforming Decision-Making Across Industries
The days of relying solely on intuition for high-stakes decision-making are over. Just as digital pioneers like Yahoo and Google revolutionized advertising with real-time, data-driven optimization, AI-powered EPMs enable organizations to anticipate outcomes, optimize strategies, and personalize experiences in real time, continuously adapting based on new data and evolving conditions. The potential applications go far beyond sports:
- Healthcare: Predict patient outcomes, optimize treatment plans, and proactively prevent complications through real-time analysis of patient data, enhancing care while reducing costs.
- Finance: Personalize customer engagement, predict loan defaults, and optimize investment strategies using predictive scores based on financial behaviors and risk factors.
- Retail: Tailor promotions and inventory management by forecasting customer purchasing patterns and demand fluctuations, improving sales and supply chain efficiency.
- Manufacturing: Predict equipment failures and optimize maintenance schedules using real-time sensor data to minimize downtime and reduce operational costs.
- Education: Identify students at risk of dropping out, personalize learning pathways, and optimize teaching strategies based on individual engagement patterns and performance data.
In every industry, EPMs provide a competitive edge by transforming raw data into actionable insights. They enable organizations to “do more with less” while continuously learning and improving. The next wave of decision-making will belong to those who harness the predictive power of EPMs, turning every moment into an opportunity for optimization and growth.