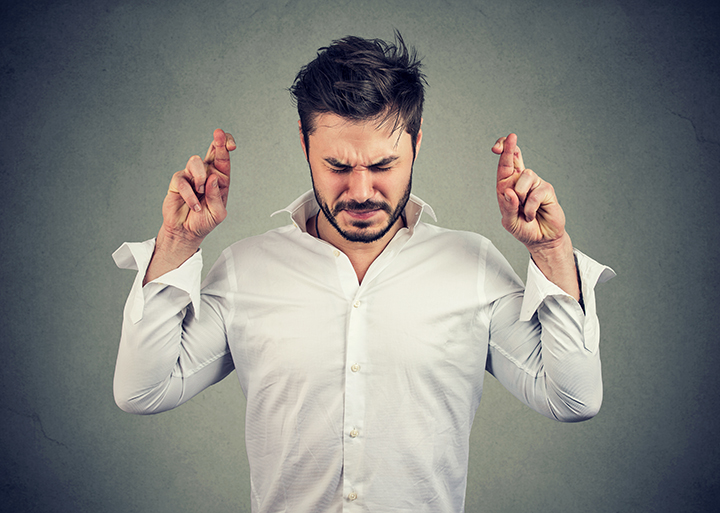
Projects fail. There are many reasons why they do, but a surprising number of them come down to one or more variations of the “Wishful Thinking” theme. From a data science standpoint, this is usually referred to as making faulty assumptions, but the idea is the same. And with very few exceptions, the assumptions being made come not from the data scientists themselves, but from management.
For instance, one myth that seems especially egregious is the notion that the data that you have in your databases can be sucked out into a data lake and immediately made useful for analysis. The reality is that the vast majority of all such data was originally created by programmers who were more concerned about getting their programs to work properly than they were about data fidelity.
Naming conventions will be all over the place, from something clearly identifiable as EmployeeID to Person to EMP to E. When you pull data from tables, most foreign key references (given as numbers) lose any connection to what they are referring to, and there’s nothing like trying to interpret whether a field such as U:235
is a user key, the number of university students, or an isotope of uranium. Context matters, and you cannot maintain context without also storing and accessing the metadata of the data that you work with.
The data that you have will also, more than likely, be dirty. That is to say, there will be different conventions used to describe things, there will be erroneous information that was entered because a programmer didn’t put sufficient constraints on an application front end, or because keys (which should never be exposed to the average person) were exposed to the average person and then typed (wrongly) in another application that uses the same database. This is especially a problem when keys are local, and provide a good argument for the use of URIs or similar global identifiers within databases – even if they are less efficient.
Another area where wishful thinking prevails is in the belief that owners of the various databases within your organizations will necessarily let you have access to them. This is perfectly understandable. Databases do not, in general, exist in isolation. They are used by applications, and any regular retrieval of large amounts of data will impact the performance of those applications. This is, in fact, one of the best arguments for building enterprise knowledge bases – the data involved exists to be read independently of the applications that rely upon them.
Related to this is the notion that it’s possible to feed data from one database into another rather than building out a data hub. I’ve been involved in many, many data integration projects over the years. In my experience, the benefits to be gained by attempting to do this are usually outweighed by the complexity involved in synchronizing all of these data systems. Again, this is an area where an enterprise knowledge base makes sense. Create the keys and metadata for the objects that you are creating within this base, then applications can read this data, do whatever manipulations they have to do, then update the knowledge base with any new relevant data about those objects. Yes, it will cost more in the short-run (because you essentially are building a new application stack), but will pay for itself many times over in the long run.
As organizations shift increasingly towards a data-centric model (rather than an application-centric one), these arguments will become louder and more frequent. It’s easy to store data in tables, but without metadata on the columns of those tables, without an underlying data model that determines an acceptable shape of that data, what’s easier for programmers is not necessarily easiest for organizations. This will often pit the ease of use of programmers (who are application-oriented) against the ease of use of data analysts (who are data-oriented). It is up to the managers within organizations to figure out what the right balance of power is between these two groups, though ultimately the data analysts likely should have primacy, as the data that they work with will ultimately determine policy within your organization.
In Media Res,
Kurt Cagle
Community Editor,
Data Science Central
To subscribe to the DSC Newsletter, go to Data Science Central and become a member today. It’s free!
Data Science Central Editorial Calendar
DSC is looking for editorial content specifically in these areas for March, with these topics having higher priority than other incoming articles.
- Military AI
- Knowledge Graphs and Modeling
- Metaverse and Shared Worlds
- Cloud and GPU
- Data Agility
- Astronomical AI
- Intelligent User Interfaces
- Verifiable Credentials
- Digital Twins
- 3D Printing
DSC Featured Articles
- Exploring the World of Algorithmic Trading Rumzz Bajwa on 08 Mar 2022
- New $1 Million Biennial Prize to Revitalize Statistics Vincent Granville on 08 Mar 2022
- Ploomber vs Kubeflow: Making MLOps Easier Michael Ido on 07 Mar 2022
- 5 Product Management Tips for Data Science Projects MitulMakadia on 07 Mar 2022
- Understanding Distance Metrics and Their Significance ajitjaokar on 07 Mar 2022
- How to Pick the Right URL for Your Landing Page EdwardNick on 06 Mar 2022
- DSC Weekly Digest 01 March 2022: Taxonomists Classify, Ontologists Conceptualize Kurt Cagle on 06 Mar 2022
- Decisions Part 1: Creating an AI-driven Decision Factory Bill Schmarzo on 06 Mar 2022
- How to Make Glowing Visualizations – Literally Vincent Granville on 02 Mar 2022
- The Hybrid to Give Your AI the Gift of Knowledge Marco Varone on 01 Mar 2022
- Ten years of Google Knowledge Graph Alan Morrison on 01 Mar 2022
- Abundance Mentality is Key to Exploiting the Economics of Data Bill Schmarzo on 28 Feb 2022
- Abundance Mentality is Key to Exploiting the Economics of Data Bill Schmarzo on 28 Feb 2022
- DeFi platforms: What dumb data and dumb code have in common Alan Morrison on 28 Feb 2022
- Application Integration vs Data Integration: A Comparison Ryan Williamson on 28 Feb 2022
Picture of the Week
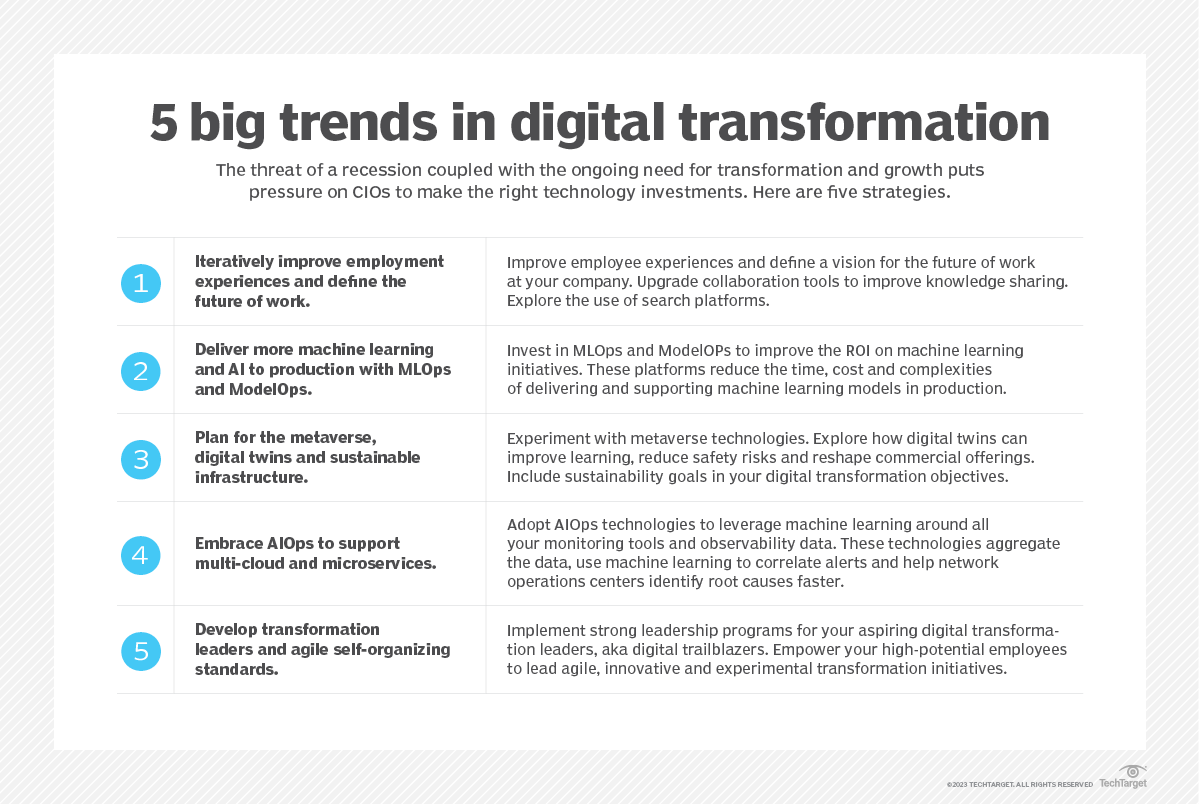