Announcements
- Enterprises today are experiencing rapid growth, citing IT observability as an essential business imperative so companies can properly monitor and manage their complex environments. Tune in to the Pursuing Full-Stack IT Observability summit to learn from leading experts how to establish enterprise observability with the right approach and solutions. Learn about the leading platforms and tools to help companies achieve full-stack observability and keep business environments functioning smoothly.
- Attackers have many opportunities to strike on-site and cloud-based enterprise applications from early in the development process. But many solutions and tools — such as the emerging DevSecOps framework – are available to better secure applications and ensure security is prioritized within DevOps and application security testing tools. Tune into Effective Application Security summit to hear leading experts discuss how to secure applications in your enterprise infrastructure with strategies like DevSecOps along with the right combination of tools and testing.
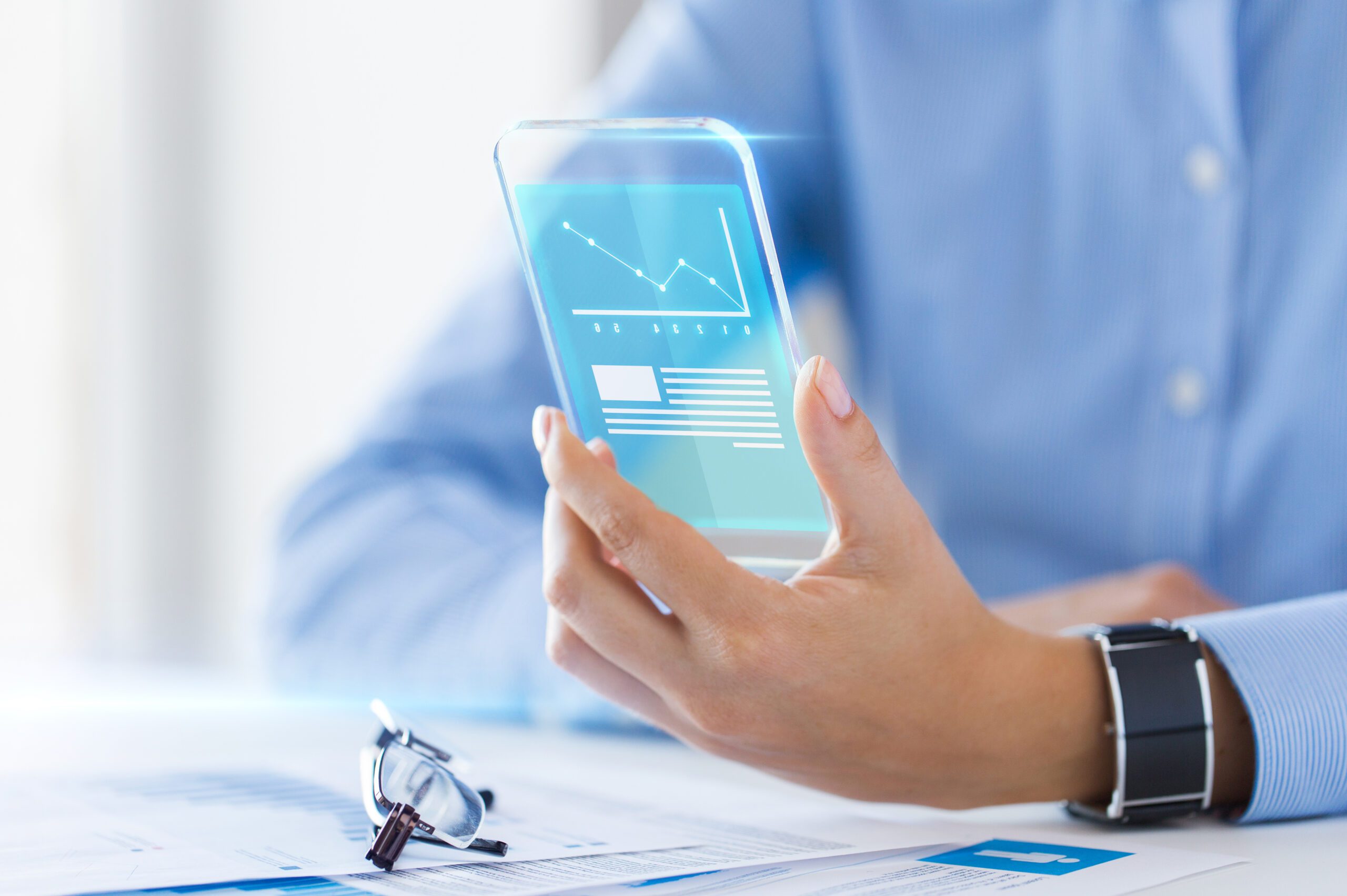
LLM success depends on quality, transparent data
Everyone from writers to coders wonder if their job is in jeopardy as prognosticators say generative AI tools will take over business in the coming years. Of course, these large language model chatbots are still unreliable, and certainly can’t be trusted to complete jobs as well as humans…at least not yet.
But as we discussed last week, the future marriage between human workers and AI will likely be highly collaborative. The rise of LLM only reinforces what business leaders have known for years: Quality, accurate data is integral to success. It’s essential to train LLMs using high-quality data samples so it is able to understand various language complexities — LLMs trained using low-quality data sets can result in incorrect, biased results.
Data accessibility and transparency is essential as tech companies large and small tap into data to create LLMs. These models must be trained with quality data to be successful, so humans developing them rely on using accurate data that is also readily available.
Bill Schmarzo notes in his “AI for Everyone” series that it is vital to educate and empower everyone to actively participate in generational AI design to ensure its development remains responsible and ethical. In part 2 this week, Bill continues to outline how a “Thinking Like a Data Scientist” methodology drives an inclusive, collaborative AI development process. Much like data scientists, responsible AI development will rely on high-quality, accessible data to be successful.
The Editors of Data Science Central
Contact The DSC Team if you are interested in contributing.
DSC Featured Articles
- 6 Reasons Real-Time Data Analytics is Beneficial for Your Business
May 16, 2023
by Gregory Batchelor - 5 Ways to Use Analytics to Inform Website Development Decisions
May 16, 2023
by Gregory Batchelor - Publishing Industry: The Extreme Crucial Role of AI in Content Moderation
May 16, 2023
by Roger Brown - 5 signs showing you need better data management
May 16, 2023
by Ben Herzberg - The AI faithful vs. the data skeptic
May 16, 2023
by Alan Morrison - How Big Data and Scraping Can Help Evaluate News Accuracy
May 16, 2023
by Aleksandras Šulženko - AI for Everyone: Learn How to Think Like a Data Scientist – Part 2
May 14, 2023
by Bill Schmarzo - Building a Secure Workplace: 5 Strategies to Raise Cybersecurity Awareness
May 10, 2023
by Evan Morris - 4 pillars of modern data quality
May 10, 2023
by Vanitha - You should never neglect to monitor your machine-learning models
May 10, 2023
by Prasanna Chitanand
Picture of the Week
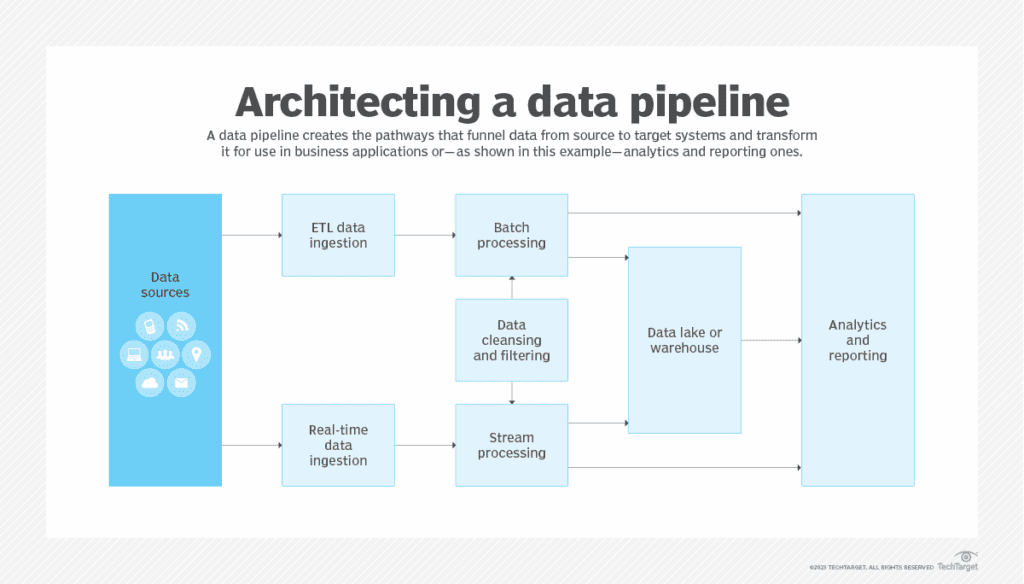