Announcements
- In 2023, organizations will battle a more complex threatscape than ever as web application breaches continue to rise, credential theft and credential stuffing remain a concern, and ransomware demands hit the big players. It’s no wonder that CISOs and security practitioners are more concerned than ever before. Join threat researchers and leading CISOs as they discuss the latest cyber threats on the horizon for 2023 and share how businesses can effectively ‘horizon scan’ to make themselves as secure as possible for the coming year.
- Digital transformation is essential for companies to survive and thrive among today’s volatile market and the proliferation of hybrid workforces. IT leaders need guidance on how to best digitize their environments in a way that ensures they are utilizing the right technology, and all areas of the business will reap the benefits. Join expert thought leaders for the three-day Accelerating Digital Transformation summit to hear digital transformation success stories, how advancing technologies like AI and RPA can enhance transformation efforts, and proven tips to integrate these new practices.
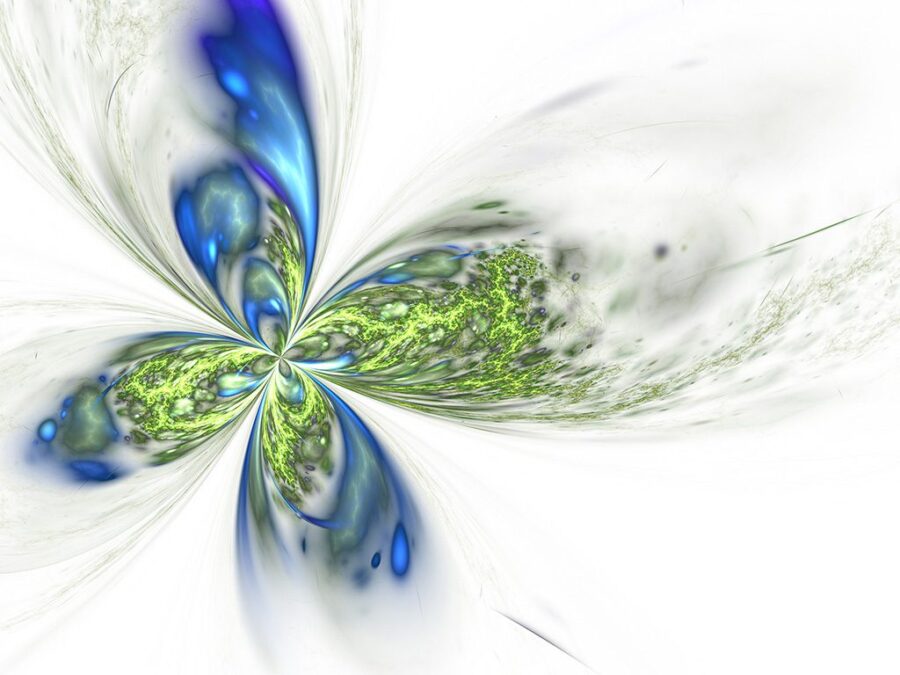
Why the Future Seldom Matches Expectations
This week saw the news that two major automotive companies, Ford and VW, were walking away from a multi-billion dollar investment into Argo AI, a venture intended to build self-driving vehicles. Instead, the companies hope to roll at least some of that effort back into augmenting drivers’ abilities to drive safely and efficiently. This follows a similar announcement a few weeks before by Elon Musk that reduced expectations about how quickly Tesla would bring true autonomous vehicles to the marketplace.
When you get down to it, an analyst’s role is to predict the future. To a very crude approximation, you can divide analytics into the attempt to predict the future (predictive analytics) and the attempt to determine why previous predictions of the future failed (forensic analytics). Given this level of demand, how much supply should be available? Given these economic conditions, how should IT budgets be spent? Given these polls, who will win the election?
Even in the more abstract realm of machine learning, this philosophy holds: Given this training data, what is the likelihood that a given datum can be categorized in a certain way? Given a sequence of words, what are the most likely phrases that will follow them? The analyst builds models assuming that most changes are predictable, given enough data. Yet a surprising number of models fail to predict the future accurately, no matter how much data they gather.
The Dreaded Chaos Butterfly
The Butterfly Effect came initially from an L. Sprague De Camp story, “The Philosopher and the Gun,” in which a time traveler travels to the Jurassic with the injunction that he should not stray from a marked path. When he accidentally steps on a butterfly, the protagonist discovers that his home language is radically altered upon his return.
Ironically, meteorologist Edward Lorenz discovered, when modeling weather patterns, that when an equation using the logistics function is applied to complex numbers, the result is a disconnected cloud of data points. However, when enough of these points were plotted, an orbit that looked surprisingly like a butterfly emerging from the chaos. One interpretation of this pseudo-path (which should not be strayed from) is that the future is susceptible to even small changes in starting conditions in chaotic situations.
Countering this interpretation is the discipline of probability. The foundation of probability is that when all events are independent, the aggregate distribution of configurations follows what’s known as a Gaussian or Normal curve. Throw enough dice, and the likelihood of a certain income can be predicted with high precision following the familiar Bell Curve shape.
On the other hand, if a tiny, weak bar magnetic is baked into each die, then the distribution is anything but familiar (and more than likely follows what’s called a Pareto distribution, or 80/20 rule). The magnets affect how each die will land relative to the others. The variables are no longer independent but instead have some degree of correlation. They will likely follow a continuous distribution, but if the model assumes the variables are independent, then the model will be wrong.
Of Polls and Politics
What happens in an election when the polls say one thing, but the other candidate wins decisively? Are the polls deliberately wrong? Was the election rigged? Should polling be ignored? The midterm elections are now underway in the United States to determine the members of Congress and many gubernatorial and local races. These elections are called “mid-terms” because they occur at the halfway point of a President’s term and often serve as a barometer of that President’s performance.
Midterm elections can be notoriously difficult to model, especially two years into a president’s first term. Polling is historically sparse (and this year is sparser than most). Push polling, in which a poll is used to push a particular framing of issues and is, in fact, a form of propaganda, is being more heavily used, and several key issues that can affect single-issue voters are currently in play. The economy, similarly, is in flux after the pandemic. Finally, this is the first year after the regular redistricting process that occurs each decade, as many political boundaries have shifted in the wake of the loss or gain of population. This means there is less reliable data about how people will vote than in 2020 when the polls were pretty accurate (there was a decade worth of data by that point to inform the model).
When an analyst or data scientist models a future scenario, they guess the shape of distribution by determining how strong the bar magnets are within the dice. This determinant is usually expressed as a margin of error (or a related quantity known as the variance) of the samples. This year, the variance is high: the likelihood of the mean of any sample being different from the mean of the actual distribution is significant. Expressed differently, the number of elections where the pollsters will guess incorrectly will likely be higher than they would in a “normal” election. The polls may be 100% accurate, but it’s also possible that the polls will be only 60-70% accurate, which, in tight elections, could determine the balance of power.
It’s worth noting that this does not imply that the election was rigged or incorrectly counted. Despite a concerted effort to discredit the validity of the election process, there have been few credible instances of election fraud conclusively proved in the last several decades. Claiming otherwise despite evidence to the contrary is also a form of propaganda and is pernicious to democracy in any form.
It should also be stated that the more samples that can be achieved in the election (the only poll that truly matters), the closer the result is to being representative of the population’s intent overall. Expanding the voting period, the number of polling places, voting by mail, and absentee voting have historically had few examples of oversampling (people voting multiple times) and what little does happen tends to cancel out, while restricting the franchise has had severe negative impacts.
Forecasting the Butterfly
In general, you can’t “tame” the butterfly, but you can, in your forecasts, recognize that the future “casting” does follow a few key themes:
- The future is predictable only if no change is happening. This may seem obvious, but any kind of predictive analytics that works on historical data will only be correct if the same events occur tomorrow as they occurred yesterday.
- Stability begets instability. Most changes are like earthquakes – stresses build up until the status quo can no longer be sustained, at which point change occurs disruptively and catastrophically.
- The future begins at the margins. Change occurs when external stressors are more potent than the resistance of the status quo. This usually is more likely to happen where the influence of the status quo is weakest, typically where the number of novel interactions between people is highest (universities, port cities, trade centers, research institutes, anywhere that cultural and scientific ideas can cross-pollinate).
- The status quo is conservative. Those most heavily invested in the status quo benefit from the way things are and typically see a change in a negative light, notably if it reduces their power and influence. They will usually provide the broadest resistance to adoption, and the more such change is threatened, the greater the resistance will become until one or the other breaks.
- Most successful technology succeeds by being disguised. Personal computers would not have succeeded if televisions (which operated on a very different principle) had not come first. The mobile web needed the mobile phone, though today, calling people on a mobile phone is a tiny part of how they are used.
- It’s easier to rebuild. Human beings are notoriously bad about heeding warnings about the future, significantly if it inconveniences them. However, once a catastrophe occurs, they usually build back with the most recent disasters in mind.
- Be aware of deep trends. Demographics, climate change, resource limits, and similar long-term megatrends act slowly, impacting everything. Technology and economics are more transient, while cultural changes are relatively ephemeral.
There is always more, but in general, you should always go into the process of modeling, knowing that there will always be hidden variables that you may not even be aware of, let alone be able to control. Living on the edge of a butterfly’s wing is hard work.
In media res,
Kurt Cagle
Community Editor,
Data Science Central
DSC Editorial Calendar: November 2022
Every month, I’ll update this section with many topics I’m especially looking for and are more likely to be featured in our spotlight area. If you are interested in tackling one or more of these topics, we have the budget for dedicated articles. Please contact Kurt Cagle for details.
- ESG (Environment-Social-Governance)
- Digital Privacy
- The Electric Economy
- VUCA (Volatility-Uncertainty-Complexity-Ambiguity)
- Labeled Property Graphs
- Inferential Machine Learning
- Geospatial Data
- Drone Traffic Control
- Linguistic Intelligence
- Ethical AI
If you are interested in posting something else, that’s fine too, but these are areas that we believe are hot right now.