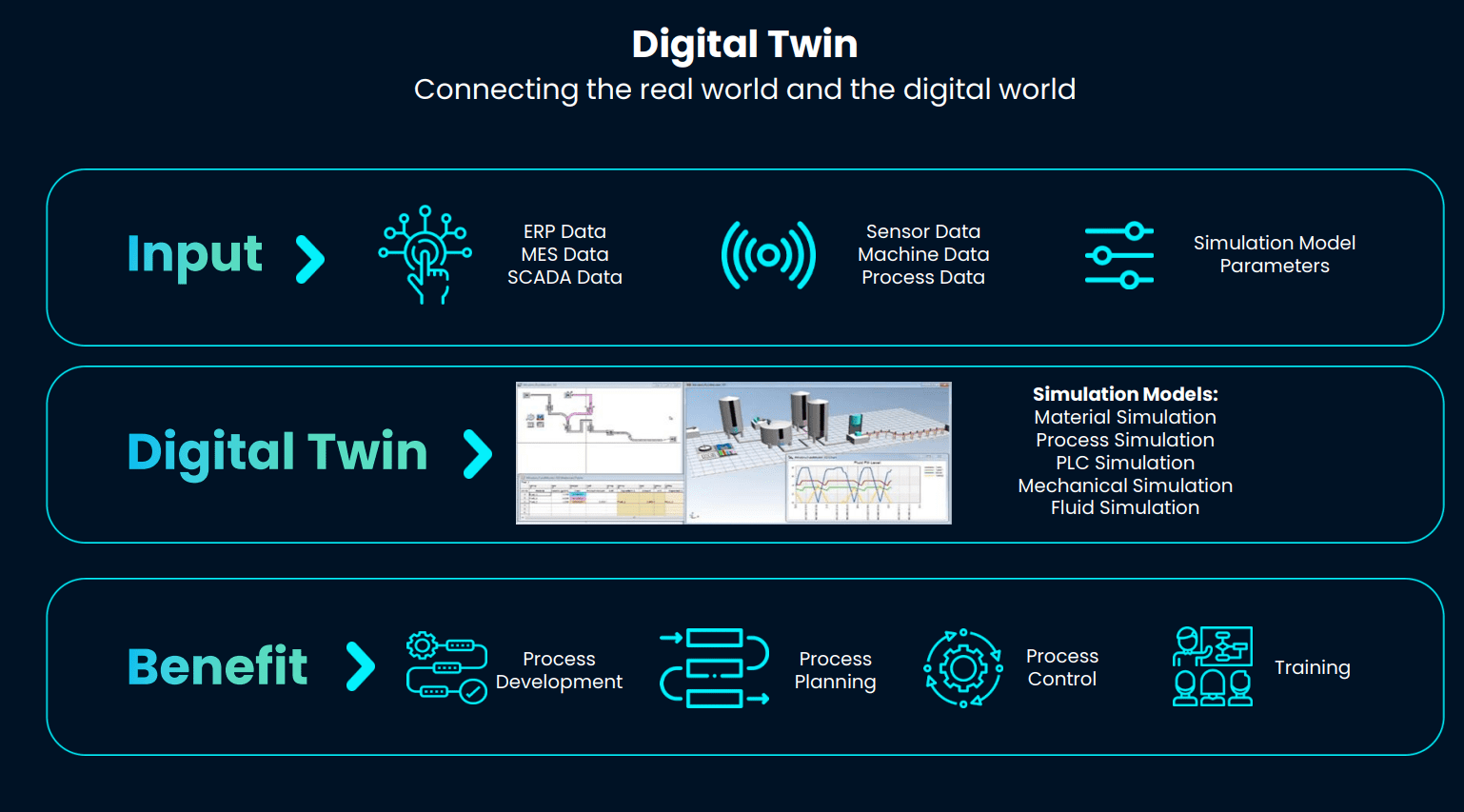
Digital twins analytics has been applied in a variety of contexts. Today, digital twins are gaining in popularity for various complex projects. In this article, we explore the use of digital twins for simulation tasks. We first explain the significance of simulation and then explain how complex manufacturing processes may be simulated as a digital twin.
What is a digital twin?
The Digital twin is a nebulous term because the term digital twin spans industries. Starting from the manufacturing industry, the concept of digital twins has evolved and now applies to many areas, including the metaverse. In addition, companies and industries have chosen to define the term digital twin in their image, giving rise to multiple definitions. However, despite these differences, digital twins have some common elements. A twin is an abstraction – like all abstractions (models of a real system) – we need to simplify the implementation of a twin from its real-life counterpart. Ideally, using the digital twin as an abstraction, we want to solve complex problems. In many cases, these problems involve simulation.
Understanding simulation in digital twins
The concept of simulation originated in engineering. Hence, many machine learning developers are unfamiliar with simulation. Nevertheless, both simulation and machine learning are critical components of digital twins. While they are similar, there are some essential differences.
A simulation follows a set of rules that have been made for it in advance. On the other hand, Machine Learning explores the world and tries to figure out what the rules govern it are. In the simulation, a model is constructed by subject matter experts to predict probabilities of variables for a complex system consisting of a large number of variables. We can think of such a system as a ‘system of systems’ – for example, predicting the behavior of markets that comprise several participants and external factors. With simulation, the inputs are not precisely known, but the model itself is often known in advance because a simulation follows a set of rules we’ve made for it.
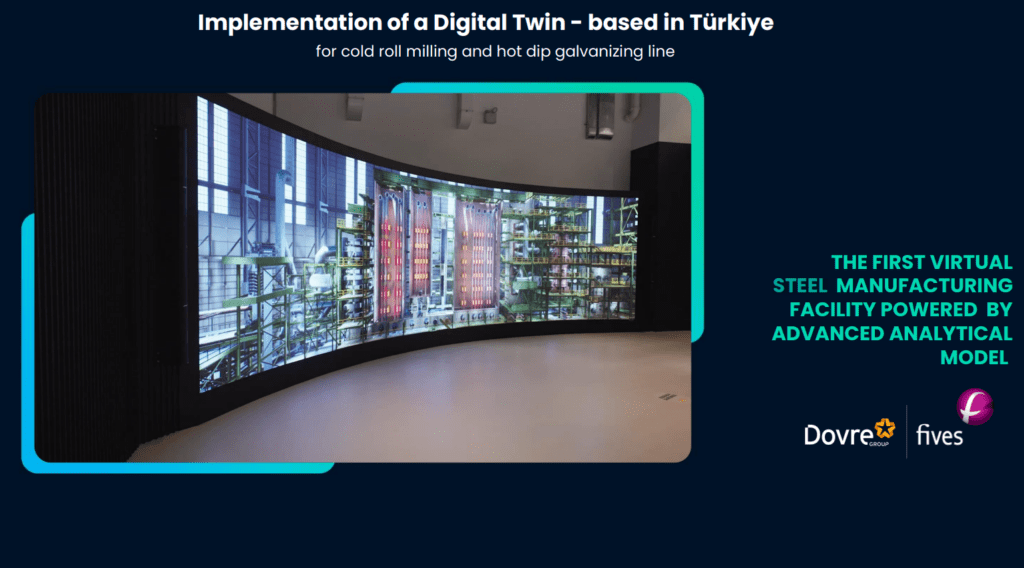
An example of a Digital Twin implementation
With this background, let us explore a case of the use of digital twins for simulating complex manufacturing processes. The example and images are provided by MEXT.
Based in Istanbul, Türkiye, MEXT enables digital transformation by implementing a digital factory. Two production lines are implemented: Intermittent Production and continuous production. For the cold roll milling and hot dip galvanizing line for steel manufacturing, a digital twin is implemented to simulate the manufacture of steel. Data is captured from various sources in the manufacturing process: ERP Data, MES Data, SCADA Data, Sensor Data, etc. From these, the digital twin simulation model and parameters are produced.
Once created, the digital Twin simulates the production process, tests alternative process settings, and supplies optimal settings to the control system to manage the process. Optimal settings can be directly transferred to the control system. You can search for improved process settings by simulating the production process without interfering with the production. You gain deeper insights into the production through simulations and the comparison of the simulations with the real-world process. You can simulate at multiple levels of granularity: Product, Asset, Process, and the entire production process.
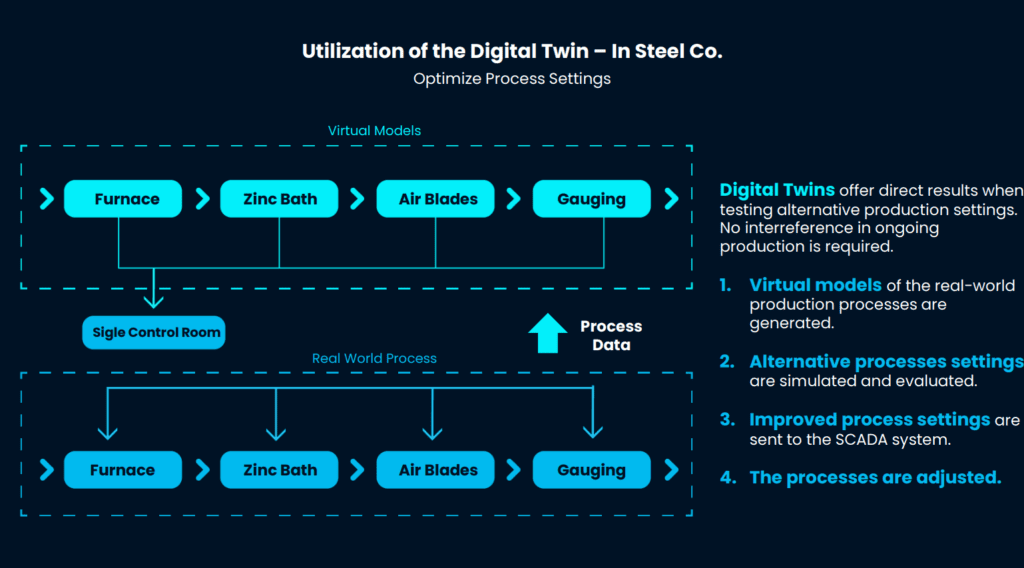
Conclusion
Currently, both digital twins and simulations are relatively niche topics. The concept of simulation is expanding from its origins in engineering. When combined with machine learning, we gain the ability to solve complex predictive analytics problems through digital twins. Developers are also acquiring new skills beyond their traditional machine-learning capabilities.
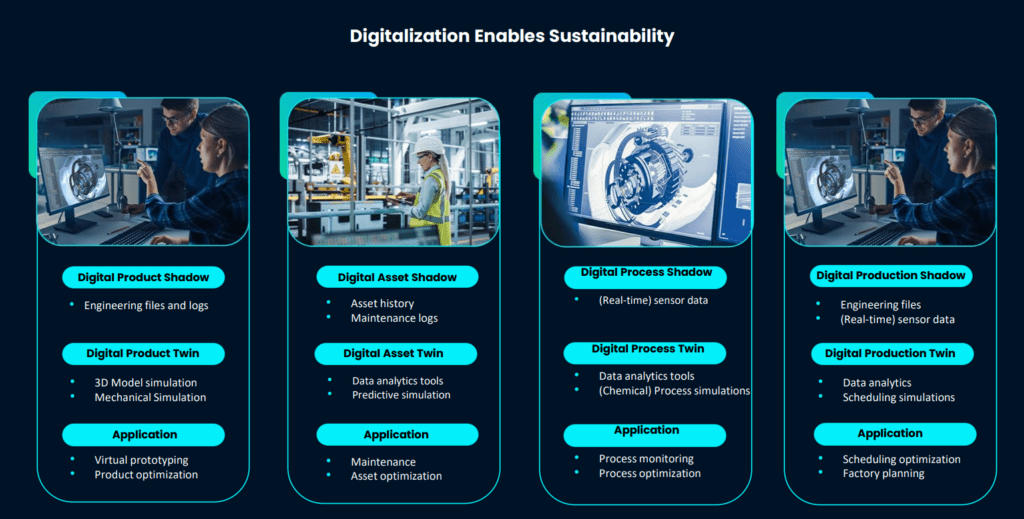