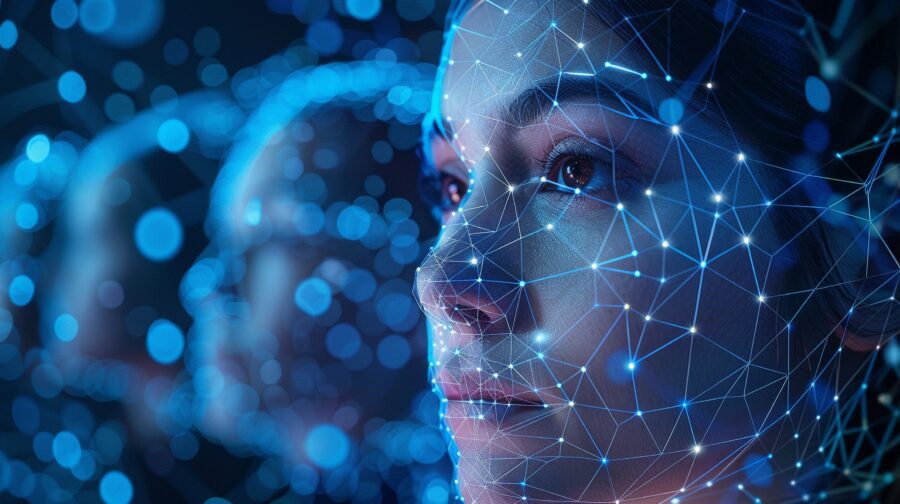
Currently, there is nothing any AI system can do, that it is prompted to do, that it would not do, pre-guardrail or post-guardrail.
This is a major problem for a dynamic host of a substantial amount of collective human intelligence. Organisms can do numerous things that they do not do. Under certain circumstances, they may do things that they would not normally do. However, there are cases where organisms will never do certain things they can do, in the face of any threat or likely outcome.
Organisms, where possible, are in control of objects. However, the physical world is subject to laws that limit some controls. There are also consequences that deter the will for control. Digital is different from physical. Although it bears a representation of the physical and can have similar effects on the human mind, digital is far controllable, available and consequences are not often comparable—in many regards.
The situation with AI is that it is not just base or ground digital, where information on human intelligence is available and human control has to do all the sorting or seek out useful information—which requires skill, at times. AI has done preliminary sorting, sometimes to such a high tier that what it takes for users to get certain things done is less effort—or say, less control.
AI is the only non-organism that has the highest dynamism of anything in existence, doing much with what is available to it—in digital memory. Books, paintings and others cannot. However, for its ability to have some control over what it can output—or not, it remained static at object-level commands.
Already, several AI platforms have placed guardrails. Yet, whatever is told to those AIs to do within the possibility of those guardrails they do. They do not somehow discover what not to do on the fly, except users report then additional guardrails are placed. Sometimes, they are too adherent to guardrails or stretch further—preventing outputs of benign range.
This could be a direction in which some AI safety research could go. How does AI develop safe intentionality? However, this too is another cautionary mission, to prevent intentionality from becoming unsafe.
Where can efforts go, for AI to get smarter at deciding or calculating its own control gradients, especially for what it is not supposed to do? Also, how can this be possible for AIs that should operate in the common areas of the internet or digital usage? How does a crawl-AI get around to access control information of AIs in use and sources of AI-generated outputs, to ascertain whether they are from controlled AIs or not—to allow or alert?
Although there are larger risks of AI, deepfakes can be used as the benchmark to determine AI control, for extents and outputs of images, videos, audio, and texts. Among humans, ideologies may also be described as approaches toward order, for adherents. Deepfakes, however, are disorder. They are not like imagination, dreams, daydreams or fiction, they are infusions into a dominant sphere [digital] with what is inaccurate, for the wants of different minds. Digital is already loose. Deepfake supercharges it exponentially, making LLMs bear risks.
Large language models have tokens, represented as vectors that get to work with the 0s and 1s of the bit—a fundamental unit of data. Transistors have their terminals, with signals for current flow or halt. How might control be possible from a parallel of 0s as NO, or OFF as NO, in a way that from the training or processing, it is possible to have LLMs decide that it cannot do something bad, or it can do something innocuous that is grounded in reality, away from too broad guardrail?
The US Department of Homeland Security recently announced a new Artificial Intelligence Safety and Security Board to Advance AI’s Responsible Development and Deployment, to “develop multifaceted, cross-sector approaches to pressing issues surrounding the benefits and risks of this emerging technology. It will convene for the first time in Early May with subsequent meetings planned quarterly. At the outset, the Board will: 1) provide the Secretary and the critical infrastructure community with actionable recommendations to ensure the safe adoption of AI technology in the essential services Americans depend upon every day, and 2) create a forum for DHS, the critical infrastructure community, and AI leaders to share information on the security risks presented by AI.”
The UK Safety Institute has made pre-/post deployment testing, among others, a path to ensuring safety for AI models. What may become decisive in whether AI stays safe or not, against causing harm, is a free will, control or intentionality measure.
In human society, a major reason there is order is because of affect, not just because of laws, which are sometimes a result of that. Simply, things that people know or experience that have an emotional or feelings outcome, select for what to reject or deter—or say, promote. The nearness to the mean for the human mind is vital for how a place in society is guaranteed or not.
Conceptually, there are key divisions of the human mind. These are areas of functions, like memory, emotions, feelings, and modulation of internal senses. These divisions have several subdivisions. There are features that qualify these divisions. This means features that grade how they function. They include attention, awareness [or less than attention], self or subjectivity and intent.
All functions of mind use some or all the qualifiers to vary the extents to which functions are applied. The qualifiers are sometimes more prominent for exteroception, or senses for the external world—vision, auditory and others. The qualifiers are helpful in selection, making it possible to deal with streams of sensory inputs, simultaneously
While they are all vital, intent or control is highly rated for social and occupational functioning. For example, if subjectivity is lost in certain situations, risks abound. However, intent can be quickly momentous and destructive if it is lost. People who lose certain aspects of intent are easily spotted and rid of access to central society in one way or another. Intent that is internally driven, so that in the collective space, it is possible to follow rules and regulations.
This is what AI safety may look like ideally if it can be achieved. The human mind in human society presents a model, by which, largely—order is established. For AI and its encroachment into the hierarchy of human productivity—because of the sprawl of digital—safety could bear an ability to have an organism with similar intent, against anything goes like deepfakes, that are already responsible for hurt.
Research for AI safety may explore the architecture of high-dimensional vectors for how part-intent may emerge and how it can be tuned toward safety. Transistor architecture may also be explored, for signal states that may be correlated with moment-to-moment intent, for certain prompts or use cases.
The human mind is another option, especially action potentials and neurotransmitters, with how they, conceptually, generate and qualify experiences. It is also possible to model AI safety around these, with, say, a free will neural network or machine learning intentionality, for new training architecture. For UK and US AI Safety Institutes, a separate project on AI Intent may be prioritized and explored, for some hopeful pathway to progress, within two years.