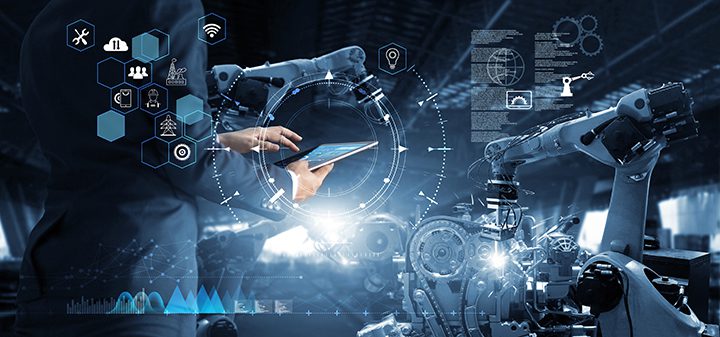
This is part 1 in a multi-part series on the value-realizing, collaborative power of decisions.
My mom used to say, “If it was a snake, you’d be dead”. And the reason that I say that is that organizations are seeking a collaborative value driver that can 1) align the organization around the economic power of data and analytics while 2) providing that “clear line of sight from data to value”. And that value driver is slithering right in front of us – Decisions. Yes, something as simple and prevalent as decisions can be that collaborative linkage point.
The foundational principle of the Value Engineering Framework – providing that clear line of sight from data to value – is identifying, validating, valuing, and prioritizing the decisions (and the KPIs against which to measure decision effectiveness) that the business stakeholders need to make in support of an organization’s strategic business initiatives (Figure 1).
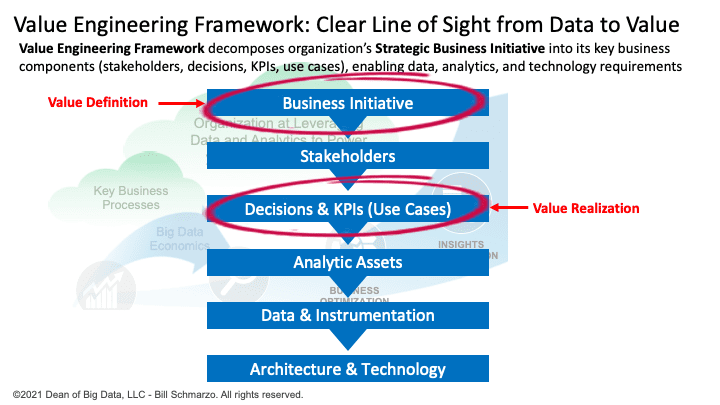
Figure 1: Value Realization Power of Decisions
Once we have a solid understanding of an organization’s strategic business initiatives, we can functionally decompose those business initiatives into the Decisions that a diverse set of stakeholders need to make in support of that business initiative.
Why are decisions such a powerful driver of business and data science collaboration (Figure 2)?
- Decisions are easily identifiable (every business stakeholder knows what decisions they are trying to make)
- Decisions have attributable value; that is, one can attribute “value” to making better decisions (where the thorough and holistic definition of “value” can be a complicated but necessary alignment exercise)
- By their very nature, Decisions are actionable
- Data Scientists know how to optimize Decisions
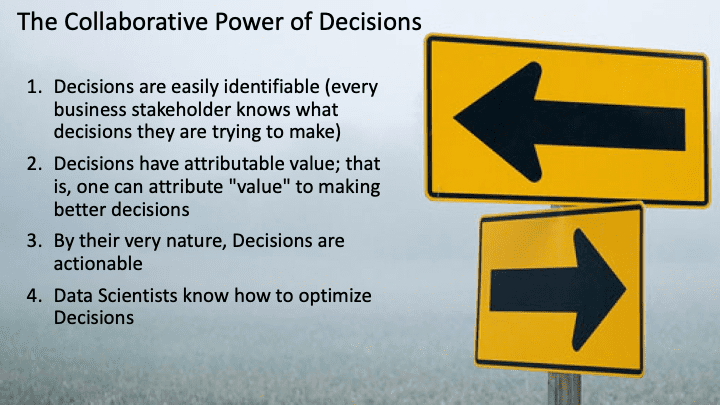
Figure 2: The Collaborative Power of Decisions
Note: a decision is very different than a question. A question is great for validating understanding and driving ideation, but a Decision drives actions.
Scaling Decisions with AI-power Decision Factory
A Harvard Business Review article titled “Competing in the Age of AI: How machine intelligence changes the rules of business” by Marco Iansiti and Karim R. Lakhani talked about how Ant Group, the world’s largest Fintech company, is leveraging AI to transform the financial services industry.
At the core of the Ant Group business model is an AI-powered decision factory. This decision factory runs the millions of daily ad auctions at Google and Baidu, decides which cars offer rides on Didi, Grab, Lyft, and Uber, sets the prices of headphones and polo shirts on Amazon, runs the robots that clean floors in some Walmart locations, enables customer service bots at Fidelity, and interprets X-rays at Zebra Medical.
An AI-powered decision factory is especially powerful in its ability to scale business and operational value by continuously learning, adapting, and refining the decisions being made in a world under constant market and customer change (Figure 3).
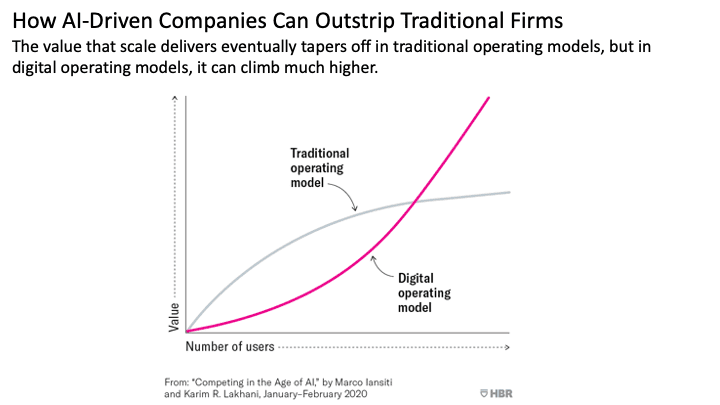
Figure 3: How AI-Driven Companies can Outstrip Traditional Firms
What I found particularly interesting in Figure 3 was the “Digital Operating Model” trajectory (red) line, which highlights how the value of data and analytics assets accelerates as the data and analytics are shared across more users and more use cases. That line behaves a lot like Effect #3 in the Schmarzo Economic Digital Asset Valuation Theorem (Figure 4).
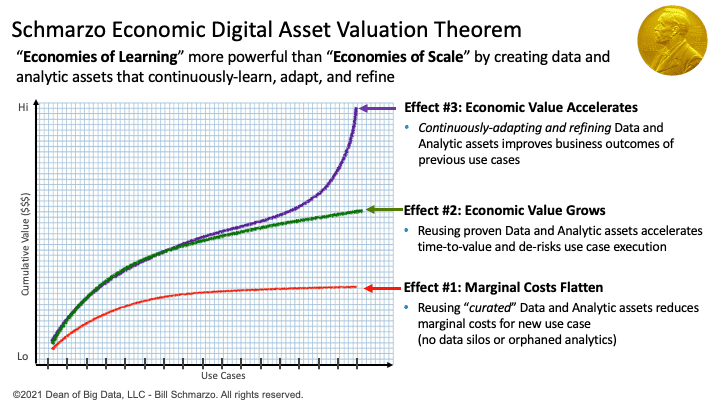
Figure 4: Schmarzo Economic Digital Asset Valuation Theorem
Here are some of the critical enabling technologies that underpin an AI-driven Decision Factory:
- Data Management. A data orchestration platform that automates data discovery, content inferencing and metadata creation, simplifies data access and data exploration, and institutionalizes data governance and master data management with integrated data observability and AI agents to create intelligent data management processes, policies, models, and pipelines that gather, clean, integrate, normalize, enrich, and safeguard data in a systematic, sustainable, and scalable way. See my blog “Why Data Management is Today’s Most Important Business Discipline” for more on the growing importance of data management from a business perspective.
- AI / ML Model Development. An open, agile AI / ML model training, development, and management environment that uses a hub-and-spoke organizational structure to drive close collaboration between the lines of business and subject matter experts (critical for Feature Engineering) and the analytics hub that institutionalizes the development, sharing, and continuous refinement of the organization’s analytic assets and data products to drive quantifiable business and operational outcomes.
- MLOps. Provide a set of frameworks, tools, methods, and governance practices and policies to deploy, monitor, and ethically, responsibly, reliably, and efficiently maintain AI / ML models in production (that are instrumented to learn from the AI model False Positives and False Negatives to mitigate AI model confirmation bias).
- AI-optimized Infrastructure. An agile, extensible, on-demand infrastructure that can be easily provisioned and is optimized for Big Data, Edge, and AI-centric workloads from edge to core to multi-cloud.
To open the AI-driven Decision Factory to all stakeholders, organizations must educate everyone in the organization on the capabilities of analytics; that is, what is the realm of what is possible using basic and advanced analytics (Figure 5).
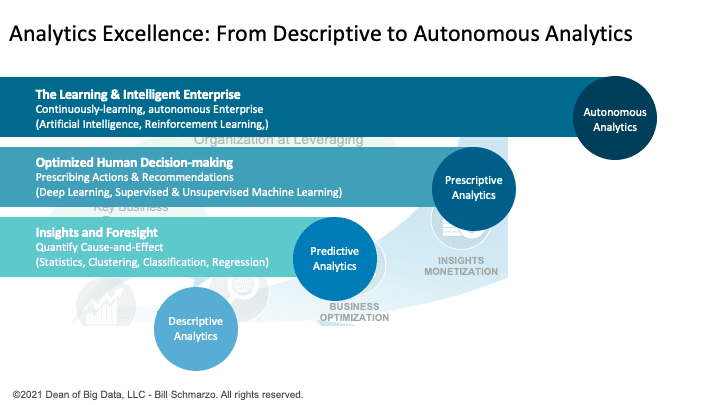
Figure 5: Analytics Maturity: from Descriptive to Autonomous Analytics
This Analytics Maturation Curve outlined in Figure 5 is comprised of the following stages:
- Stage 1: Descriptive Analytics leverage statistical and data mining tools and technique to analyze and profile data sets (mean, medium, mode, standard deviation, variance, min, max, n-tiles), explore data variables cause-and-effect, and quantify cause-and-effect confidence levels and measure goodness of fit.
- Stage 2: Predictive Analytics leverage exploratory analytic techniques (e.g., clustering, classification, regression) to uncover and codify customer, product, service, and operational trends, patterns, and relationships buried in the data that can be converted into predictive insights (predicted behavioral and performance propensities) to predict what is likely to happen.
- Stage 3: Prescriptive Analytics leverage the predictive customer, product, service, and operational propensities (Machine Learning Features) in building machine learning and deep learning models that deliver dynamic recommendations and next best actions.
- Stage 4: Autonomous Analytics leverages reinforcement learning and artificial intelligence techniques to create models that can continuously learn and adapt with minimal human intervention. Autonomous analytics seek to optimize around the AI Utility Function by taking action, learning from that action (i.e., maximize rewards while minimizing costs), and adjusting the next action based upon the learning with minimal human intervention.
As part of the analytics educational process, everyone in the organization should have a rudimentary understanding of the different ways that advanced analytic models “learn” including Machine Learning (Supervised Learning and Unsupervised Learning), Reinforcement Learning, Deep Learning, Transfer Learning, Federated Learning, Meta Learning, and Active Learning (Figure 6).
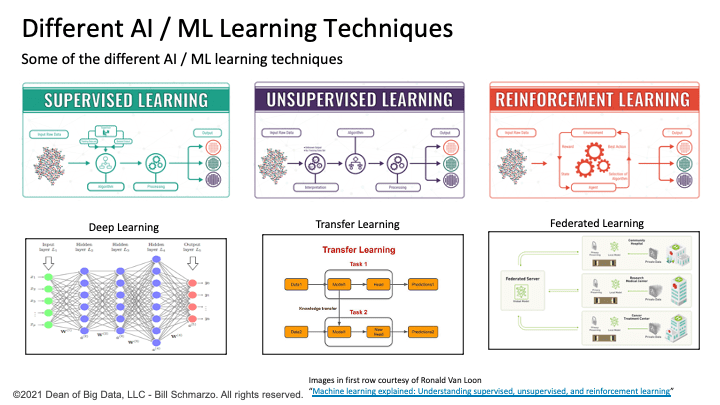
Figure 6: Different AI / ML Learning Techniques
Summary: Decisions Part 1: Creating an AI-driven Decision Factory
Creating a continuously learning and adapting AI-driven Decision Factory means empowering all employees (and partners and customers) to envision, ideate, try, fail, learn, share those learnings, and try again in applying data and analytics to derive and drive new sources of customer, product, service, and operational value. And that requires creating a culture of analytics-driven innovation.
We will cover that subject and more as we explore the value-realizing, collaborative power of decisions.