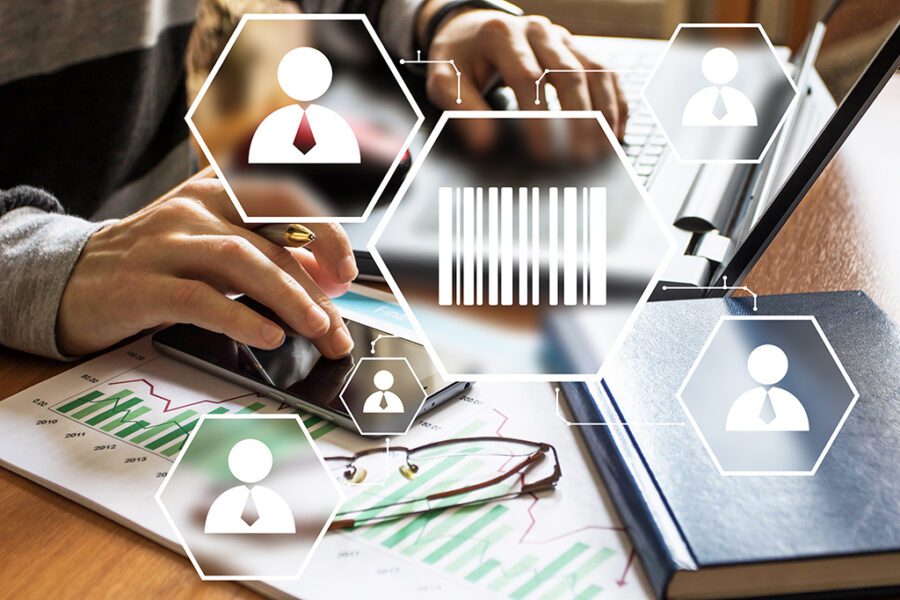
When I was the Vice President of Advertiser Analytics at Yahoo!, I painfully learned that my targeted user personas (Media Planners & Buyers and Campaign Managers) didn’t want more data in helping them optimize their marketing, campaign, and advertising spend across the Yahoo! Ad Network. Heck, they didn’t even want analytics!
The aspirations for these personas were to become VPs of Digital Marketing or Directors of Social Media Marketing or Digital Advertising Executives. The last thing they wanted was to become data analysts who had to churn through massive data sets to uncover the audience, messaging, and campaign insights needed to achieve their marketing, campaign, and advertising objectives. In fact, that’s what they wanted Yahoo! to do for them!
Given their campaign objectives and goals (views, clicks, shares, comments, conversions, etc.), they wanted Yahoo! to tell them the ideal target audiences, the best websites to engage those audiences, the best times of day to reach them, keywords to buy, and what messaging and content were most interesting to them (based on views, clicks, shares, comments, and conversions). What these personas wanted from Yahoo! were decisions – decisions that optimized across these objectives to deliver meaningful outcomes that helped them attain their desired marketing, campaign, and advertising objectives.
They wanted to decisions. What they needed were data products.
What is a Data Product?
Kevin Petri, Vice President of Research at Eckerson Group, recently posted a question on LinkedIn asking for feedback on the machine learning platform architecture in Figure 1.
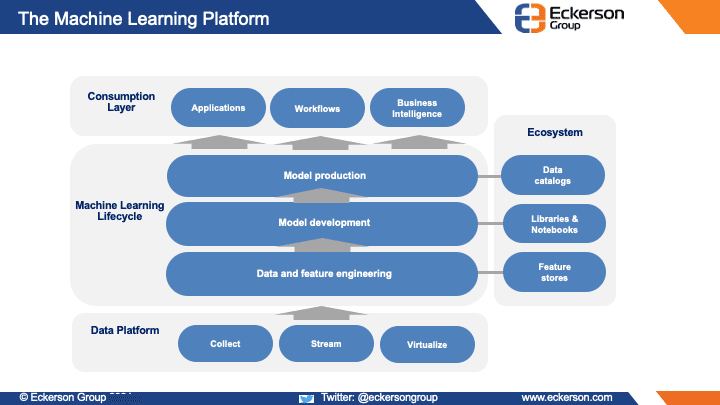
Figure 1: Machine Learning Platform as defined by the Eckerson Group
As Kevin defined it:
“The machine learning (ML) platform offers a flexible environment in which data science teams can manage machine learning in an efficient, governed, and scalable way. It helps data science teams standardize features, ML models, and software code; then reuse and share those artifacts as they integrate with business processes.”
The biggest opportunity for making the machine learning platform relevant to the business is clarifying how we package and monetize the machine learning platform (and the underlying, relevant data sets) by integrating the concept of Data Products (that drive specific, meaningful, relevant business outcomes) into the architecture (Figure 2).
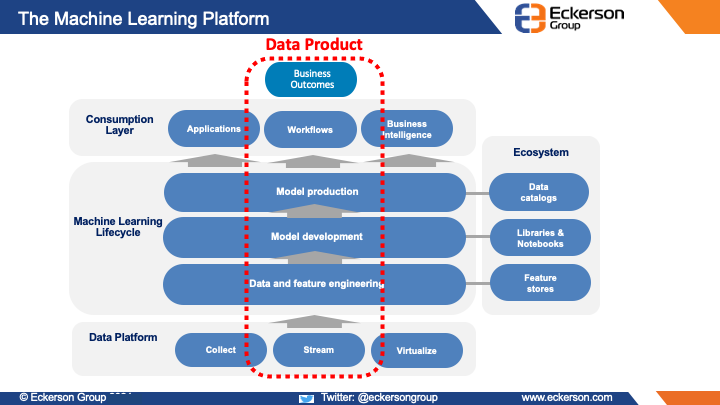
Figure 2: Machine Learning Platform + Data Products
Data Products are a category of domain-infused, AI/ML-powered apps designed to help non-technical users manage data-intensive operations to achieve specific, meaningful, relevant business outcomes.
Well, let’s review some key requirements in building a Data Products business model.
Data Products #1: Identifying Sources of Customer Value Creation
The defining characteristic of a Data Product is its ability to leverage customer, product, service, and operational insights to “intelligently simplify” the decisions that customers are trying to make. Delivering successful data products requires an intimate knowledge of the customers’ intent, what outcomes they are seeking, and how success will be measured.
The “Thinking Like a Data Scientist” methodology provides a framework and design templates (i.e., Stakeholder Persona) to help the Data Products development team to clearly understand and align around the stakeholder’s objectives and goals, desired outcomes, current pain points, key decisions, and KPIs against which to measure decision effectiveness (Figure 3).
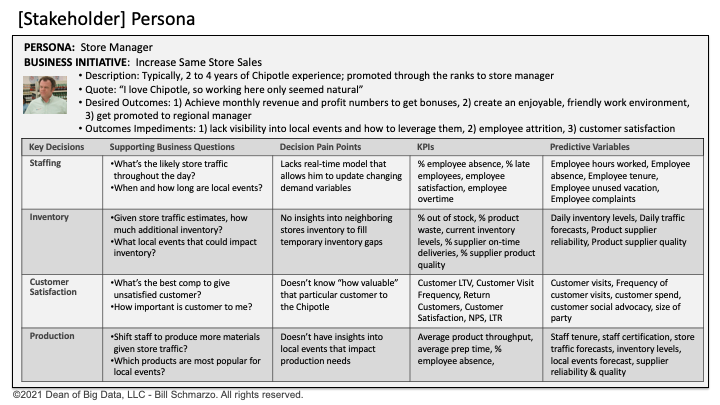
Figure 3: Stakeholder Persona Template
Another critical design template in defining the Data Product stakeholder usage requirements, benefits, and pains is the Stakeholder Journey Map (Figure 4).
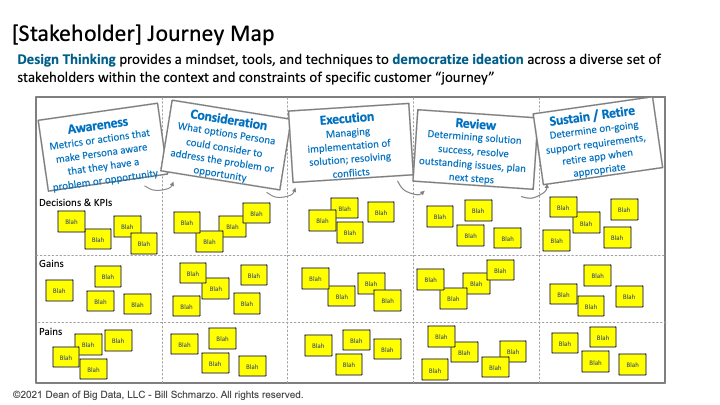
Figure 4: Stakeholder Journey Map
The Stakeholder Journey Map further refines the decisions, KPIs, Gains (sources of customer value), and the Pains (impediments to customer value) that the targeted stakeholder experiences along their journey to achieving the desired outcome. The Stakeholder Journey Map is a wonderful tool for walking in the shoes of your stakeholders in order to identify, validate, value, and prioritize the data and analytics (and ultimately the Data Products) that could help them successfully navigate their journey and achieve their desired outcomes and goals.
All of the “Thinking Like a Data Scientist” work culminates in the Hypothesis Development Canvas, another design template artifact that details what capabilities we are going to need to enable in our Data Product. The Hypothesis Development Canvas is a great alignment tool for ensuring that the business and operational requirements and desired outcomes of the targeted stakeholders are captured so that the data and analytics team can build a usable, relevant Data Product (Figure 5).
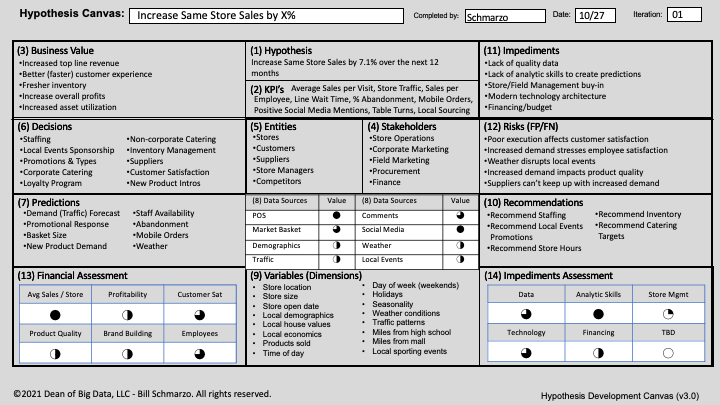
Figure 5: Hypothesis Development Canvas
Data Products #2: Exploiting the Economics of Data and Analytics
In the blog “Features Part 2: Clarifying the Data-Features-Use Case Value Topology”, I introduced the data and analytics architectural typology that links the critical data and analytic components including data, features, ML models, predictive scores, and Key Performance Indicators (KPIs) that deliver business outcomes via business use cases (Figure 6).
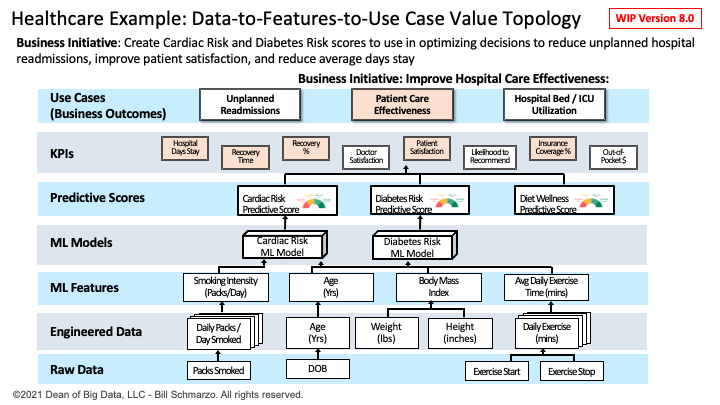
Figure 6: Healthcare Use Case Machine Learning Platform Topology
This solution stack in Figure 5 looks very similar to Kevin’s Machine Learning platform discussed in Figure 1 with a bit more detail and an example of how these data and analytic components interact to deliver a Healthcare business outcome.
And here’s the power of Data Products – Data Products can share and reuse the same data and analytics infrastructure and same data and analytic assets at near-zero marginal cost. That’s right. If architected correctly, Data Products can exploit the economics of data and analytics through data and analytics sharing, reuse, and continuously learning and adapting (see the business and operational effects of the Schmarzo Economic Digital Asset Valuation Theorem).
Data Products #3: Orchestrating Data Products to Re-invent Value Chain
That ability to share, reuse and continuously refine the underlying data and analytic components not only accelerates the development of Data Products but also enables the orchestration or linkage of Data Products across a common data and analytics architecture to address more complex business value chain use cases.
For example, we can orchestrate a series of data products to solve a bigger problem, such as improving the organization’s prospecting and customer retention effectiveness. That is, improving and accelerating the ability to identify, reach, acquire, retain, mature, and build a community around more high-value customers (Figure 7).
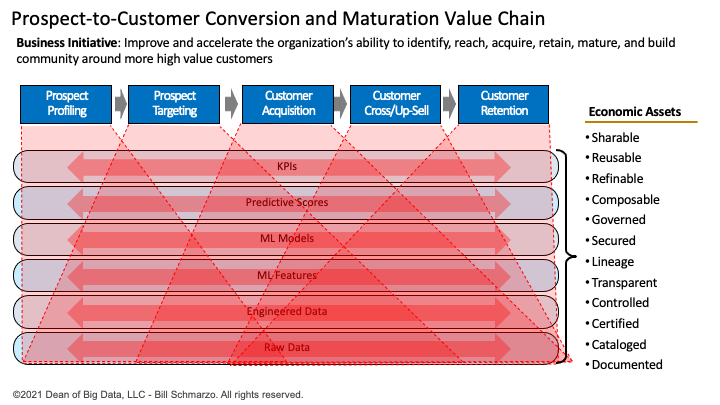
Figure 7: Example of Orchestrating Data Products to Solve More Complex Use Cases
The Prospect-to-Customer value chain in Figure 6 could integrate across a series of data products (Profiling Data Product, Segmentation Data Product, Targeting Data Product, Cross-sell Data Product, Advocacy Data Product, Retirement Data Product) where the customer, product, and promotional learnings from each data product are pushed back down into a contextual knowledge center (asset models, analytic profiles, feature stores) where those customers, product, and promotional learnings can be shared and re-used across the other Data Products.
Summary: Building a Data Products Business
Data Products represent the packaging of the Machine Learning platform to deliver well-defined business or operational outcomes, and continuously learn and adapt the effectiveness of those Data Products are used (something that AI / ML are great at facilitating).
The Machine Learning platform offers organizations the opportunity to extend their business model by transforming their data (or more specifically, the customer, product, service, and operational insights buried in the data) into customer-engaging, revenue-generating, market-transforming, continuous-learning, and adapting Data Products.