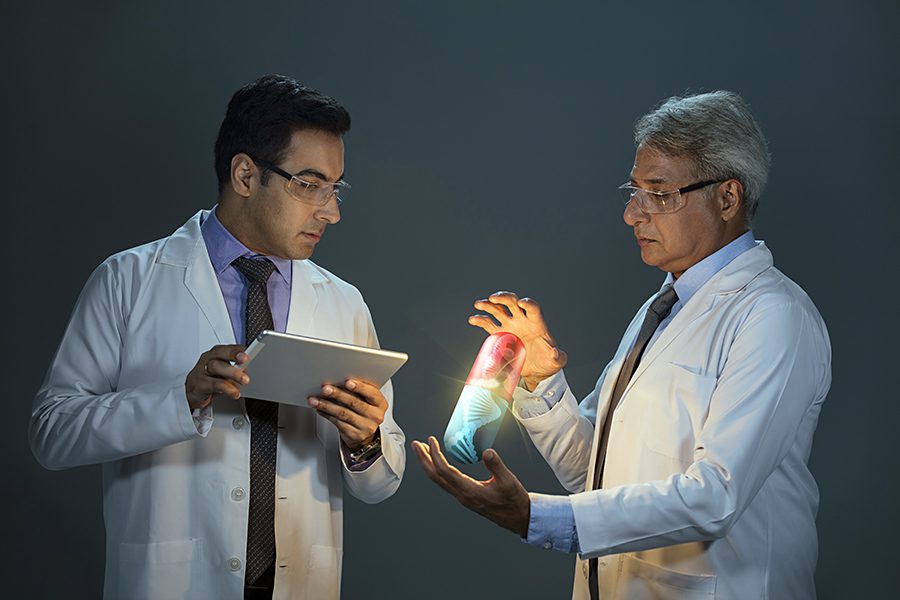
Data annotation is at the forefront of the recent revolution in healthcare AI, driving continuous progress in the field through continuous innovation through the idea of Artificial Intelligence. A computer program can use human intelligence to perform many tasks that humans carry out today. The concept is called artificial intelligence (AI).
Finding tumors, discovering kidney stones, or checking for tooth decay without manual intervention is possible with AI. However, AI engines need to be taught how to think and behave in order to be able to do that. In order for this to happen, humans must create accurate training datasets.
AI engines are built on the foundation of data labeling & annotation. As long as the foundation is strong, AI engines will be reliable. The terms ‘data’ and ‘annotation’ may be understood separately, but what is meant by ‘data annotation’ needs to be explicitly stated because of its position as a means through which artificial intelligence enables healthcare industry participants to accomplish more.
Healthcare Data Processing — Labeling & Annotating Raw Medical Datasets
Human intelligence cannot be used to make sense of what we could call raw data since it cannot be accessed by a machine, or even by software. Essentially, computers are designed to ingest information, apprehend patterns, and apply them to the next set of data they come across without human bias in order to reach the conclusions they have been taught to reach.
The raw data must be transformed into a format that a machine can ingest and use to calculate rules. A software program needs to be taught how to interpret MRI scans if they are the means of evaluating whether a tumor exists or not. Otherwise, they are just meaningless sets of pixels for the software program. This brings the need for healthcare data processing, i.e., the medical images are annotated with appropriate markings and labeled with the relevant tags to enable machine learning models to understand the patterns within the pixels.
Developing Automated Disease Diagnostic Abilities in Machines through AI
AI is capable of working similarly to a specialist doctor who knows how to review hundreds of X-ray and MRI scans every week to arrive at a conclusion about whether a patient has any symptoms of disease or not. Developing the same cognizance in machines is possible with the right medical training data which is appropriately labeled with the right tags and annotated with the right markings.
Feeding the medical training data into a machine learning model designed for automating medical processes requires high-quality data labeling and annotation. The training data fed into the machine enables the AI-powered medical application to perform the medical tasks it is designed for. There is a bunch of medical data labeling companies in the market, e.g., Cogito, Analytics, etc. that boast high-end expertise in patient records labeling and medical data annotation that you may be seeking for the purpose of training an AI algorithm.
Enabling AI in Healthcare through Data Labeling
Thanks to continuous innovations in data annotation, AI quality has improved greatly in recent years. Artificial intelligence is used to simplify the process in several aspects of healthcare, such as virtual assistants, diagnoses, medicine, and surgery.
The ability of artificial intelligence to perform better and improve its accuracy requires years of development training. Consequently, it is common to have artificial intelligence capable of prescribing, inferring, analyzing, and estimating data.
Because the quality of data annotation and medical document labeling gives make or break shot when it comes to implementing AI in healthcare. For this reason, the medical data, e.g., clinical reports and diagnostic scans need to be properly labeled and annotated before they can be applied in health care AI. Training and recruiting these professionals could be a great deal of time and expense.
It is even more evident that AI is having an increasingly strong influence. In many practical applications today, AI produces similar or faster results than human intervention based on statistical data. There are several of these technologies, such as AI-enabled surgery is sometimes more effective than human intervention in certain surgical procedures today. Researchers found the rate of complications during AI-assisted robotic surgeries was five times lower than when surgeons performed the procedure alone.
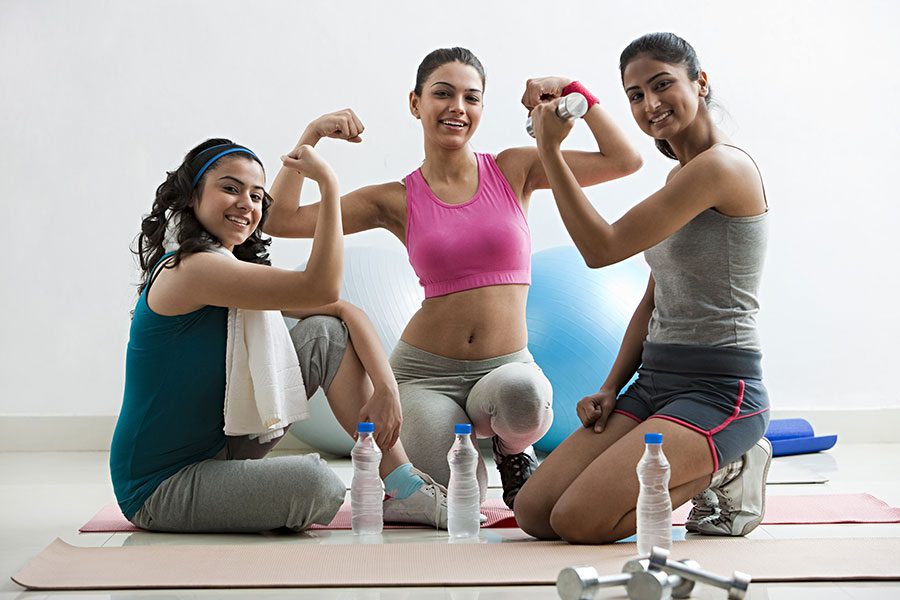
AI-assisted robotic surgery; Similar to how AI has enhanced driving safety, similar technology is being used to make surgery a safer and quicker process. Here are some use cases of AI implementation in healthcare that show how the machine learning technology has significantly improved the medical practice and procedures:
Virtual Nursing Care
Patients’ resources are wasted needlessly when they are taken to hospitals without necessity. By offering 24/7 answers to patients’ questions, AI is able to produce personalized responses by improving Sentiment/Intent Analysis and Entity Recognition. Aside from determining illnesses, scheduling appointments, monitoring health statuses, and reminding patients about medications, AI uses remote monitoring sensors.
Aid Clinical Diagnostic Decisions
Specialists can better diagnose diseases by analyzing medical images with appropriately enhanced AI algorithms through medical document labeling and image annotation. Diagnostic problems, such as CT scan analysis, are prone to human error. As a result of incorporating AI into the process, medical analysis has become quicker, more accurate, and lower in cost.
Drugs Manufacturing
Healthcare providers will be able to see chemical and biological interactions more efficiently with machine learning algorithms, making it possible to rapidly develop drugs for a variety of new diseases and conditions.
Why Prefer Outsourcing for Medical Data Labeling?
Despite the fact that healthcare data processing, i.e., medical labeling and data annotation is highly significant for incorporating AI in healthcare, it is believed to be the most tedious and unappreciated part of the medical machine learning process. It, in all the commonsense, stands to a reason why medical practitioners and medicine & healthcare equipment manufacturers should prefer outsourcing when integrating AI into the medical processes.
Here are the 3 key points on how outsourcing healthcare data processing can bring an edge to the AI implementation approach in your healthcare practice/process:
You have Industry-Specific Expertise to Harness
When you pay your money, you have the liberty to make the right choices for your business ventures. Tending to keep everything in-house related to healthcare data processing will stretch your budget beyond the limit. However, wearing an outsourced outfit for medical image annotation and labeling can get you sorted for everything at a lower cost, with industry-specific expertise from professionals in the data processing domain.
You have a Dedicated Team to Work on Your Data Labeling Tasks
While the whole cost of data labeling and annotation gets lower with an outsourced medical document labeling partner, you have the advantage of engaging a dedicated team to work on your medical data processing tasks. Companies like Anolytics, Cogito, and to name a few others, attain annotation expertise in a long span of time. Getting any one of such expert annotation companies to handle your medical data labeling things will get you sorted for the rest.
On-Point Accuracy and Quality up to the Par in Data Labeling
Maintaining quality and on-the-point accuracy in labeling and annotating data is too much hard work and knowledge. Moreover, access to modern annotation and labeling tools, which ensure accuracy and quality in labeling, is a prohibitively expensive transaction. An outsourced partner in hand not only boasts knowledge but also brings access to the modern annotation and labeling tools to provide you with on-point accuracy in the data labeling work.
Conclusion
As the new era begins, the ability to be incompetent is no longer acceptable. Health care providers can now better and faster serve their patients with the aid of artificial intelligence than ever before. Datasets and data annotation and other medical data processing things are the core building blocks for healthcare AI
, getting right with the datasets and medical data processing requires expert intervention.
Though the innovations around AI, machine learning, and the core annotation and labeling processes going on in the background brings a promise of a fulfilling future for automation in healthcare, conquering challenges around datasets and data labeling still demands the intervention of an outsourced data labeling and annotation expert for an accomplished AI incorporation in medical practice.