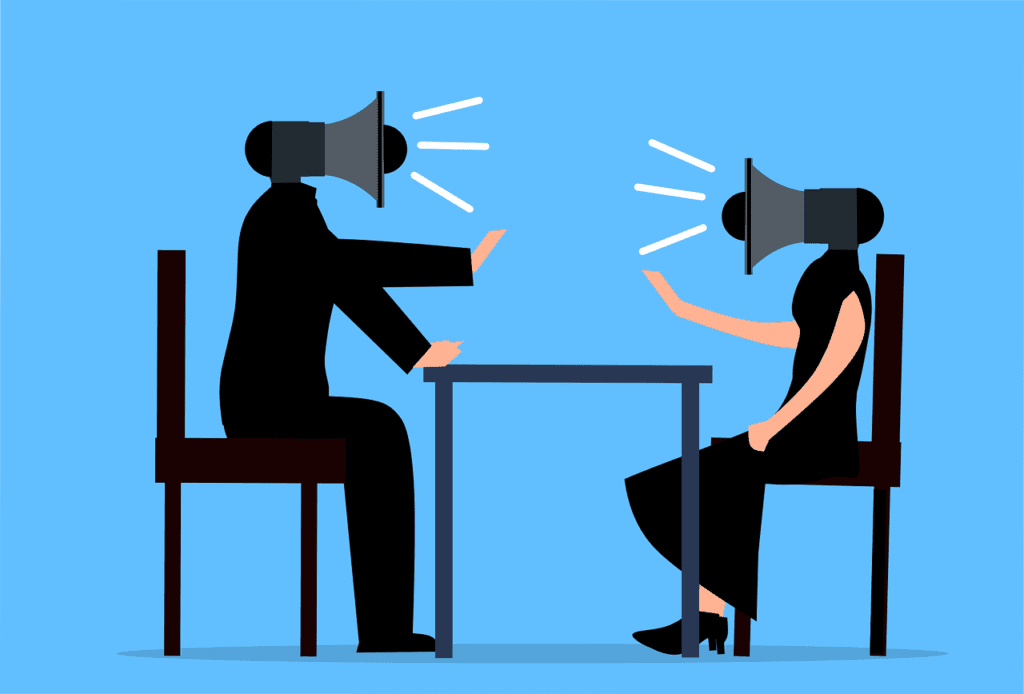
If I could name one reason why business will face at least one more AI winter, it’s the lack of nuance in most business AI discussions. The buzz about large language models (LLMs) has sucked much of the oxygen out of the air for complementary technologies. The truth is that LLMs are no more a panacea than “blockchain” was back in the 2010s.
Why is there a lack of nuance in AI discussions? One tribe–the numerical, probabilistic, neural net tribe behind today’s generative AI–dominates the discussion and wins the vast majority of investment. The reason they dominate is the utility of the prompt interface and its ability to emulate natural human language. Today’s GAI delivers plausible results, and at a minimum, gives coders a first draft to work with.
When it comes to AI, most investors are placing one big bet. The bet is that statistical machine learning alone is enough.
Today’s lopsided AI tribalism reflects what’s happening in politics too. Consider the bell curve of current political opinion. The moderate majority in the middle is even more silent than it was in the 1960s. Meanwhile, the extremes in the long tail are far more strident and vocal. They’re the ones getting the lion’s share of attention. As a result, they command a lot more sway per person than the moderate middle does.
Within the context of the extremes dominating the moderate middle, objective journalism has had to take a back seat. In a 31 July MediaBuzz podcast, veteran Fox News media reporter Howard Kurtz observed, “I’m a journalist. I’m not on either side. But people don’t want to accept that anymore.”
The business community does understand it needs general AI, not just narrow AI. And yet, General AI is complex. It is deep, underexplored and multi-faceted. The universe of the different kinds of logic AI must exploit is broad. There is no way one tribe by itself clears a path for general AI.
Instead, a hybrid approach, one that today is already blending the power of neural nets and the symbolic AI of knowledge graphs, is really the only approach that harnesses the potential of smart, connected data and moves us toward meaning through extensible, machine readable context.
The nature of understanding in effective communications
In July 2023, CEO Chris Brockmann of knowledge graph solutions provider Eccenca told a story in a LinkedIn post about a point of disagreement regarding knowledge-based versus numeric-only AI.
“The other party,” Chris said, “seemed to believe that numeric AI could deduce decisions from data through ‘learning.’ I was trying to explain that good decisions as well as true innovations first and foremost require an #understanding of the ‘why’, e.g., the #context as well as a lot of foundational knowledge.”
Chris shared a video from an evangelist named Courage Edoma that shed light on the topic of understanding. In the video, Edoma made these simple but insightful points, which I’m paraphrasing here:
- Seek understanding. Find out what you need to do with what you know.
- Understanding is knowing something or someone correctly.
- Understanding is the ability to interpret something correctly….
- Understanding makes a message profitable.
- You can’t profit from a message you don’t understand.
- You can’t benefit from information without understanding.
- Incorrect messages can be dangerous.
- Misunderstanding is behind many of society’s problems.
- Understanding leads to smooth relationships.
- Understanding gives us an upper hand in business.
Blending the deterministic and the probabilistic in today’s AI
Guesswork has its place, but facts and rules are foundational for shared business understanding. Data scientists focused on machine learning don’t always consider that deterministic, fact- and rule-based computation was the norm until the 1990s, when advances in compute, networking and storage finally allowed machine learning to flourish.
The emergence of effective, large-scale machine learning doesn’t imply that more mature, but still evolving methods of computation become obsolete. “Symbolic AI” is a term that’s often misunderstood because it has been narrowly defined merely in terms of 1980s-era decision support systems. Today’s knowledge graphs are also symbolic, and yet much broader in scope and richer in capabilities.
Effective AI governance hinges on a foundation of logically interrelated facts and accompanying rulesets that humans and machines together can enforce. Both the facts and the rules can live with the instance data in a knowledge graph.
Ronald Ross, Co-Founder of Business Rule Solutions and author of the book Rules: Shaping Behavior and Knowledge, points out that “Rules are how you create order from disorder. That’s what laws, statutes, regulations, contracts, agreements, etc. are all about. Rules are also how you remember things and avoid unconscious biases and discrimination (perhaps in machine learning as well).”
Many who are developing knowledge graph capabilities already understand how symbiotic knowledge graphs and large language models can be. Mike Tung, CEO of the automated knowledge graph platform Diffbot, notes that “By guiding the generation of language with the ‘rails’ of a Knowledge Graph, we can create hybrid systems that are provably accurate with trusted data provenance, while leveraging the LLM’s strong abilities in transforming text.”