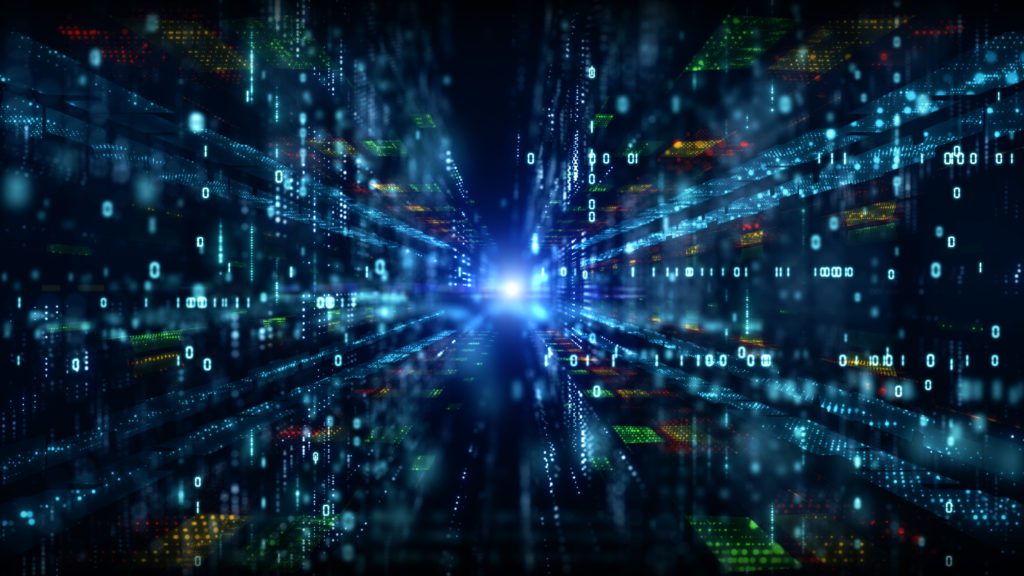
Defining Data Observabilityand Data Quality
As companies gather seemingly endless data streams from an increasing number of sources, they start to amass an ecosystem of data storage, would-be end-users, and pipelines. With each additional layer of complexity, opportunities for data downtime, moments when data is partial, erroneous, missing, or otherwise inaccurate, multiply. As a result, data teams spend most of their time on data quality issues instead of working on revenue-generating activities for the business.
Data observability can be defined as the holistic view that includes monitoring, tracking, and triaging incidents to prevent downtime of the systems. At the same time, Data quality is a measurement of how to fit a data set to cater to an organization’s specific needs.
Contrasts between Data Observability and Data Quality
Data observability is based on five pillars. These include;
- Freshness
Data pipelines can break for many different reasons, but one of the main culprits is a freshness issue. Is freshness the belief of “is my data updated? What is its recency? Are there loopholes in time when the data has not been updated and do I need to know about that?”
- Distribution
Distribution relates to your data assets’ field-level health. Null values are one way that helps us understand distribution at the field level. For example, if you expect a specific percent invalid rate for a particular field and then suddenly increases in a big way, you may have a distribution issue on your hands. Apart from null values, other measurements of a distribution change include unusual representations of expected values in a data asset.
- Volume
Volume refers to the number of data in a file or database, and it checks whether your data intake meets the expected capacity. In addition, volume refers to the completeness of your data tables and offers insights into the health of your data sources.
- Schema
Schema is a structure explained in formal language as supported by a database management system. Often, schema changes are the culprits of data downtime incidents. For example, fields are added or removed, changed, etc., and tables are removed or not loaded correctly. Having an audit of your schema is an excellent way to think about the state of your information as part of this Data Observability.
- Lineage
Lineage aids in telling a story about the state of your information; for example, upstream, there was a schema change that led to a table downstream that had a freshness issue that leads to in another table downstream that had a distribution error that resulted in an erroneous report the team is using to make a data-driven conclusion about their product.
Data quality, on the other hand, is based on six metrics.
- Completeness
You can think about completeness in two ways: at the attribute level or record level. This measures whether all the required data is present in a specific dataset. Measuring completeness at the record level is a bit more complex, as not all fields will be mandatory.
- Accuracy
How accurately does your information reflect the real-world object? Data accuracy is usually black or white in the financial sector. It either isn’t or is accurate. This is because the number of pounds and pennies in an account is precise.
- Consistency
Maintaining synchronicity between different databases is essential. However, to ensure data remains consistent daily, software systems are often the answer.
- Validity
Validity involves measuring how well data adapts to required value attributes. For example, ensuring dates adjust to the same format, i.e., date/month/year or month/date/year.
- Timeliness
Timeliness shows the accuracy of data at a specific point in time. An example of this is when a customer moves to a new house, how timely are they informing their bank of their new address? Few people do this immediately, so there will be a negative impact on the timeliness of their data. Poor punctuality can also lead to bad decision-making.
- Integrity
To ensure data integrity, it’s essential to maintain all the data quality metrics we’ve mentioned above as your data moves between different systems. Typically, data stored in multiple systems break data integrity.
Where do they overlap?
Data observability and data quality overlap when data observability is used to better data quality. When organizations adopt data observability to enhance data quality, there are bound to be great results. Some of them include;
- Cost savings by getting data anomalies before they impact consumers-When an anomaly occurs, the data observability engine alerts the team immediately, allowing time to investigate and troubleshoot the problem before it affects consumers. Since the Data Engineering team is notified of the issue before it involves stakeholders, they could fix their pipeline and avert future anomalies from jeopardizing the integrity of their data.
- Improved collaboration by tracing field-level lineage-data observability helps understand dependencies.
- Raising productivity by keeping tabs on deprecated data sets-data observability gives greater transparency into critical data assets’ relevancy and usage patterns, informing them when different attributes are deprecated.
- Increase cost savings by reducing time to resolve tiresome data fire drills and regain trust in critical decision-making data.
- Better organization between data engineering and data analyst teams to comprehend critical dependencies between data assets.
- Drive greater efficiency and productivity by adding end-to-end visibility into the health, usage patterns, and relevancy of data assets.
Conclusion
It can be concluded that data observability and data quality rely on organizations to function well. As much as there are differences, the two overlap in various ways that help better data quality and delivery. To learn more about data observability, schedule a demo.