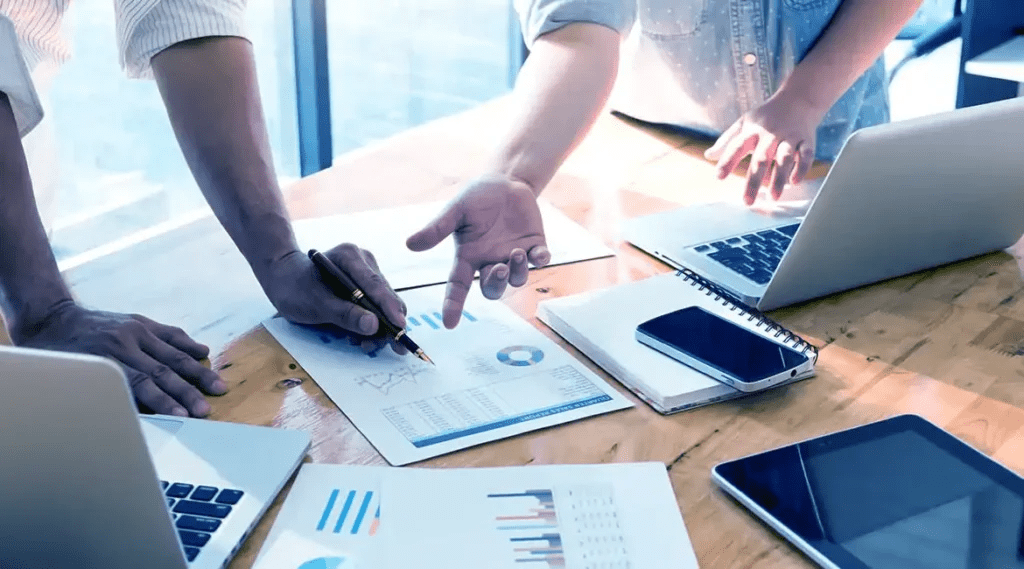
As companies gather seemingly endless data streams from an increasing number of sources, they start to amass an ecosystem of data storage, would-be end-users, and pipelines. With each additional layer of complexity, opportunities for data downtime, and moments when data is partial, erroneous, missing, or otherwise inaccurate, multiply. As a result, data teams spend most of their time on data quality issues instead of working on revenue-generating activities for the business.
Data observability can be defined as the holistic view that includes monitoring, tracking, and triaging incidents to prevent downtime of the systems. At the same time, Data quality is a measurement of how to fit a data set to cater to an organization’s specific needs.
Where do they overlap?
Data observability is used to better data quality. When organizations adopt data observability to enhance data quality, there are bound to be great results. Some of them include;
- Cost savings by getting data anomalies before they impact consumers-When an anomaly occurs, the data observability engine alerts the team immediately, allowing time to investigate and troubleshoot the problem before it affects consumers. Since the Data Engineering team is notified of the issue before it involves stakeholders, they could fix their pipeline and avert future anomalies from jeopardizing the integrity of their data.
- Improved collaboration by tracing field-level lineage-data observability helps understand dependencies.
- Raising productivity by keeping tabs on deprecated data sets-data observability gives greater transparency into critical data assets’ relevancy and usage patterns, informing them when different attributes are deprecated.
- Increase cost savings by reducing time to resolve tiresome data fire drills and regain trust in critical decision-making data.
- Better organization between data engineering and data analyst teams to comprehend critical dependencies between data assets.
- Drive greater efficiency and productivity by adding end-to-end visibility into the health, usage patterns, and relevancy of data assets.
It can be concluded that data observability and data quality rely on organizations to function well. As much as there are differences, the two overlap in various ways that help better data quality and delivery. To learn more about data observability, schedule a demo with us.