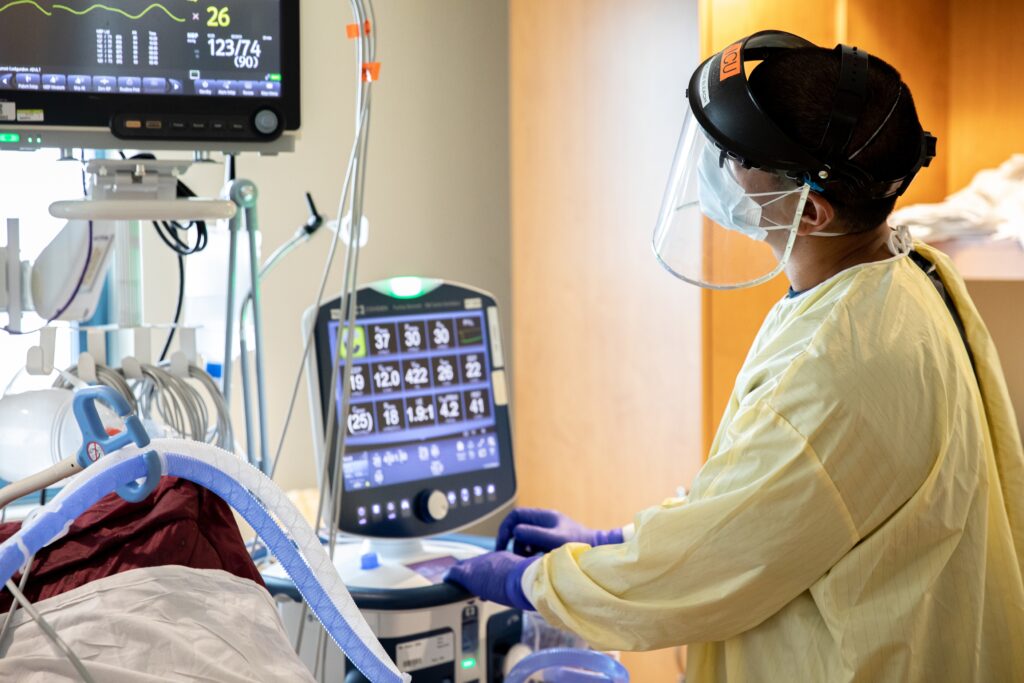
One of the more telling exhibits at April’s NTT Upgrade 2024 event in San Francisco was the Autonomous Closed-loop Intervention System (ACIS) in development at NTT Research’s Medical & Health Informatics Lab (MEI). The ACIS concept underscores how hospitals can embrace a systems-level automation initiative starting with the intensive care unit to create a patient-centric, personalized, continually updated data management environment that can radically boost patient outcomes.
The situation in a typical ICU
A major part of an intensive care unit’s work in a hospital involves stabilizing patients in serious or critical condition. Stabilization is the process of ensuring that vital signs such as rate of breathing, blood oxygen levels, pulse, blood pressure and temperature stop trending in the wrong direction and instead return to normal levels as soon as possible.
ICUs can struggle or fail to stabilize a patient for a wide range of reasons. From a data perspective, doctors, nurses and the support systems they depend on need the right information at the right time and place to optimize the level of care.
As you might imagine, ICUs tend to lack quite a bit of information about new patients just arriving in the ICU. To determine the best treatments requires interoperability at the data layer between sources. Some information is patient-specific, while other information is cohort-specific or more general in terms of a given condition the patient suffers from.
Detailed information about treatments is equally important in this scenario. The MEI Lab at NTT Research uses a digital twins metaphor to describe how feedback loops and interoperable data models can continually improve patient, condition and treatment data.
The more hospital staffs and their systems lack insights about a patient and the specifics of how they respond to specific treatment, the more they’re forced to guess when making treatment decisions. The more guesswork, trial and error to meet the need, the longer it takes to stabilize a patient.
Automated, personalized medicine in the era of digital twins
“If we know enough about a patient, if we have a digital representation of that patient, then we can predict how individual drugs will affect that patient,” says Jon Peterson, distinguished research scientist at the MEI Lab. “Knowing those predictions, we can put together a system that will allow us to very rapidly deliver the appropriate drugs to a patient.”
The digital twin approach reflects an understanding of the challenges ICU staffs have when it comes to responding quickly and appropriately with the right amounts of the right drugs, given circumstances that change quickly and patients that have individual needs. ACIS is designed to harness three kinds of evolving, digital twin-oriented data sources:
- Cardiovascular information from the patient
- Cardiovascular information from the patient population at large
- A drug library
The system then monitors the patient’s response to the treatment and adjusts dosages accordingly. This feedback loop updates the digital twin of the patient. You could imagine that hospitals could also contribute anonymized information back to the drug pipeline providers in order to update drug library information.
What ACIS implies in terms of organization-wide data requirements
I’ve written before in these pages about the best practice of knowledge graph-based data management when creating and evolving digital twins. (See the IOTICS Portsmouth Ports use case at https://www.datasciencecentral.com/preconditions-for-decoupled-and-decentralized-data-centric-systems/, for example.) Portsmouth Ports now monitors and shares timely metrics on emissions updates to a broad range of industry users. Armed with this information, shipping companies, for example, can determine which vessels, when and where, are out of compliance with emissions control requirements. This level of temporal + spatial detail is essential to bring various transportation types and the ports themselves into compliance with UK regulations.)
Also relevant is the Montefiore Health Patient-Centered Analytics Learning Machine (PALM) in the Bronx,. (See https://www.datasciencecentral.com/beyond-data-science-a-knowledge-foundation-for-the-ai-ready-enterprise/ for more information.) PALM brings together many critical, but disparate external and internal sources so that machine learning and advanced analytics methods can be run that benefit from the entire connected, continually updated whole. The knowledge graph-based PALM as a result can predict and prevent specific occurrences of sepsis and respiratory failure, for instance.
Knowledge graphs, digital twins and other top trending AI technologies in 2024
In closing, here are three facts worth noting when it comes to trending technologies that need to be harnessed together in next-generation systems like ACIS:
- Gartner in April 2024 released its Impact Radar. In the bullseye of its Impact Radar target are two technologies: knowledge graphs and generative AI. These two technologies can and should be designed to be symbiotic.
- Without knowledge graphs, GAI will continue to hallucinate, an issue semantic graph database provider Stardog explores at length in its 19-question May 2024 FAQ “19 Questions about LLM Hallucinations.” In the discussion of Number 18, Stardog points out: “…databases matter because structured data and data records matter. In fact, one under-appreciated fact of retrieval augmented generation’s (RAG’s) inadequacy is that it doesn’t work very well with structured data….”
- Gartner in another April 2024 article, “When Not to Use Generative AI,” emphasizes the need for a system-level perspective of the kind that ACIS encourages for organizations to use AI appropriately. “Generative AI is only one piece of the much broader AI landscape, and most business problems require a combination of different AI techniques. Ignore this fact, and you risk overestimating the impacts of GenAI and implementing the technology for use cases where it will not deliver the intended results.”