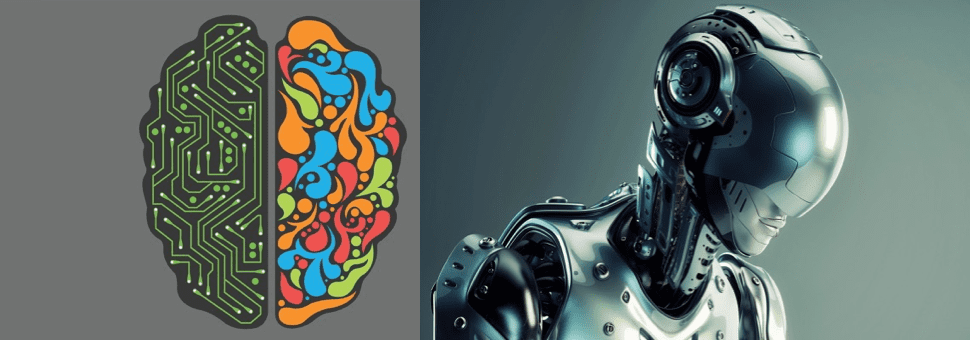
The immediate and pressing need for ‘digitizing’ your supply-chain
- According to a 2020 CGE report, companies which commit to digitizing their supply chains can expect to save up to 50 percent on supply-chain costs; besides, a 20% reduction in procurement costs, apart from an increase in revenue of over 10% — resulting from incremental competitive advantage. ( Source: Center for Global Enterprise (CGE) in partnership with CREATe.org)
- World-class supply chain organizations can save up to 45 % in procurement costs through digital transformation. (Source: Hackett Group)
One may conclude: ‘Digitizing’ the supply-chain has become a survival necessity for companies to stay competitive. Apart from a substantial jump in the efficiency-effectiveness, the customer-experience, and upside to revenues, companies can expect a huge-huge cost-saving…
A Look at the Future: Components of Data-driven (Digital) Supply-chain
Note
Any Data-driven Supply-chain, or AI-driven Supply-chain will only be as good as the ‘quality, granularity, and richness’ of the underlying “data” supporting the decisions.So the most critical component of any “Digital Supply-chain” is the data, and the underlying Information Supply-chain supporting the Physical goods Supply-chain.
A good strategy consultant, among other things, is expected to begin by describing the end goal… So, before we attempt to create a roadmap for building a data-driven supply chain, let us first try to envision what it might look like.
Defining the “ideal” end-state: Featuring an AI-driven Digital Supply-chain of future
The supply chains of the future will be powered by self-managing, automated technologies that can collect and analyze data in real time. Few examples below:
- #Cloud-based SCM tools & AI-driven algorithms for supply-chain planning and optimization
- #Granular geo-tagged data from extended value-chain covering supplier’s suppliers; to customers’ customers
- #Location-data (Location-stamp on every transaction)
- #Robotics in factories and warehouses: Robotic Automatic Storage and Retrieval systems
- #Self-driving trucks with GPS
- #IOT and Radio-tags embedded into into every SKU & every device in the Supply chain
- #Internet Based AI driven Bots (Listening Posts) for continuously scanning the market (internet) for better sources, better materials & better pricing & for quickly gathering & processing risk data (Geopolitical / supply-related)
- #AI-driven automated processes wherever possible
- #Data-driven decision-making across the value-chain.
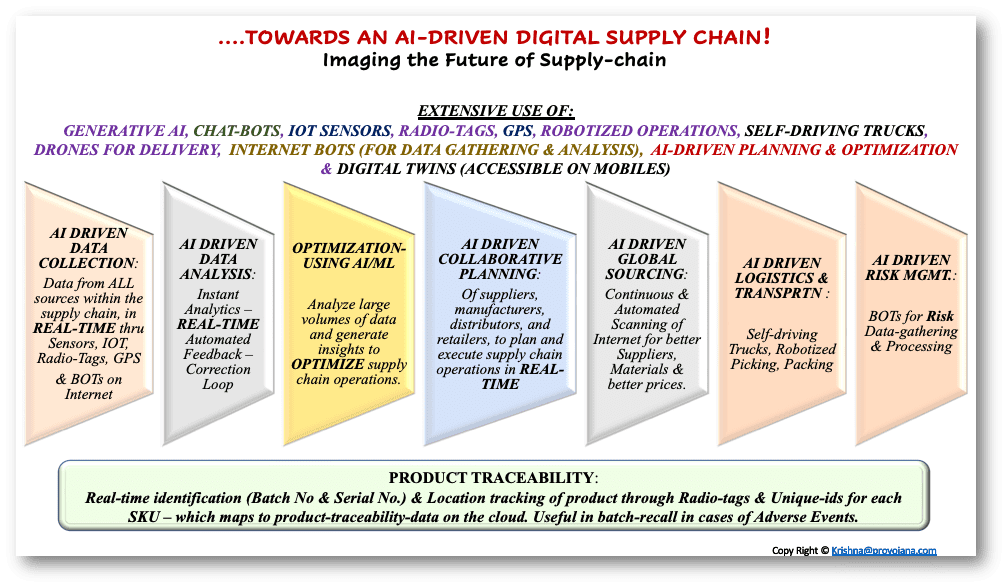
1. Data Collection: This is the most important part of the ‘Digital Supply-chain’. The future supply chains are expected to extensively use IOT, Radio tags, GPS, etc. for Automated Data Acquisition (event-driven, or period-driven in rare cases).
- # Enterprise data enhanced by seamlessly integrated geo-tagged micro-market data (perhaps made available on subscription mode by a third party)
- # All transactional data without exception will be geo-tagged & instantly distributed to cloud-based Apps & Data lake-houses.
- # Every Truck would have a GPS its location will be tracked 24×7. Every “Distribution Package” will carry a Radio-tag and its movement will be tracked through supply-chain.
- # Every purchase, every order fulfillment, every job progress, every project will be tracked with time & location-stamped data.
- # Every product & Every Job would carry a Radio-tag, and its movement through the workflow-stages would be automatically tracked.
- # Every Store & Warehouse would have completely automated, AI-driven — Automated Storage and Retrieval Systems (ASRS), that automatically load and unload the unitized-palletized loads, while keeping track of the locations (Bin Number) for every Part-No & every SKU.
- # All equipment & devices without exception would be IOT/GPS enabled and would automatically generate and distribute data into multiple analytics engines for control & continuous improvement.
- # All workers may carry id-tags with a GPS, or their mobiles will be tracked through a custom-corporate app.
- # All intra-office communication on mobiles through short-messaging apps & e-mails will be time-stamped and location-stamped.
- # Real-time Batch Tracking & Product Traceability data through Radio-tags & Unique-ids for each SKU
- # All communications with customers and vendors will be time and location-stamped.
- # The internal Enterprise-data will be complimented/supplemented with external-data, from sources such as Census data, Market data, etc., — all sorted, normalized, and geo-tagged, before uploading into Data Lake-house.
2. Transportation:
- # Every Truck would be Self-driven, GPS enabled, and will have the facilities for sending emergency distress signals.
- # Digital Twin (SCADA equivalent system to control Transportation network) to carry drill-down information of every package, every SKU carried by every Truck, down to the level of ‘serial number’ of every SKU.
- # AI-controlled Self-driving Drones for delivering products — especially for the last-mile delivery.
3. Warehousing & Supply-chain Operations: Completely Robotized — Automated AI-driven –
- # Robotized picking and packing, AI-driven automated storage and retrieval system (ASRS)
- # Automated Instant-Inventory taking at the press of a button using radiotags on every box, every pallet.
4. Customer / Vendor Collaboration
- # Generative AI-based Chatbots to communicate
- # Generative AI-based IVRS & Robotic calls for voice communication
- # Generative AI-based Social Media Campaigns
5. Risk Management :
- # Risk data to be collected using AI-driven Bot Listeners for picking up “Risk Signals”, “Mavericks”, or any “unusual activity”, or “unusual communications” — both from internal-enterprise-data & from the other external-data-sources including Public & Private databases, Social Media, and the Internet.
- # AI-driven Risk Reporting, Early-Warning-Signals
- # AI-driven automated ‘course-correction’ and ‘communications’ with vendors, customers, distributors, and employees.
- # The Lifed-items (SKUs with an expiry-date) to be picked and packed based on shorted expiry Batches first.
6. Product Traceability, Batch Tracking & Adverse-event Reporting
- # Real-time identification (Batch No & Serial No.) & Location tracking of product through Radio-tags & Unique-ids for each SKU — which maps to product-traceability-data on the cloud
- # Traceability data includes: the origin of materials and parts, their processing history, and their distribution, as stated in ISO 9000:2005 (2005) & Additionally, data connected to the past, use, or location of a product.
- # Easy & Instantaneous Batch-Recall in case of any AER– Adverse Event Reported (as in the case of Food or Pharmaceuticals, or even consumer-durables like automobiles ) with reference to a particular batch-number, would automatically trigger an alert along with a “Do not Sell / Do not Use Warning” — not just for the particular batch-number, but also for all the batches which used materials & parts from the same input-batches from same suppliers. The alert would automatically replicate itself through the entire supply-chain (distribution network) no matter where the particular ‘risky’ batches are located.
7. Digital Twins:
- # To be Mobile based: To be operated from “Anywhere & Anytime” for Planning-monitoring-correction & control
- # Digital Twins to map 100% of the extended-supply-chain, all stages, including the controllable Vendor’s Supply-chain & Customer’s Supply-chain.
- # Control Tower & AI to Co-Pilot decision making: AI-driven control towerto help managers make decisions & manage the overall performance of the supply-chain. Managers handle extreme exceptions alone.
8. Global Sourcing & Continuous improvement
- # Continuous & Automated Scanning of the Internet for better Suppliers, Materials & better prices — using Bots
- # Use of Generative AI for Price-Negotiations with customers.
- # AI-driven BOTs (Listening-posts) on the Internet, and Social-Media for picking-up signals on Threats (such as Adverse Events and geo-political risks), or Opportunities (better materials, better prices, better sources
Building a AI-driven Digital Supply-chain
I have extensively written on data-driven decision-making, data-to-decision lifecycle, and creating a roadmap and a business case for building a data-driven organization earlier. ( Please refer to my book Big Data for Big Decisions: Building a Data-driven Organization, P. Taylor & Francis, UK, 2022 & Articles on Data Science Central).
As I have argued: fancy advanced analytics platforms, and AI apart, the key to building a Data-driven organization is to focus on the key decisions — the 10% of the organizational decisions that account for 90% of business outcomes.
So for building a data-driven supply-chain, the following key questions must be asked and answered:
1. What are the key “supply-chain decisions” that account for over 80–90% of Supply-chain efficiency?
2. What is the data that supports the identified key decisions?
Note: The bigger and more primal issue most organizations face is: that they simply do not have the data that supports (the) key decisions within the organization…not with the requisite granularity or the quality. Needless to say, building a data-driven supply chain in the absence of the data is a futile and vain exercise, which nevertheless many CDOs are attempting to.
To quickly summarize, I advocate the following steps for building a data-driven supply-chain
Building A Data-Driven Organization | Building A Digital Supply-Chain | ||
Start from decisions. List all Organizational decisions, along with metrics like “Value-at-stake”, and the “frequency” | Create a Master-list of Process-Constraints & List decisions associated with each process constraint.Each process constraint at each workflow-stage represents a set-of decisions? (There can be many process-constraints, but only a few significant ones)The same / similar process-constraints may be recurring at multiple workflow-stages.. Note the Frequency of recurrence. Working out “value-at-stake” for each decision: Can we estimate the ‘loss of throughput’ due to bottlenecks, at each stage? | ||
Identify the 10% of the decisions that account for 90% of business outcomes | List the bottlenecks – from the biggest to smallest? And the 10% of the bottlenecks that account for 90% of throughput loss? Create a List of process constraints associated with the 10% of ‘critical’ bottlenecks. Note: All workflow stages are important. The absence of a current bottleneck does not diminish the possibility of a future bottleneck. | ||
Create a Roadmap & Business case | Create a roadmap for digitizing the supply chain by prioritizing the process constraints associated with the 10% of bottlenecks responsible for 80-90% of throughput loss. Note: Digitizing the key process constraints at all workflow stages is important… The criticality of a bottleneck (& associated process constraints) dictates its priority. | ||
Data behind the Decisions Create & Map the Information Supply-chain & the following as needed: Services Supply-chain & Cashflow Supply-chain | Map the flow of information (Data + Insights)’ required at each workflow-stage Map the flow of information (Data + insights) required for ‘easing-out’ the process-constraints and the possible bottlenecks. Map the Information supply-chain in-its entirety – (from data creation, to distribution, consumption, archiving, retrieval and repurposing) Cross-map the Information Supply-chain with Physical-goods Supply-chain – (clearly indicating the cause & effect relationship between the ‘information availability’ and its ‘impact on the process-constraint’.) | ||
Data that you need vs. data you have | Check: If you have all the data that you need to support the Supply-chain decisions For the Delta-Data: Create & implement a Data sourcing strategy | ||
Building Analytics to improve the quality of decisions | Build Analytics & AI solutions to improve the quality of Supply-chain decisions | ||
Build a Digital Twin: A remote replica of that provides the status & the control mechanism for the entire supply-chain (similar to SCADA systems). Typically you can change the process parameters using ‘digital twin’. |