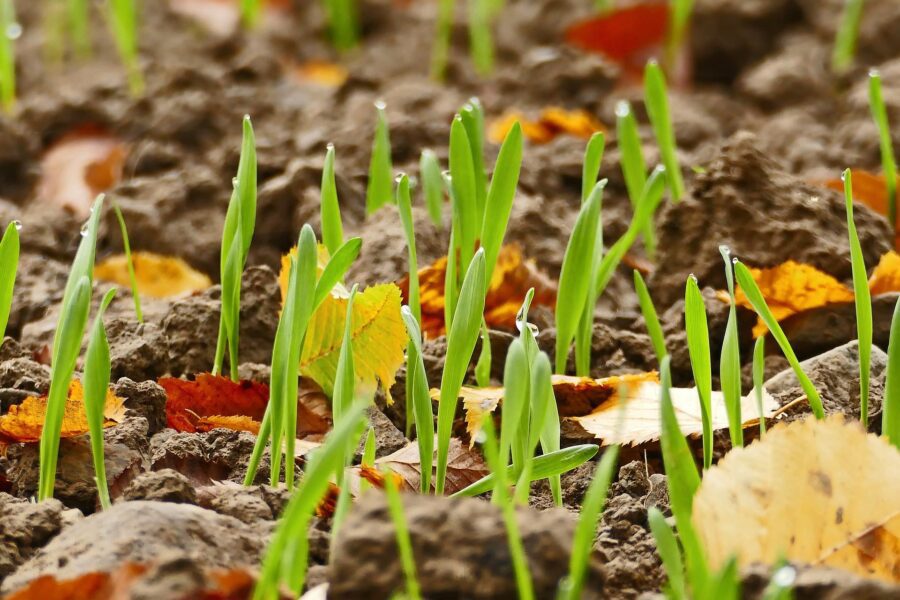
One of the things I do as a next gen systems-aware trends observer is look across the vast landscape of tech’s numerous tribal nations, compare and contrast, and try to draw conclusions about trends that might cross these boundaries, even in a small way. Much of data’s future depends on these tribes learning from and aligning with each other, rather than merely competing.”Coopetition” from the book of the same name is the term Brandenburger and Nalebuff came up with in the 1990s to describe what I’m talking about.
Even among those with the same job description who are using the same terms, there are many different tribes, each of whom uses the term “native” in a different way. “Native app” developers, for instance, seem to be different from “native web” developers. Even those who advocate hybrid apps want to call their apps “native.”
One thing you apparently don’t want your app to be is non-native, or at least you don’t want it to be perceived that way.
Although the goal of a given app is often to collect, analyze and share the results of data, many developers seem only focused on the immediate data need, rather than longer term reusability. An app, whether mobile or desktop, has been a place to trap data so that it serves only a single purpose.
Single-purpose data is doomed to be siloed, stranded and duplicative.
Organic Data-Centric Architecture
Don’t get me wrong–apps can be quite good at collecting data. It’s just what happens to data after it’s collected that can be disturbing. Data duplication is rampant. Even inside individual companies in the US, a given Social Security Number to uniquely identify a user can end up in thousands of different places.
The main cause for this tendency toward duplication and a throwaway data culture has to do with ancient architecture. A few companies are using well-designed data-centric architecture, but the rest have stuck with the old design, because it’s what they know, and because their perception is that the new design is untested and its stated advantages uncertain.
Another factor is that app design sells, but systems and data architecture design are much harder to sell to customers, because the payoff can be over the horizon, difficult to quantify and the outcome less certain. Most execs don’t want to talk about the plumbing.
The result is that every day, we create more data debt, due to application-heavy systems that generate lots of duplication, not to mention expanding risk footprints.
What’s the alternative to creating more and more inorganic data debt? An organic data farming approach, a commons focused on seeding, nurturing and harvesting data that’s inherently reusable and higher quality to begin with.
Departmental Tribalism
Inside enterprises, technologists become parts of another set of tribes. These are the tribes that reflect different departments,. Each department has its own turf, its own websites and SaaS subscriptions, and thus its own code sprawl and data siloing.
The departments that manage data are tribally different from one another, for reasons of history and focus. Some data is called “content”, while other data is called “knowledge”, and still other data is just called “data”, even though it’s just a subset of what’s being collected that could be harnessed if well integrated into the mix. Many large enterprises have a content management department, a knowledge management department, and a data management department–three different departments who often don’t often talk to one another.
It’s less necessary today to manage these different kinds of data differently, if you take advantage of the knowledge management techniques of knowledge graphs, This set of methods work for structured as well as less-structured data.
More and more, less structured data (”content” and “knowledge”) management opens the door to more scalable management of structured data too. The data management department can find innovations they can use that are long-established in the knowledge and content management departments–who are inherently more web-centric and thus able to take advantage of the scalability of webs or “graphs”.
Creating a Cross-Departmental Data Farming Commons
Treating different data differently is expensive, but how do you get three departments to work together as one, sharing the same architecture and methodology, in order to reduce the budget so that innovation becomes possible? Here are a few tried-and-true strategies that have worked for others:
- Start by addressing a single pain point, a project that a humble amount of effort and resources can sustain, to solve a problem all three departments can identify with..
- Make sure you’ve got strong, enduring support from activist leadership.
- Seek out those who’ve been using an approach to address a similar pain point successfully and learn from them.
- Bring together a project team with the mindset, skills, and passion to be able to execute, involving others who can help navigate the organizational thicket.
Ideally, passionate, cross-functional teams who empathize with those in multiple departments who feel the pain of a common problem can make several stakeholders happy at once with a data farming commons philosophy.