Interview with Dr. Andrea Isoni – SHOW 16
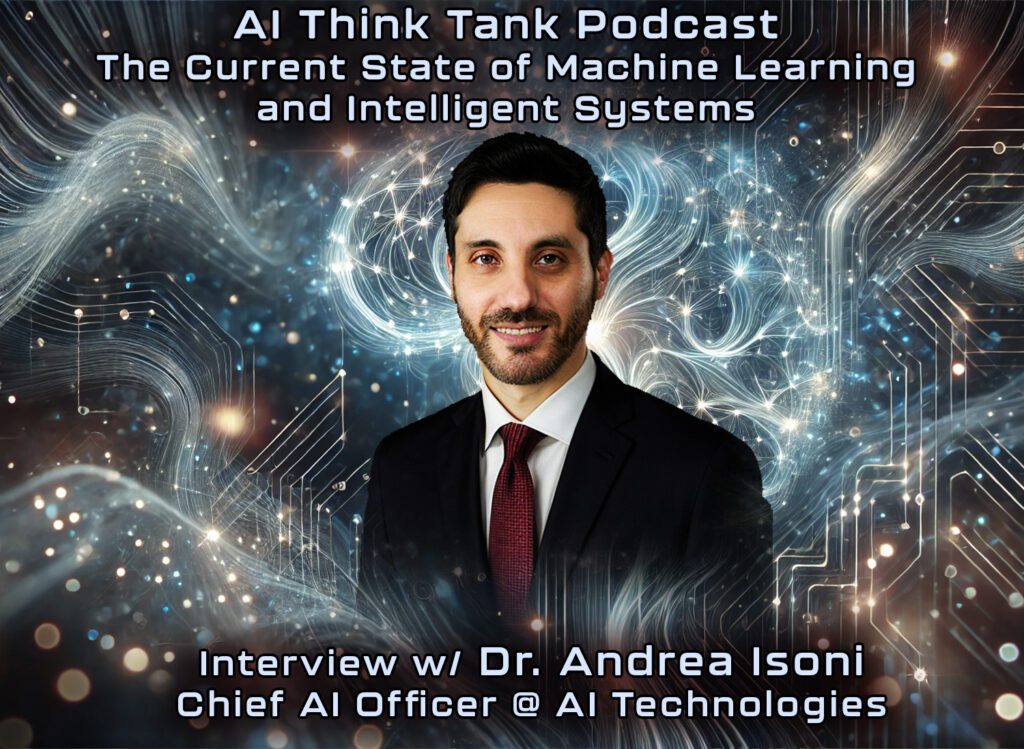
Intelligent systems are evolving faster than ever, and keeping up with the latest advancements requires expertise, foresight, and a deep understanding of both the technological and regulatory landscapes. In AI Think Tank Podcast – Show 16, I had the pleasure of sitting down with Dr. Andrea Isoni, Director and Chief AI Officer at AI Technologies, a consultancy specializing in machine learning and AI-driven solutions. Dr. Isoni is not only a thought leader in AI but also a contributor to international AI standards, including the newly established ISO 42001 for machine learning adoption.
Throughout our conversation, we explored critical topics shaping the AI industry, from regulatory compliance and model specialization to the challenges of explainability and the rise of agentic AI systems. This discussion offered a rare glimpse into the inner workings of AI deployment across industries, the complexities of standardization, and the future trajectory of AI development.
AI standards: The backbone of responsible AI deployment
As AI systems become increasingly embedded in critical industries—finance, healthcare, cybersecurity, and beyond—standardization is a necessity. Dr. Isoni, who has been directly involved in voting on the wording of international AI standards, explained why ISO 42001 is a significant milestone.
“Whenever you implement an AI solution, it’s not just because the AI Act is announced, at least in the UK. But it’s also because whenever you use an AI solution, you need to standardize the output. Hallucination is less of a problem today, but you still need procedures for when things go wrong—because everything can go wrong at least once.”
ISO 42001 provides a framework for organizations adopting machine learning, ensuring they minimize risks associated with AI failures. However, as Dr. Isoni clarified, compliance with ISO standards does not replace legal requirements—it simply helps organizations demonstrate that they are taking all reasonable steps to ensure AI reliability.
One of the most striking parallels we drew in our discussion was between AI standards and the ISO 9660 standard for CDs and DVDs. Just as ISO 9660 ensured that a music CD could be played on any player regardless of manufacturer, AI standards help ensure that machine learning models function predictably and ethically across different applications.
The AI specialization boom: Large models, smaller applications
Another major trend in AI is the shift toward specialized models. While large language models (LLMs) dominate the AI landscape, their efficiency can be improved by distilling them into smaller, more task-specific versions.
“Even when we consider smaller models, we need to understand one thing: Where do small models come from? Usually from the large models. You first need a super large model, and only after can you create a smaller version of that.”
Dr. Isoni explained that fine-tuned AI models, such as those optimized for coding (DeepSeek Coder, Code Llama) or language translation (ASA), are examples of this trend. Rather than creating new models from scratch, organizations increasingly take powerful foundation models and refine them for niche applications.
Additionally, post-training methodologies such as reinforcement learning from human feedback (RLHF) allow AI systems to refine their performance in specialized domains. As Dr. Isoni predicted, 2025 will likely see an explosion of specialized AI models built from large-scale pre-trained models.
Explainability: The persistent challenge of AI transparency
One of the most fundamental questions in AI research is: How do we explain what a model is doing? As AI systems become more complex, their decision-making processes remain opaque—a phenomenon often called the “black box” problem.
“I don’t think we will ever truly understand each activation in a large model. Even if we knew which neurons fired, understanding why would be extremely difficult. The best we can do is approximate the reasoning behind a model’s decisions.”
We discussed various approaches to increasing transparency, such as chain-of-thought (CoT) reasoning. Some advanced AI models, including DeepSeek, generate step-by-step explanations of their reasoning process, allowing users to understand why the AI arrived at a particular conclusion. However, true interpretability remains elusive, and standardizing AI explainability across different models is nearly impossible.
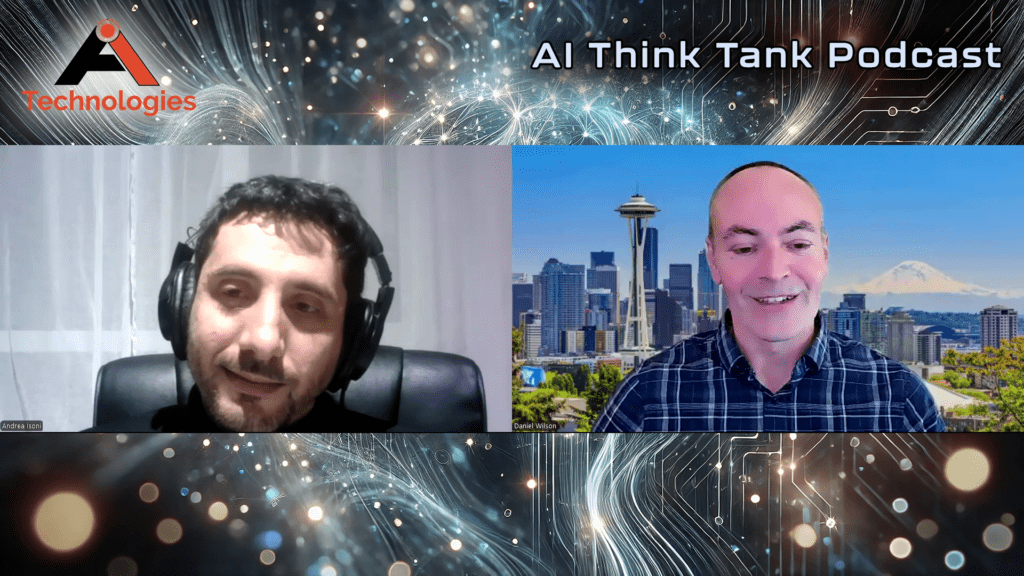
Agentic AI: The next evolution or a distant dream?
Agentic AI, where AI models autonomously break down tasks into subtasks and execute them, is one of the most hyped areas in AI development. But how close are we to true autonomous agents? According to Dr. Isoni, the answer is: not as close as some may think.
“The news makes it sound like the agentic era is coming now. But the reality is different. It’s too complex for industries today. The infrastructure isn’t there yet.”
For companies, deploying AI agents is fraught with legal, security, and technical challenges. While research labs and AI startups are experimenting with agentic AI for specific tasks—such as research automation and market monitoring—widespread adoption remains years away.
One of the most fascinating developments in this space is the intersection of AI and blockchain. Some companies are experimenting with decentralized AI systems that pool computing resources and data contributions from multiple users to refine specialized AI models.
“We may see a new battle, similar to Wikipedia versus Encarta. Will proprietary AI models dominate, or will decentralized, community-driven AI models take the lead? It’s unclear, but we’re at the early stages of that battle right now.”
The infrastructure gap: AI’s hidden bottleneck
AI compute power remains a bottleneck in model training and deployment. When it comes to data centers, the disparity between the U.S. and the rest of the world is staggering.
“There are over 3,000 data centers in the U.S. The second-highest country? Germany—with fewer than 300.”
This discrepancy means that companies outside the U.S. face significant hurdles in accessing the computational power necessary to train and deploy cutting-edge AI models. Cloud computing helps alleviate some of these challenges, but as Dr. Isoni pointed out, data localization laws complicate matters further—especially for industries like finance, where regulations prohibit storing certain types of data outside national borders.
Final thoughts: The future of AI implementation
Our conversation made one thing clear: AI is undergoing a profound transformation. As new models emerge and AI standardization efforts gain momentum, businesses must navigate an increasingly complex landscape.
Companies working with AI need to balance innovation with responsibility, ensuring that AI systems are secure, explainable, and compliant with evolving regulations. Specialization will continue to define the AI market, with more organizations fine-tuning large models for specific applications rather than developing models from scratch.
At the same time, infrastructure challenges and regulatory constraints will shape AI deployment strategies. Companies operating in highly regulated industries, such as finance and healthcare, must carefully consider whether to use cloud-based AI solutions, hybrid models, or on-premises infrastructure to meet compliance requirements.
As Dr. Isoni aptly summarized:
“AI is progressing fast, but large-scale deployment requires more than just powerful models. It requires standards, infrastructure, and a deep understanding of the risks involved. We’re moving forward, but there’s still a long way to go.”
With this in mind, the future of AI is not just about breakthroughs in model performance—it’s about creating a robust ecosystem where AI can be deployed safely, efficiently, and ethically. And that, perhaps, is the greatest challenge of all.
Join us as we continue to explore the cutting-edge of AI and data science with leading experts in the field. Subscribe to the AI Think Tank Podcast on YouTube. Would you like to join the show as a live attendee and interact with guests? Contact Us