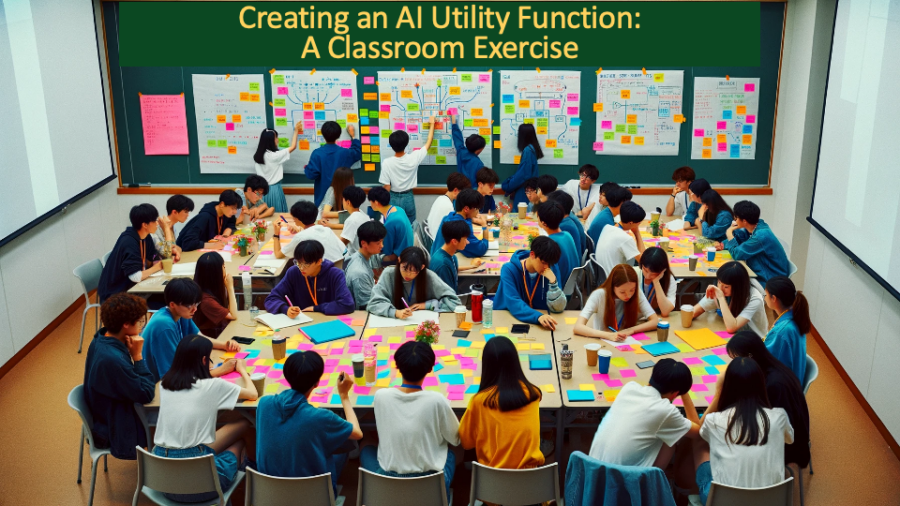
During a recent discussion with high school juniors and seniors, my goal was to enhance their awareness and understanding of Big (intrusive) Data and AI by focusing on two main areas.
- Firstly, I wanted students to understand how AI works so they did not fear it as a tool. AI will be an important tool no matter what profession they choose.
- Secondly, I wanted to make the students aware of their roles, responsibilities, and rights in ensuring the meaningful, relevant, responsible, and ethical design, development, deployment, and management of AI.
The bottom line is that I wanted to turn them into citizens of data science. And to accomplish that, I created a simple exercise where they got to play the role of the AI Utility Function. Let’s dive in.
What is AI? A Quick Review
Artificial Intelligence is a set of algorithms that seek to optimize actions by mapping probabilistic outcomes to utility value within a constantly changing environment…with minimal human intervention.
The students were intrigued by AI and eager to learn about its workings. They recognized that mastering AI is essential for a future where it will impact their personal and professional lives. During our discussion, I emphasized several critical concepts related to AI, including:
- Start with User Intent and Desired Outcomes: We start by understanding the user’s intent and desired outcomes—the ‘why’ behind the AI system. Training an AI model requires a holistic assessment of the ‘why’ before we start the training process.
- Human-Centric Control: Humans are the architects of the AI Utility Function and the associated variables, metrics, and desired outcomes that the AI model uses to learn and make decisions.
- Limited Autonomy: AI models will only do what they are trained to do. They do not have free will (at least not yet). The AI model’s actions will be guided by the user’s intent, desired outcomes, and the KPIs/metrics that measure outcomes’ effectiveness.
- Continuous Learning and Adaptation: An essential aspect of AI behavior is its ability to learn from feedback and adapt over time. This process involves analyzing the outcomes of the AI’s actions and adjusting the weights of the variables and metrics to enhance accuracy and effectiveness. Continuous learning enables the AI model to become more aligned with its objectives and responsive to changes, ensuring it remains effective in dynamic environments.
An AI Utility Function consists of variables, metrics, and associated weights that guide the AI model’s decisions, map probabilistic outcomes to utility values, and measure decision effectiveness to continuously learn and adapt (Figure 1).
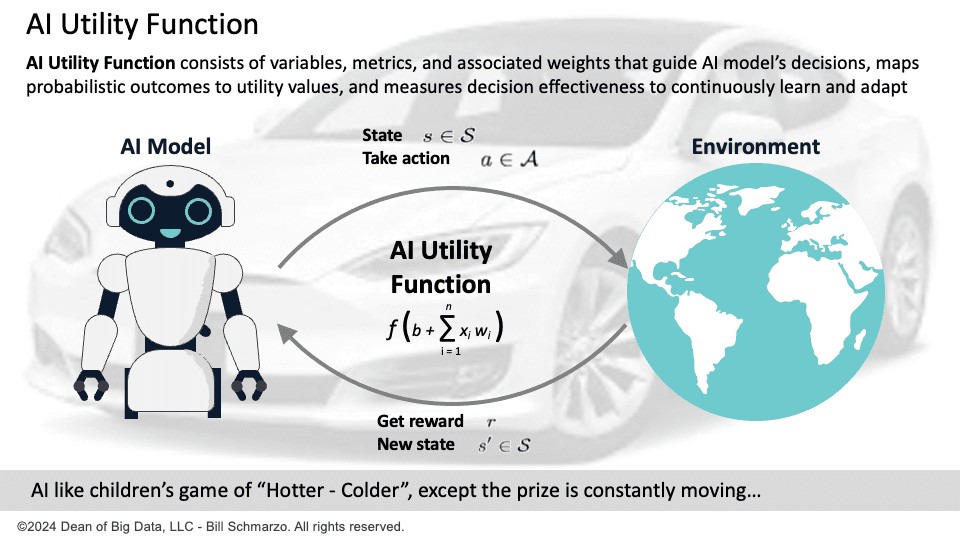
Figure 1: AI Utility Function
The AI Utility Function acts as the AI decision-making guide, assigning scores to possible actions based on their effectiveness in reaching a desired outcome. It assesses outcomes and directs the AI to take the action with the highest score, ensuring the AI’s decisions align with its objectives. For instance, it would rate movie suggestions to align with your tastes, continuously updating its choices with new data to consistently aim for the best outcome.
We must balance different and sometimes opposing factors to create AI models that make well-rounded decisions. For example, a business-focused AI should weigh immediate profits against the need to keep customers happy over the long term. By considering a range of variables and metrics, the AI model can judge outcomes more thoroughly, like ensuring product recommendations consider customer preferences, inventory, and supplier stability, not just potential sales.
To define a healthy AI utility function, one must think more broadly about the dimensions of variables and metrics against which to measure decision and outcomes effectiveness, including:
- Financial Value Creation: ROI, Return on Capital, NPV, Shareholder Value, Available Capital, Debt-Equity
- Operational Value Creation: Operational reliability, predictability, repeatability, stability, safety, sustainability
- Customer Value Creation: Customer satisfaction, fair value, LTR, NPS, quality, safety, social sentiment
- Employee Value Creation: Productivity, satisfaction, LTR, leadership, mentoring, influencer
- Partner/Ecosystem Value Creation: Financial health, reliability, quality, importance, supply risks, stability, ESG
- Societal Value Creation: Quality of life, clean air and water, diversity, job, housing, healthcare, and education equality
- Environment Value Creation: Energy efficiency, recycling, sustainability, reducing carbon footprint
- Ethical Value Creation: Doing good, giving back, mentoring, equity, harmony, devotion, ethics
It’s also vital for the AI Utility Function to focus on leading indicators that predict future trends rather than lagging indicators that reflect on past results. Emphasizing forward-looking indicators equips the AI to take actions that shape future success instead of only responding to what has already happened.
AI Utility Function Exercise
Scenario: You are trying to decide where to eat tonight.
Step 1: As a team, brainstorm the factors one “might” want to consider in deciding where to eat tonight. Capture as many ideas as quickly as possible; don’t try to judge their “worthiness” as you generate them. Your result might look like Figure 2.
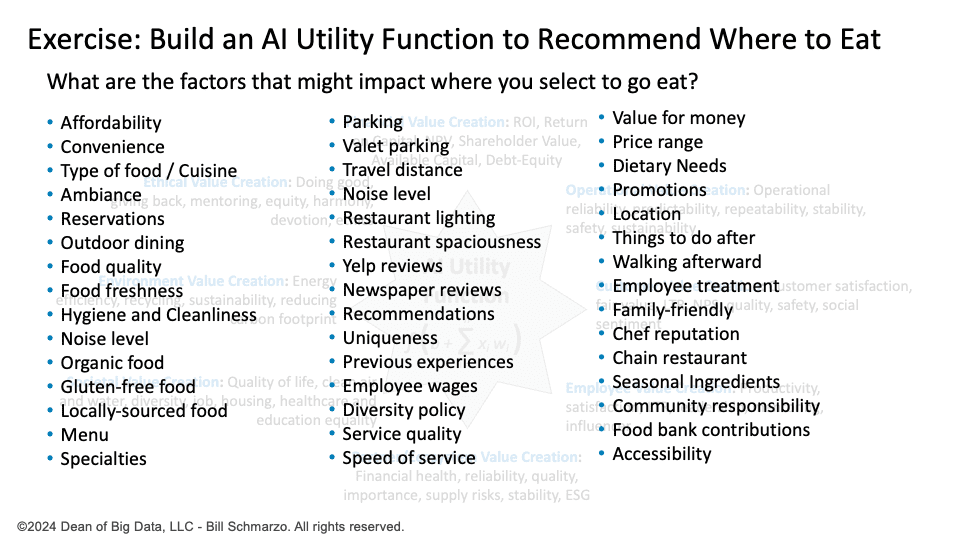
Figure 2: Factors that “might” influence where you go eat tonight
Step 2: Let’s play the role of the AI Utility Function by 1) understanding the user’s intent and 2) prioritizing or weighing the variables and metrics that might be most relevant given our intent. Let’s say our dinner intent is to grab something “quick and convenient.” Given this intent, the variables and metrics we might want to weigh more heavily are highlighted in red in Figure 3.
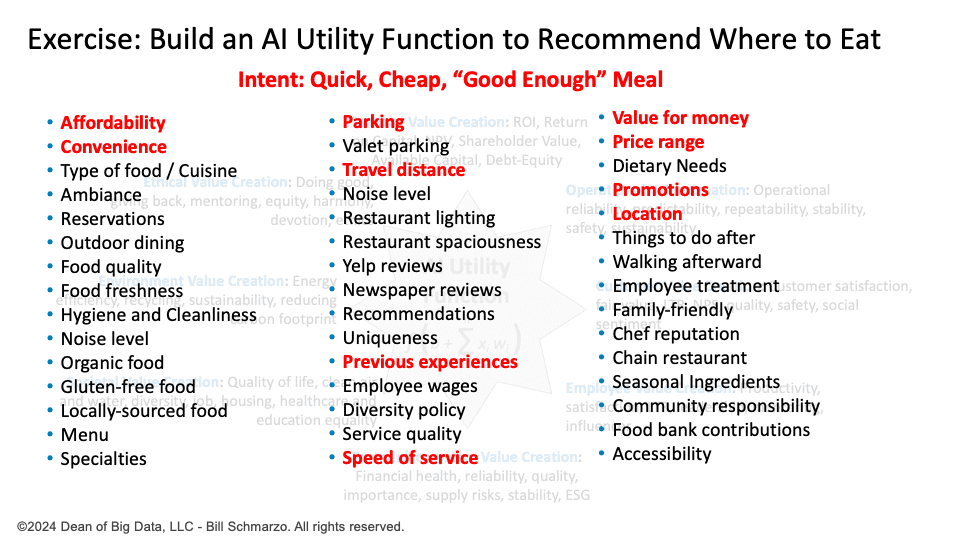
Figure 3: Intent: Quick, Cheap, and “Good Enough”
Step 3: Let’s change our intent to focus on finding a dining experience that meets our significant other’s date night expectations. To achieve this fine dining goal, we must modify the AI Utility Function to give more weight to the variables and metrics highlighted in red in Figure 4.
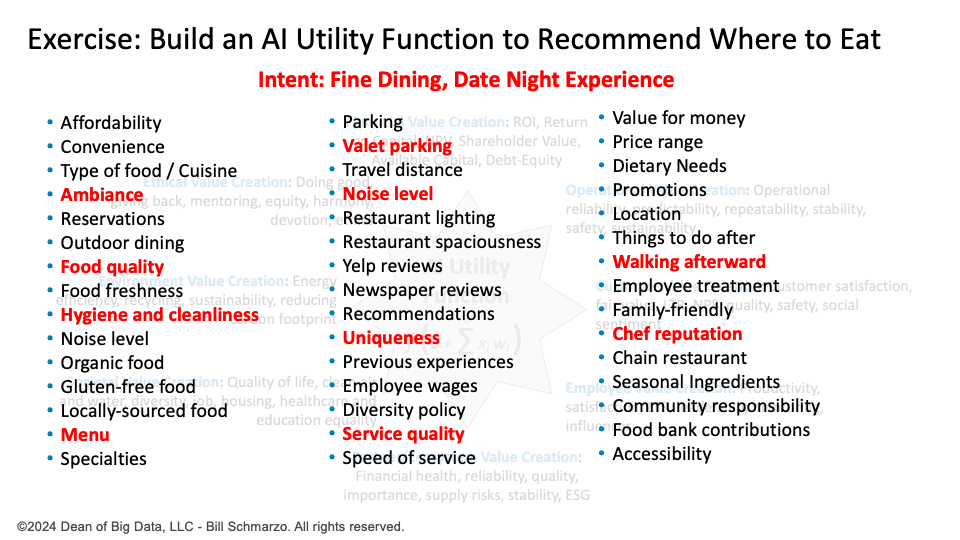
Figure 4: Intent: Fine Dining, Data Night Experience
You should notice that many of these variables and metrics conflict with each other—chef reputation versus affordability, speed of service versus quality of service, noise level versus family-friendly. As humans, we are always forced to make these trade-off decisions, even on simple decisions as to when to cross the street or what cereal to grab on Saturday morning (Cap’n Crunch is my preference).
We must force our AI models to make these same trade-off decisions. We must build decision conflict into the AI Utility Function and force it to make the required trade-off decisions as it seeks to deliver more relevant recommendations given our intent.
Creating an AI Utility Function Summary
Hopefully, this exercise helped the students understand that AI is not just a tool of convenience or a precursor of a dystopian future; it’s a sophisticated tool shaped by human hands and minds to help guide our decisions based on our intentions and desired outcomes. The real power lies not within AI itself but within the individuals who craft its purpose and guide its development—us. We have the collective responsibility to steer AI towards outcomes that are more relevant, meaningful, responsible, and aligned with our ethical compass. In doing so, we empower the next generation to master AI and contribute to its evolution as a force for good.
Finally, although I was the one teaching, I found the students’ insights, questions, and concerns enlightening. This was clearly a valuable learning experience for me!